Deep-Learning-Based Radio Map Reconstruction for V2X Communications
IEEE TRANSACTIONS ON VEHICULAR TECHNOLOGY(2024)
Abstract
Radio environment map (REM) reconstruction based on large-scale channel measurements is a promising technology for future mobility services involving vehicle-to-everything (V2X) communications. REMs provide contextual information which can be exploited to reduce V2X communication latency and control signaling, for instance, through a fast access to channel state information. However, the accuracy of radio mapping techniques is limited by the availability of measurements, which require for collection significant signaling overhead. Moreover, mobility scenarios impose strict latency constraints that render fast channel acquisition a challenging problem. This paper presents a low-complexity deep-learning-based approach based on long-short term memory (LSTM) cells for REM reconstruction on roads, addressed as a data-filling problem. To improve model generalization, the network is trained on a virtually infinite dataset generated according to a 3GPP-compliant freeway scenario, considering different correlation properties and missing point configurations. The results show that the proposed approach provides a performance closer to the theoretical lower bound than the classical Ordinary Kriging spatial interpolation method, without increasing the complexity order. Experiments performed in realistic scenarios using a 3D city model confirm the generalization capability of the proposed solution.
MoreTranslated text
Key words
Deep learning,radio environment maps (REMs),RNN,vehicle-to-everything (V2X),vehicular communications
AI Read Science
Must-Reading Tree
Example
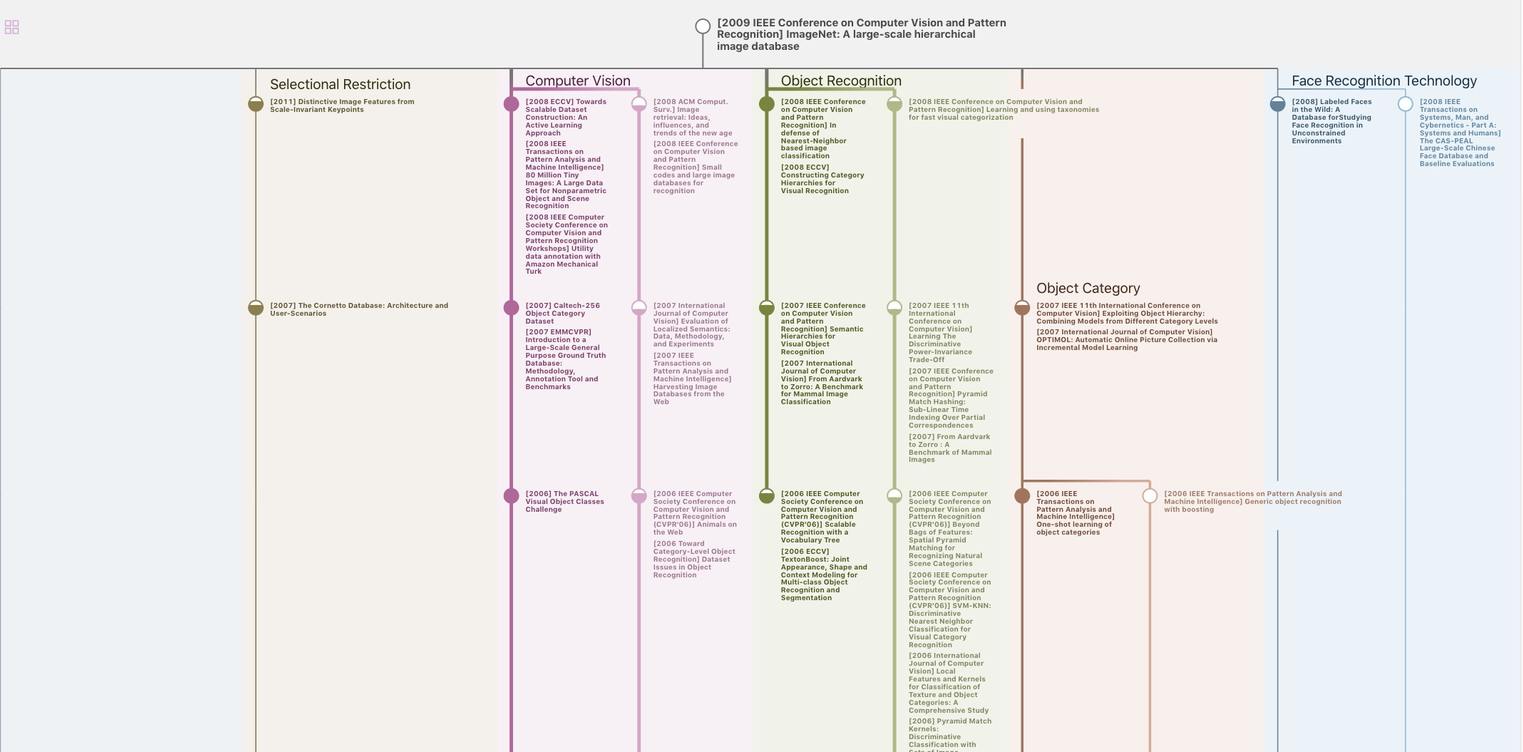
Generate MRT to find the research sequence of this paper
Chat Paper
Summary is being generated by the instructions you defined