Improved Demonstration-Knowledge Utilization in Reinforcement Learning
IEEE transactions on artificial intelligence(2023)
摘要
Reinforcement learning has made great success in recent years. Generally, the learning process requires a huge amount of interaction with the environment before an agent can achieve acceptable performance. This motivates many techniques, such as incorporating prior knowledge which is usually presented as experts’ demonstration, and using a probability distribution to represent state-and-action values, to accelerate the learning process. The methods perform well when the prior knowledge is genuinely correct and no much change occurs to the learning environment. However, the requirement is not perfectly realistic in many complex applications. The demonstration knowledge may not reflect the true environment and even be full of noise. In this paper, we introduce a dynamic distribution merging method to improve knowledge utilization in a general reinforcement learning algorithm, namely Q-learning. The new method adapts a normal distribution to represent state-action values and merges the prior and learned knowledge in a discriminative way. We theoretically analyze the new learning method and demonstrate its empirical performance over multiple problem domains.
更多查看译文
关键词
reinforcement learning,demonstration-knowledge
AI 理解论文
溯源树
样例
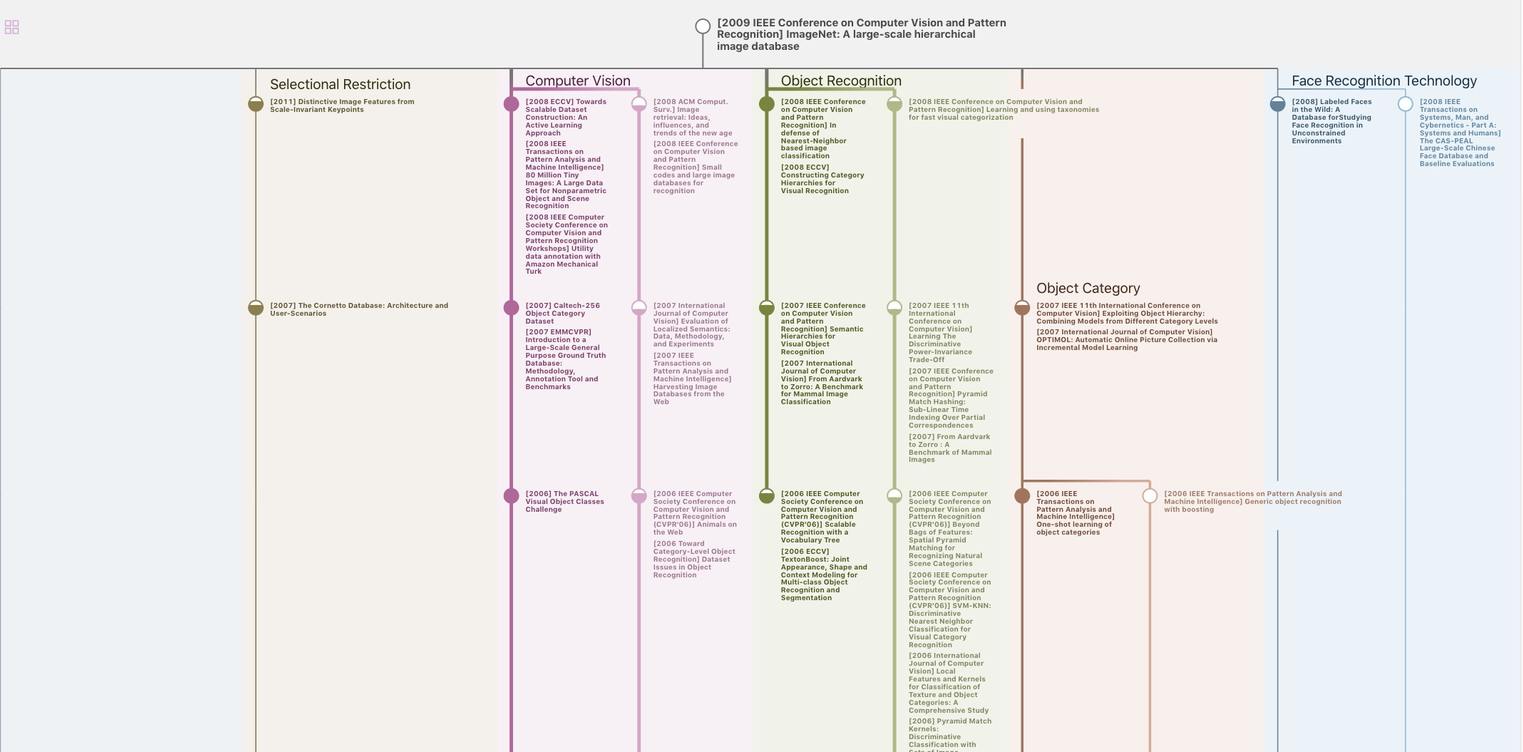
生成溯源树,研究论文发展脉络
Chat Paper
正在生成论文摘要