Simplified Learned Approximate Message Passing Network for Beamspace Channel Estimation in mmWave Massive MIMO Systems
IEEE Transactions on Wireless Communications(2023)
摘要
Millimeter-wave (mmWave) communication using lens antenna array has emerged as a promising techique for the next generation wireless communication systems, which has the advantage of considerably reducing the number of required radio-frequency (RF) chains. However, the beamspace channel estimation is a challenging problem due to the fact that the number of RF chains is much smaller than that of transmit/receive antennas at the base station (BS). In this paper, motivated by the sparsity structure of lens-antenna based beamspace channels, we first model beamspace channel estimation problem as a sparse signal recovery problem, which can be solved by the compressed sensing (CS) algorithms. Then, we propose a simplified learned approximate message passing (SL-AMP) network by pre-learning the prior parameters of beamspace channel. Specifically, we use the Wasserstein generative adversarial net (GAN) to learn the underlying channel distribution and generate the corresponding samples to address the lack of training data problem. Next we choose the Bernoulli Gaussian mixture distribution to capture the channel prior and derive the corresponding shrinkage function. Finally, simulations results show that the proposed SL-AMP network can achieve relatively better performance with much lower training complexity compared with the existing LAMP network.
更多查看译文
关键词
Beamspace channel estimation,millimeter wave,massive MIMO,generative adversarial net (GAN),approximate message passing (AMP),deep learning
AI 理解论文
溯源树
样例
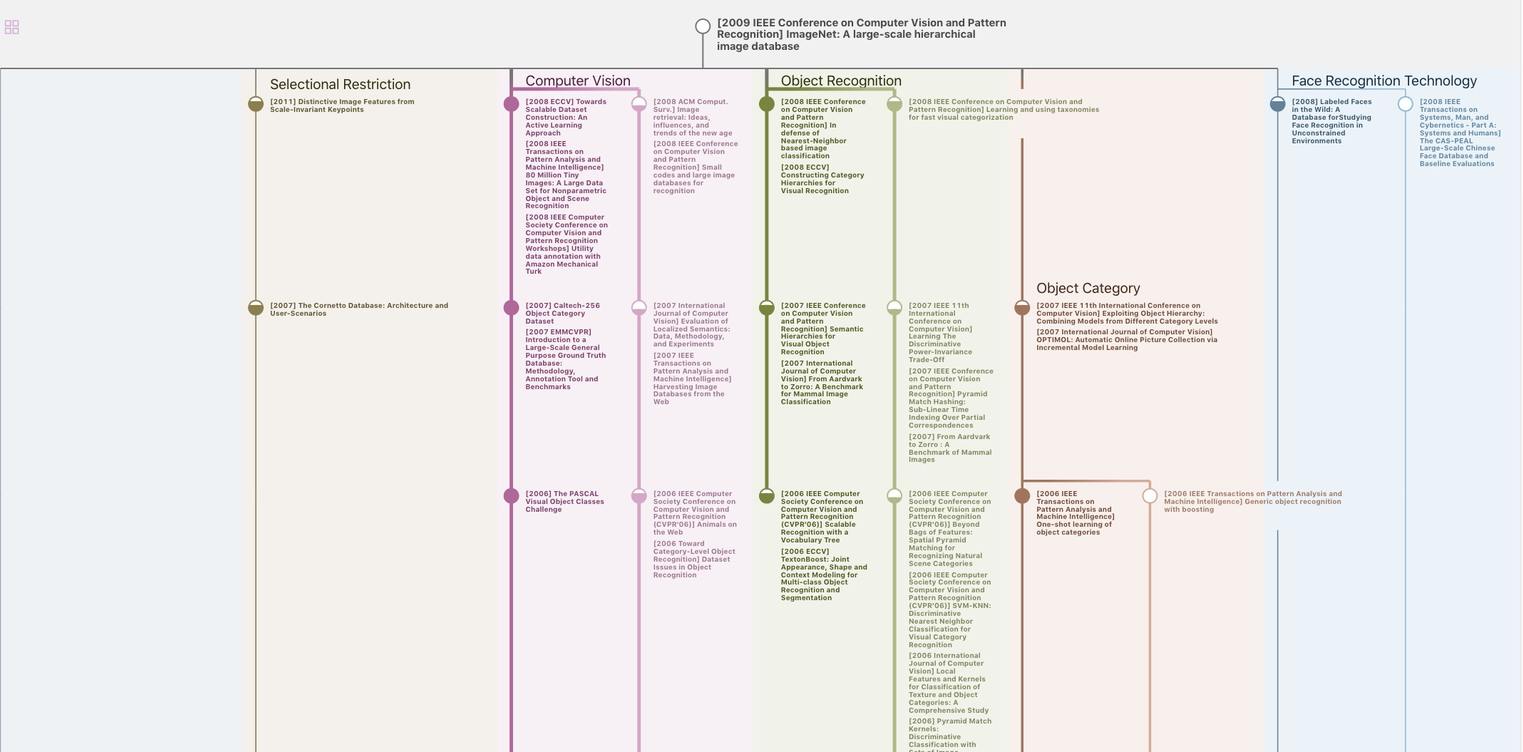
生成溯源树,研究论文发展脉络
Chat Paper
正在生成论文摘要