Towards a Realistic Decentralized Naive Bayes with Differential Privacy.
Zenodo (CERN European Organization for Nuclear Research)(2022)
Abstract
This is an extended version of our work in [16]. In this paper, we introduce two novel algorithms to collaboratively train Naive Bayes models across multiple private data sources: Federated Naive Bayes and Gossip Naive Bayes. Instead of directly providing access to their data, the data owners compute local updates that are then aggregated to build a global model. In order to also prevent indirect privacy leaks from the updates or from the final model, our algorithms protect the exchanged information with differential privacy. We experimentally evaluate our proposed approaches, examining different scenarios and focusing on potential real-world issues, such as different data owner offering different amounts of data or requesting different levels of privacy. Our results show that both Federated and Gossip Naive Bayes achieve similar accuracy to a “vanilla” Naive Bayes while maintaining reasonable privacy guarantees, while being extremely robust to heterogeneous data owners.
MoreTranslated text
Key words
realistic decentralized naive bayes,privacy
AI Read Science
Must-Reading Tree
Example
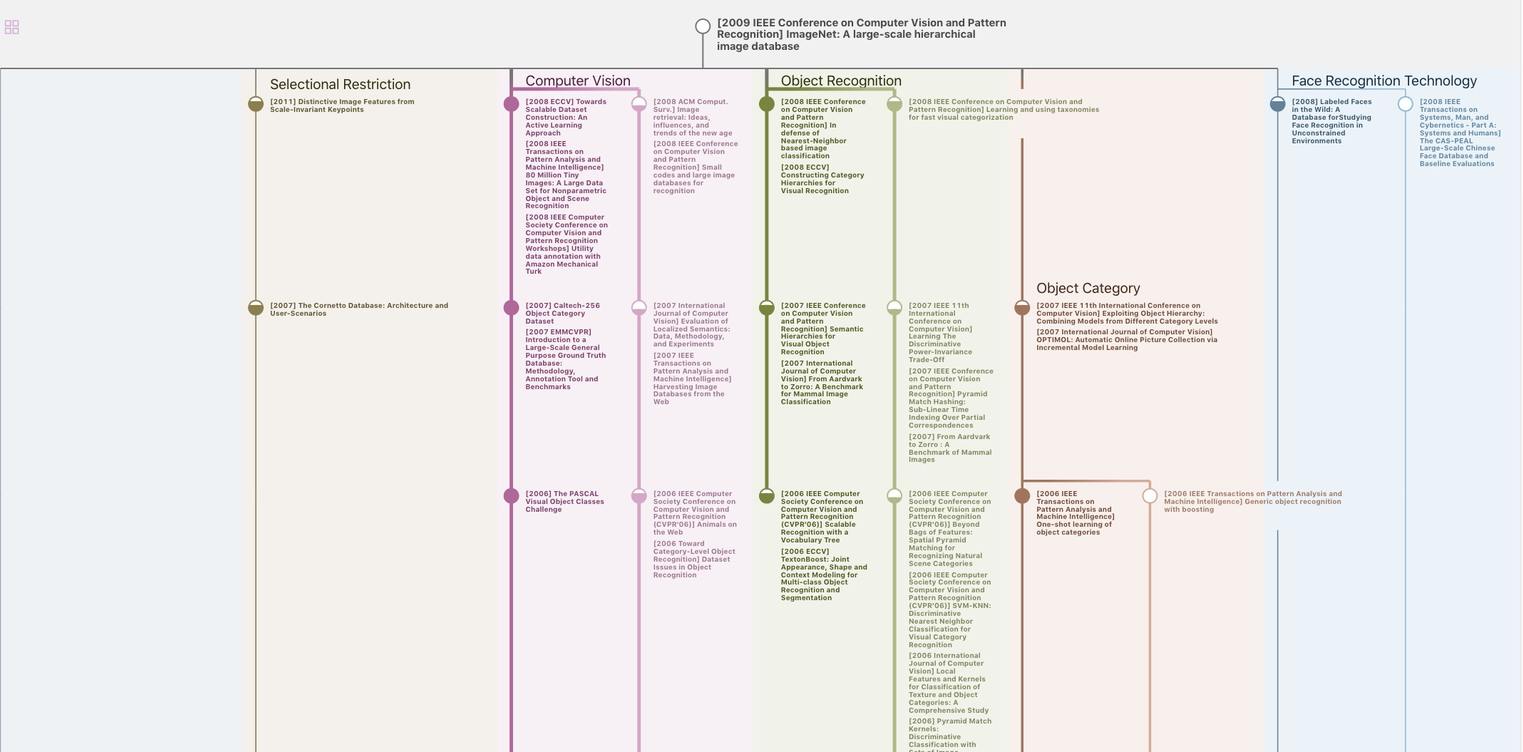
Generate MRT to find the research sequence of this paper
Chat Paper
Summary is being generated by the instructions you defined