Machine Learning Predicts metastasis of cT1 solid lung cancer
Lung cancer(2023)
摘要
Introduction or background: There are some clinical T1 stage (cT1) lung cancers with metastases at initial diagnosed, which is harm to human health. Incorrect assessments may lead to delayed diagnosis and increase the risk of complications. Objectives: This study aims to construct a practical, robust and non-invasive model to better predict metastasis in cT1 solid lung cancer. Methods: 148 cases non-metastatic and 138 cases metastasis cT1 solid lung cancer were included in our study. Random Forest model, AdaBoost model and Gradient Boosting model were developed and validated, with pair-wise Pearson correlation analysis and BH (Benjamini Hochberg) adjustment of 9 clinical features (Carcinoembryonic antigen, Nodule diameter, Cytokeratin 19 fragment antigen21-1, Neuron specific enolase, Squamous cell carcinoma antigen, Age, Nodules numbers, Nodules location, Sex) Results: With repeating 10-folds cross-validation applied in model training, we obtained the best hyperparameters of three classifiers. Eventually, our Random Forest model yielded an accuracy of 0.89 with AUC of 0.92 (95%CI: 0.88-0.94) compared with Gradient Boosting and AdaBoost classifiers in internal testing dataset, yielded accuracy of 0.84 and 0.77 with AUC of 0.87 (95%CI: 0.84-0.93) and 0.90 (95%CI: 0.86-0.92), respectively. Conclusions: We developed a classifier for metastasis of cT1 lung cancer and validated its accuracy in our internal testing dataset. Furthermore, we embedded this classifier in a web application (http:// 192.168.181.134/PNMPre/), a user-friendly tool assisting in predicting metastasis of cT1 solid lung cancer. It could provide more precise treatment for cT1 solid lung cancer at higher risk of metastasis.
更多查看译文
关键词
lung cancer,machine learning,ct1
AI 理解论文
溯源树
样例
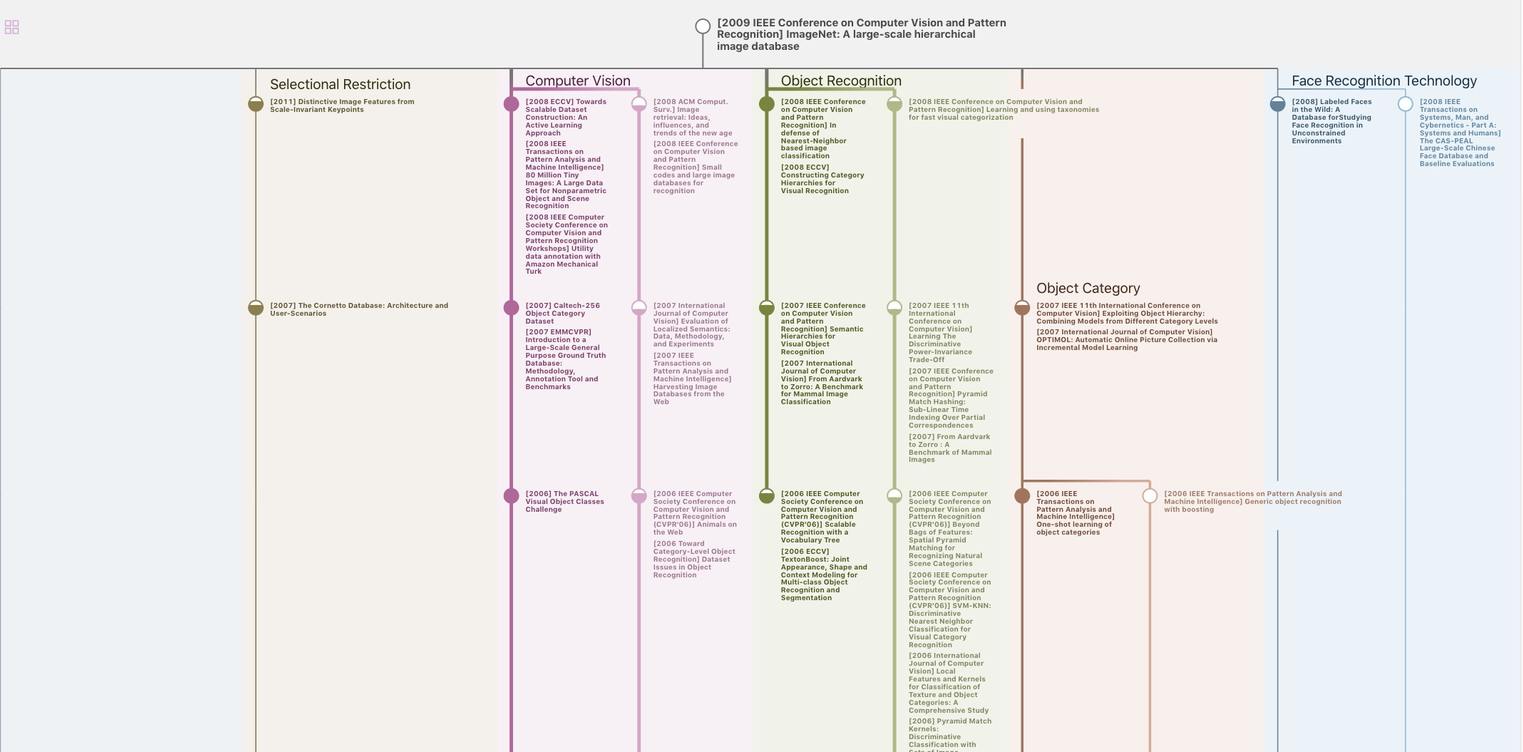
生成溯源树,研究论文发展脉络
Chat Paper
正在生成论文摘要