Adaptive Activation Network for Weakly Supervised Semantic Segmentation.
IEEE Trans. Multim.(2024)
摘要
Class activation maps generated by image classifiers are widely used as priors for image-level weakly supervised semantic segmentation. However, these activation maps mainly focus on the sparse discriminative regions, which has been a bottleneck for the segmentation task. Based on our observations, the activation maps actually capture almost the entire target regions, and some regions with lower activation values are easily to be neglected. Thus, to solve the issue, we propose an adaptive activation network with two branches to recalibrate the low-confidence regions in the activation maps. Specifically, an activation enhancement branch is designed to redistribute the activation values by leveraging attention mechanism. Since multi-scale images can provide complementary information, a scale adaptation branch is paralleled to supervise the activation enhancement branch. The mutual supervision and fusion of the two branches can promote the less-discriminative parts, and deactivate the background regions. Based on them, a simple yet effective denoising module is proposed to further improve the quality of pseudo masks, which makes use of the large scale predictions of the trained segmentation network. Extensive experiments on the PASCAL VOC 2012 and MS COCO 2014 benchmarks show that our method achieves state-of-the-art performance, demonstrating the effectiveness of our algorithm. Code will be made publicly available.
更多查看译文
关键词
Weakly supervised semantic segmentation,class activation maps,discriminative region,adaptive activation,scale adaptation
AI 理解论文
溯源树
样例
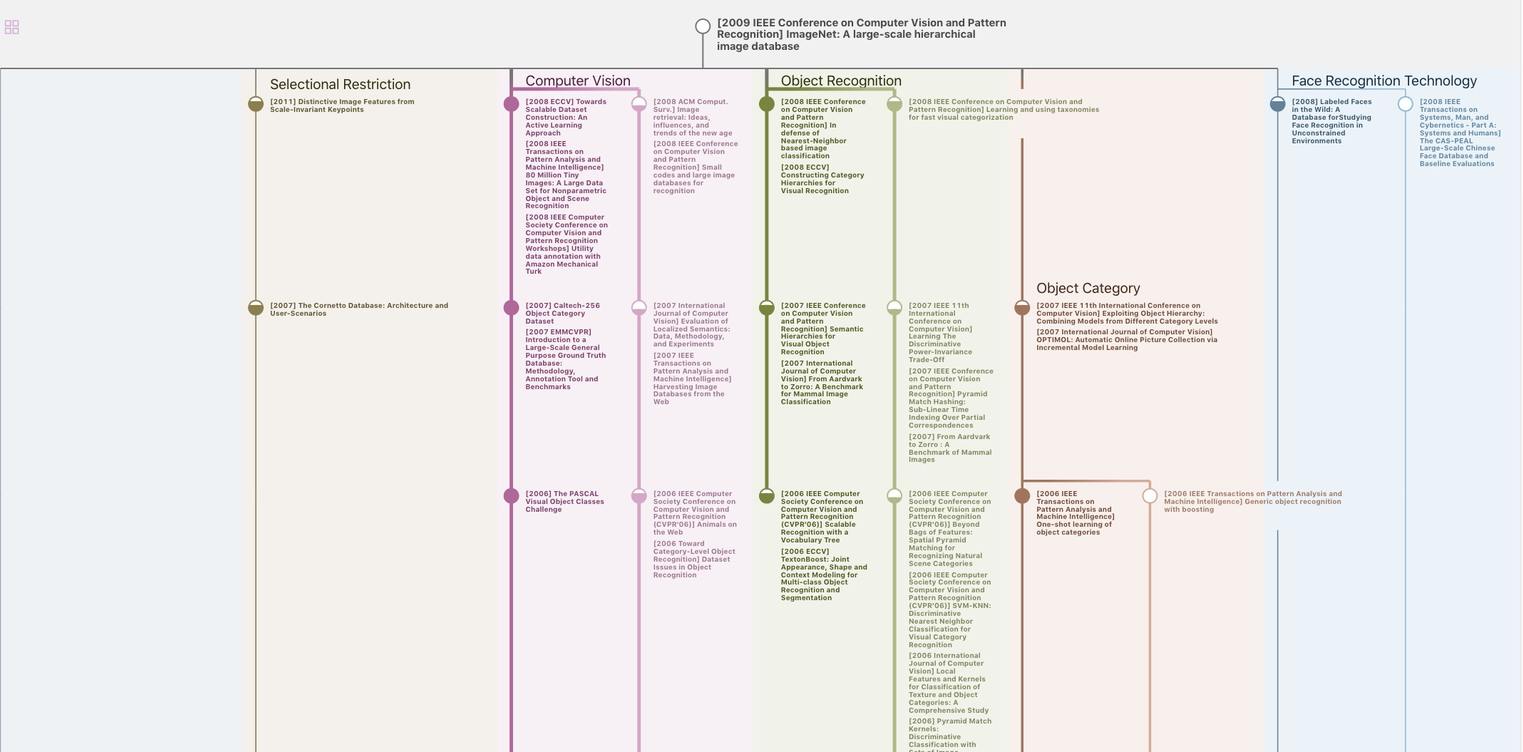
生成溯源树,研究论文发展脉络
Chat Paper
正在生成论文摘要