Automated Identification of Differential-Variational Equations for Static Systems
JOURNAL OF APPLIED MECHANICS-TRANSACTIONS OF THE ASME(2024)
摘要
Data-driven equation identification for dynamical systems has achieved great progress, which for static systems, however, has not kept pace. Unlike dynamical systems, static systems are time invariant, so we cannot capture discrete data along the time stream, which requires identifying governing equations only from scarce data. This work is devoted to this topic, building a data-driven method for extracting the differential-variational equations that govern static behaviors only from scarce, noisy data of responses, loads, as well as the values of system attributes if available. Compared to the differential framework typically adopted in equation identification, the differential-variational framework, due to its spatial integration and variation arbitrariness, brings some advantages, such as high robustness to data noise and low requirements on data amounts. The application, efficacy, and all the aforementioned advantages of this method are demonstrated by four numerical examples, including three continuous systems and one discrete system.
更多查看译文
关键词
data-driven equation identification,differential-variational framework,static system,sparse optimization,dimensional analysis,elasticity,structures
AI 理解论文
溯源树
样例
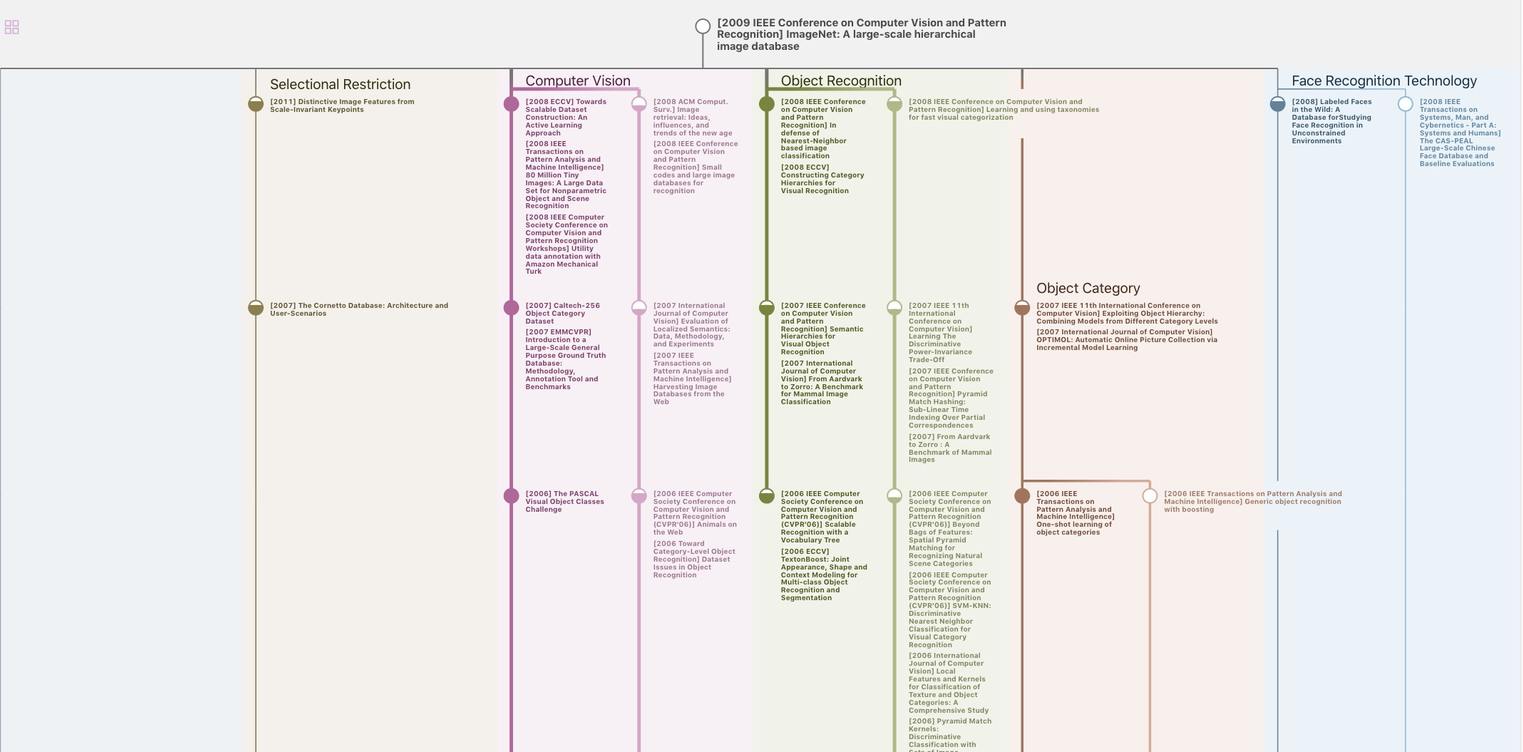
生成溯源树,研究论文发展脉络
Chat Paper
正在生成论文摘要