Ensemble Deep Learning Object Detection Fusion for Cell Tracking, Mitosis, and Lineage
IEEE open journal of engineering in medicine and biology(2023)
摘要
Cell tracking and motility analysis are essential for understanding multicellular processes, automated quantification in biomedical experiments, and medical diagnosis and treatment. However, manual tracking is labor-intensive, tedious, and prone to selection bias and errors. Building upon our previous work, we propose a new deep learning-based method, EDNet, for cell detection, tracking, and motility analysis that is more robust to shape across different cell lines, and models cell lineage and proliferation. EDNet uses an ensemble approach for 2D cell detection that is deep-architecture-agnostic and achieves state-of-the-art performance surpassing single-model YOLO and FasterRCNN convolutional neural networks. EDNet detections are used in our M2Track multiobject tracking algorithm for tracking cells, detecting cell mitosis (cell division) events, and cell lineage graphs. Our methods produce state-of-the-art performance on the Cell Tracking and Mitosis (CTMCv1) dataset with a Multiple Object Tracking Accuracy (MOTA) score of 50.6% and tracking lineage graph edit (TRA) score of 52.5%. Additionally, we compare our detection and tracking methods to human performance on external data in studying the motility of muscle stem cells with different physiological and molecular stimuli. We believe that our method has the potential to improve the accuracy and efficiency of cell tracking and motility analysis. This could lead to significant advances in biomedical research and medical diagnosis. Our code is made publicly available on GitHub
1
.
更多查看译文
关键词
cell tracking,deep learning,detection,ensemble,multiobject tracking,deformable object tracking
AI 理解论文
溯源树
样例
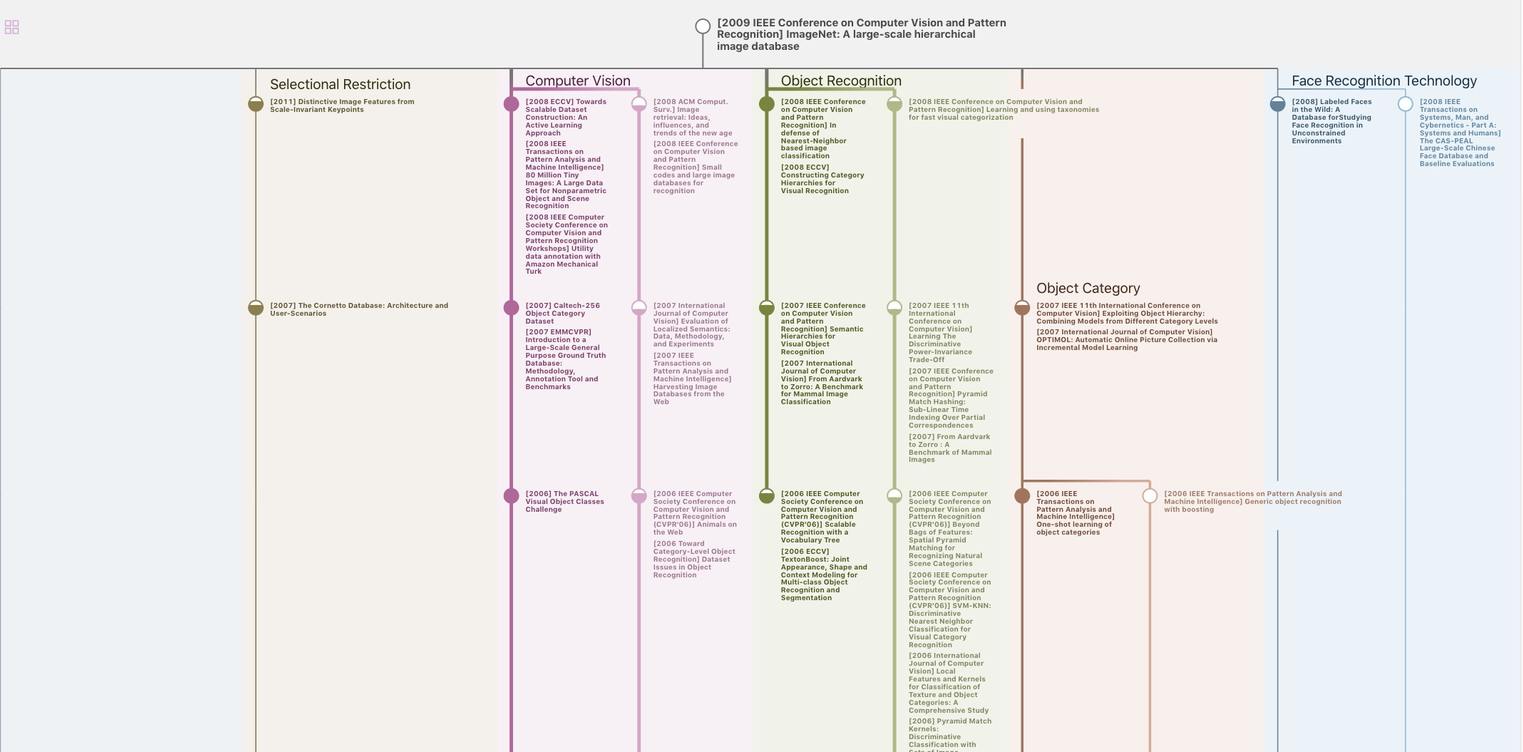
生成溯源树,研究论文发展脉络
Chat Paper
正在生成论文摘要