Higher Chest X-ray Resolution Improves Classification Performance
arXiv (Cornell University)(2023)
摘要
Deep learning models for image classification are often trained at a resolution of 224 x 224 pixels for historical and efficiency reasons. However, chest X-rays are acquired at a much higher resolution to display subtle pathologies. This study investigates the effect of training resolution on chest X-ray classification performance, using the chest X-ray 14 dataset. The results show that training with a higher image resolution, specifically 1024 x 1024 pixels, results in the best overall classification performance with a mean AUC of 84.2 % compared to 82.7 % when trained with 256 x 256 pixel images. Additionally, comparison of bounding boxes and GradCAM saliency maps suggest that low resolutions, such as 256 x 256 pixels, are insufficient for identifying small pathologies and force the model to use spurious discriminating features. Our code is publicly available at https://gitlab.lrz.de/IP/cxr-resolution
更多查看译文
关键词
chest,resolution,classification,performance,x-ray
AI 理解论文
溯源树
样例
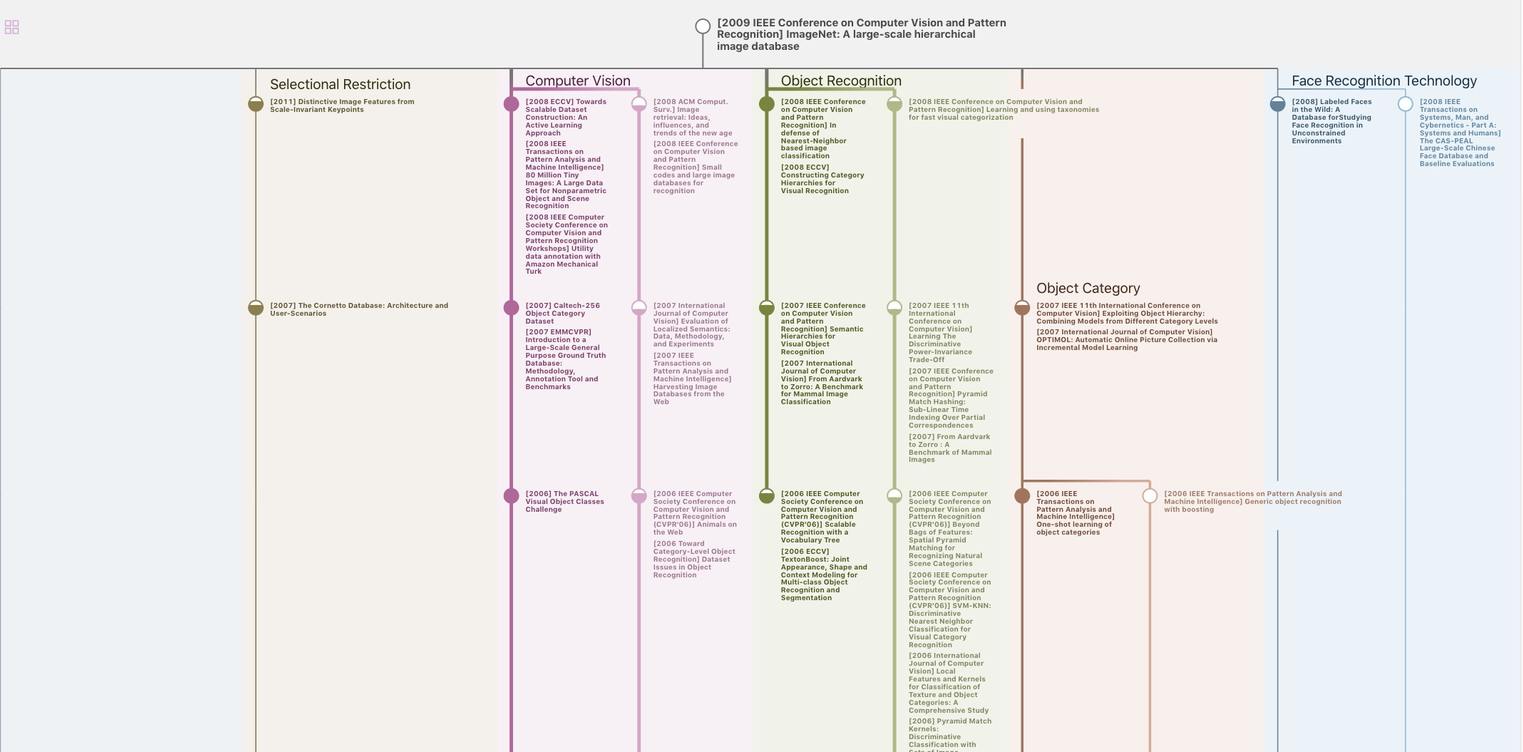
生成溯源树,研究论文发展脉络
Chat Paper
正在生成论文摘要