fMRI-PTE: A Large-scale fMRI Pretrained Transformer Encoder for Multi-Subject Brain Activity Decoding
arXiv (Cornell University)(2023)
摘要
The exploration of brain activity and its decoding from fMRI data has been a longstanding pursuit, driven by its potential applications in brain-computer interfaces, medical diagnostics, and virtual reality. Previous approaches have primarily focused on individual subject analysis, highlighting the need for a more universal and adaptable framework, which is the core motivation behind our work. In this work, we propose fMRI-PTE, an innovative auto-encoder approach for fMRI pre-training, with a focus on addressing the challenges of varying fMRI data dimensions due to individual brain differences. Our approach involves transforming fMRI signals into unified 2D representations, ensuring consistency in dimensions and preserving distinct brain activity patterns. We introduce a novel learning strategy tailored for pre-training 2D fMRI images, enhancing the quality of reconstruction. fMRI-PTE's adaptability with image generators enables the generation of well-represented fMRI features, facilitating various downstream tasks, including within-subject and cross-subject brain activity decoding. Our contributions encompass introducing fMRI-PTE, innovative data transformation, efficient training, a novel learning strategy, and the universal applicability of our approach. Extensive experiments validate and support our claims, offering a promising foundation for further research in this domain.
更多查看译文
关键词
encoder,brain,fmri-pte,large-scale,multi-subject
AI 理解论文
溯源树
样例
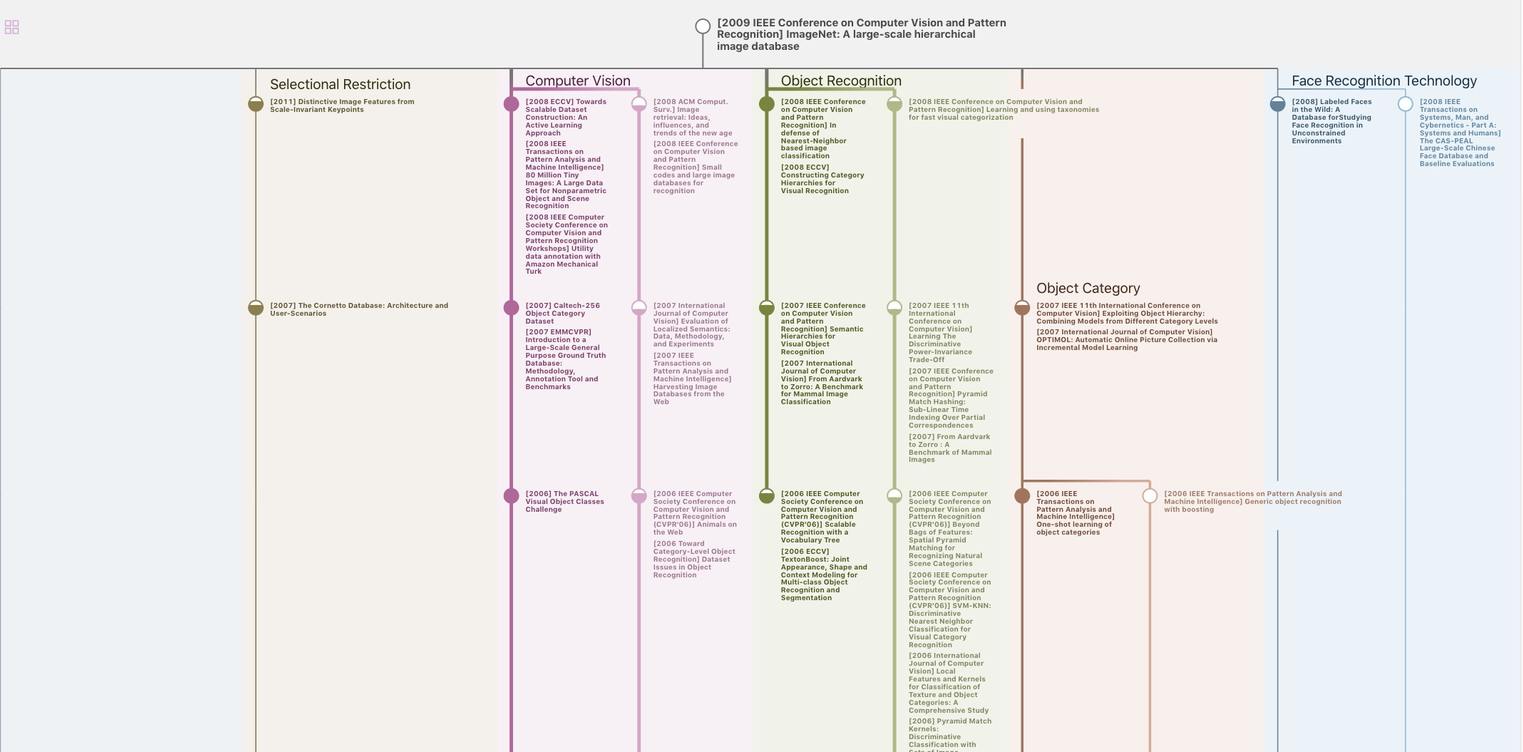
生成溯源树,研究论文发展脉络
Chat Paper
正在生成论文摘要