Towards Adaptive Multi-Scale Intermediate Domain via Progressive Training for Unsupervised Domain Adaptation
IEEE TRANSACTIONS ON MULTIMEDIA(2024)
摘要
Unsupervised domain adaptation (UDA) involves the transfer of knowledge from a labelled source domain to an unlabelled target domain. Recent studies have introduced the concept of intermediate domains to handle significant domain discrepancies between source and target domains. Constructing an appropriate intermediate domain is a crucial step in handling scenarios with substantial domain differences. In this article, we propose a novel progressive UDA method called adaptive multi-scale intermediate domain via progressive training (AMPT), which has achieved remarkable effectiveness in alleviating large discrepancies between domains. We design a multi-scale similarity metrics module to solve the issue of different scales across different domains by simultaneously computing the pairwise distance between source domain images and the target domain images on multiple scales. Furthermore, we explore a progressive training strategy that facilitates smooth adaptation of the source domain to the target domain by utilizing the intermediate domain. During the progressive training process, considering a positive feedback mechanism, we iteratively leverage task losses and distillation loss through a dynamic threshold. This ensures that the trained intermediate domain branch progressively constrains the target domain branch, and the intermediate domain can be generated dynamically, leading to a smoother gradual adaptation from the source domain to the target domain. Extensive experimental results demonstrate the superiority of our proposed method AMPT on well-known UDA datasets, including Office-Home, Office-31 and DomainNet.
更多查看译文
关键词
Intermediate domain,multi-scale,progressive training,unsupervised domain adaptation
AI 理解论文
溯源树
样例
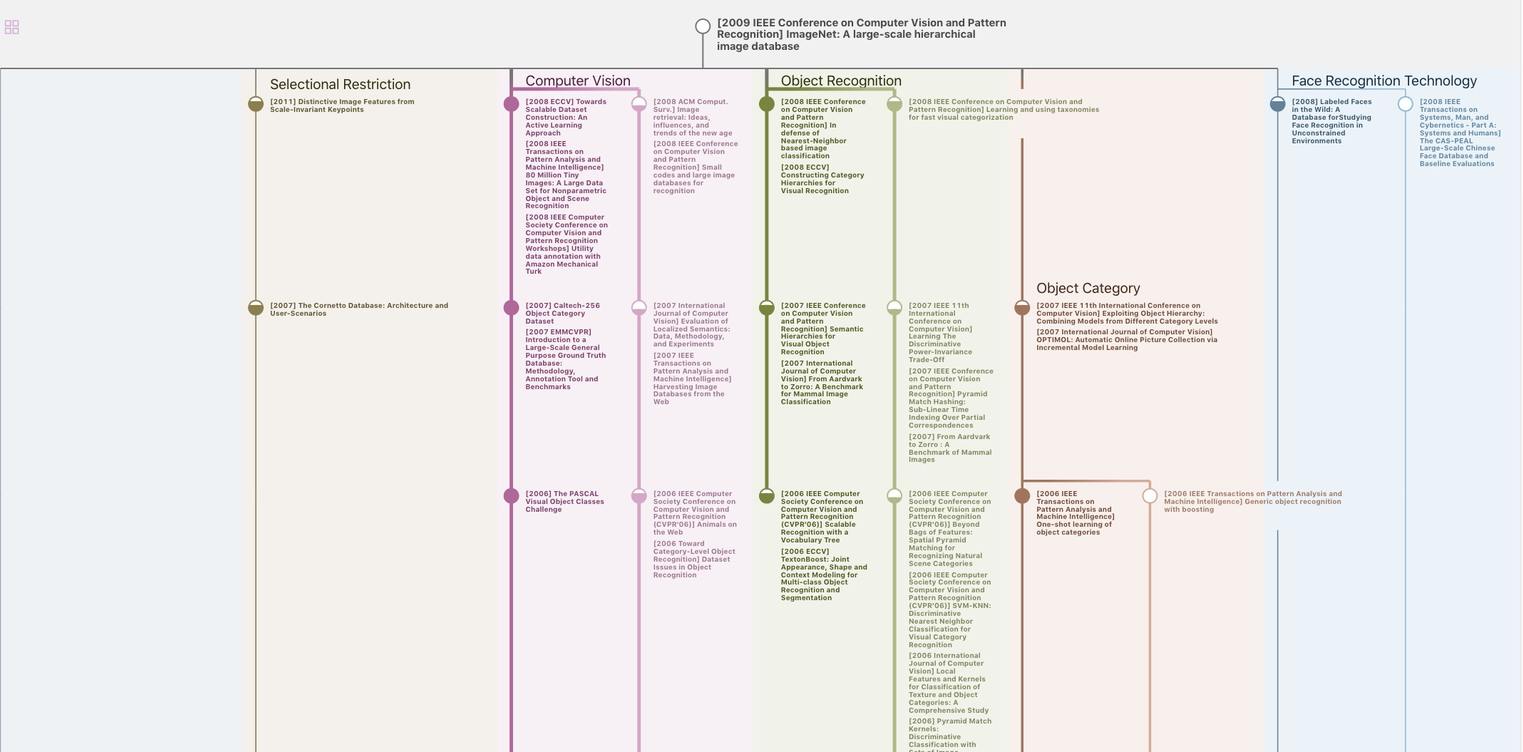
生成溯源树,研究论文发展脉络
Chat Paper
正在生成论文摘要