AMS-PAN: Breast ultrasound image segmentation model combining attention mechanism and multi-scale features
Biomedical Signal Processing and Control(2023)
摘要
Breast ultrasound medical images are characterized by poor imaging quality and irregular target edges. During the diagnosis process, it is difficult for physicians to segment tumors manually, and the segmentation accuracy required for diagnosis is high, so there is an urgent need for an automated method to improve the segmentation accuracy as a technical tool to assist diagnosis. This study designed an improved Pyramid Attention Network combining Attention mechanism and Multi-Scale features (AMS-PAN) for breast ultrasound image segmentation. On the encoding side, the model adopts the depthwise separable convolution strategy to achieve a multi-scale receptive field with cumulative small-size convolution, which performs multi-dimensional feature extraction and forms a feature pyramid. The model uses Global Attention Upsample (GAU) feature fusion on the decoding side. In order to further process the fused feature information, the proposed method uses a Spatial and Channel Attention (SCA) module to shift the model’s segmentation focus to the edge texture information. The good segmentation performance of our method is verified through experiments on BUSI and OASBUD. All the designed parts have contributed to the segmentation performance in practical applications. Compared with the traditional non-deep learning methods and the current mainstream deep learning methods, the improvement of the model in Dice and IoU metrics is pronounced. AMS-PAN has high computational efficiency, and its good performance has been proven to play a role in ultrasound detection tasks of breast tumors for physicians to specific auxiliary diagnostic roles to guide the subsequent diagnosis and treatment services for patients.
更多查看译文
关键词
attention mechanism,segmentation,breast,ams-pan,multi-scale
AI 理解论文
溯源树
样例
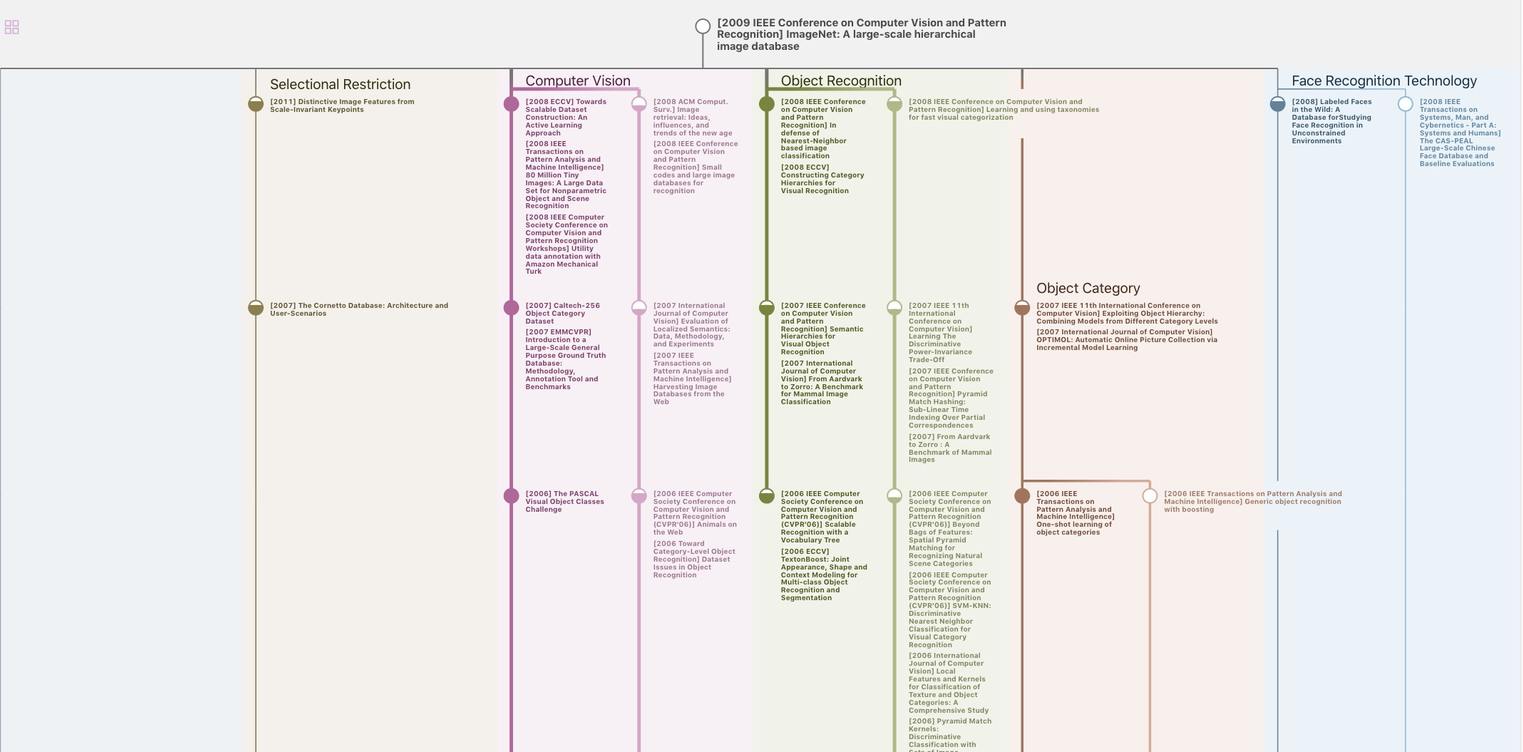
生成溯源树,研究论文发展脉络
Chat Paper
正在生成论文摘要