Causality-based Fair Multiple Decision by Response Functions
ACM TRANSACTIONS ON KNOWLEDGE DISCOVERY FROM DATA(2024)
Key words
Fairness,causality,multiple decision learning,hidden confounders and unidentifiable issues,response-function variables
AI Read Science
Must-Reading Tree
Example
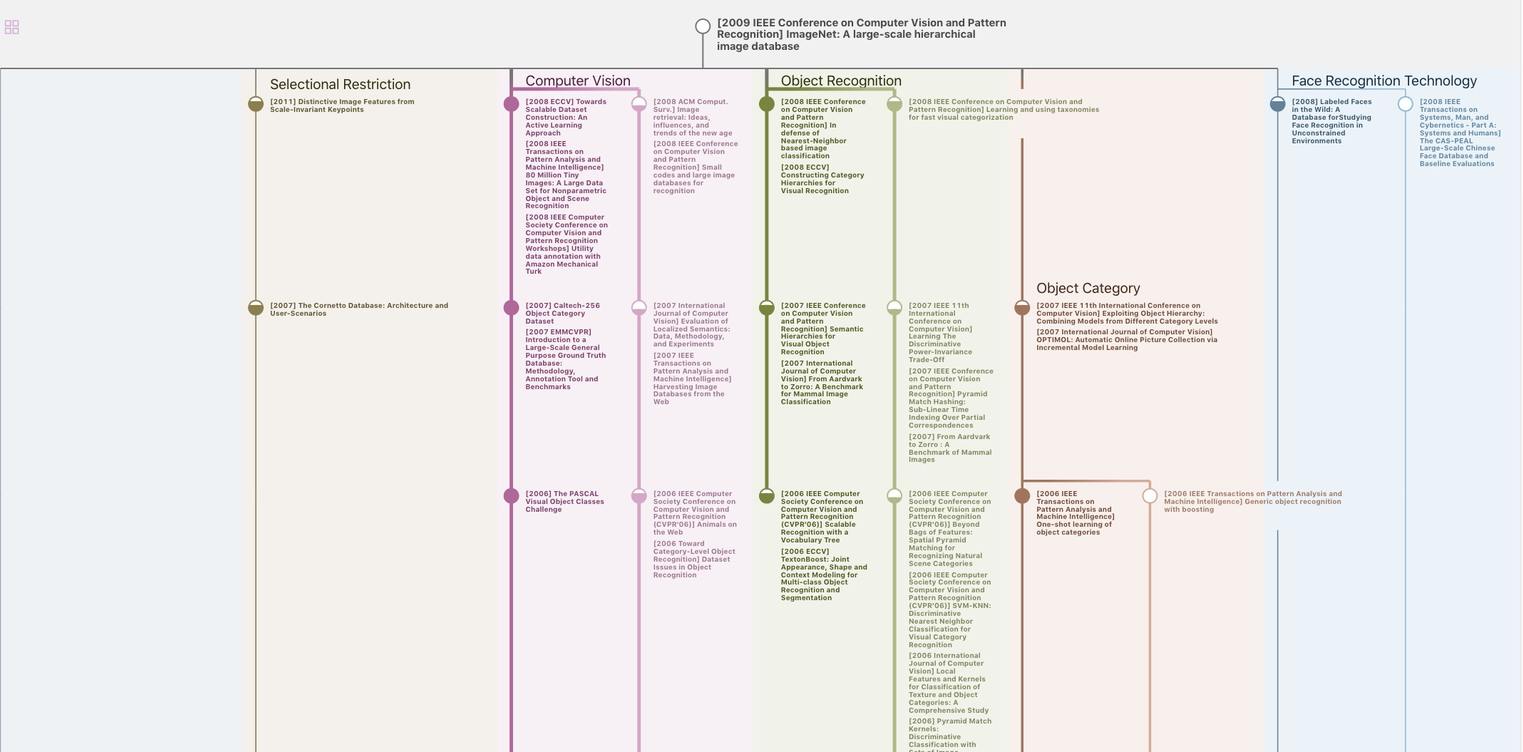
Generate MRT to find the research sequence of this paper
Chat Paper
Summary is being generated by the instructions you defined