Consistent-1-to-3: Consistent Image to 3D View Synthesis via Geometry-aware Diffusion Models
arXiv (Cornell University)(2023)
Abstract
Zero-shot novel view synthesis (NVS) from a single image is an essential problem in 3D object understanding. While recent approaches that leverage pre-trained generative models can synthesize high-quality novel views from in-the-wild inputs, they still struggle to maintain 3D consistency across different views. In this paper, we present Consistent-1-to-3, which is a generative framework that significantly mitigate this issue. Specifically, we decompose the NVS task into two stages: (i) transforming observed regions to a novel view, and (ii) hallucinating unseen regions. We design a scene representation transformer and view-conditioned diffusion model for performing these two stages respectively. Inside the models, to enforce 3D consistency, we propose to employ epipolor-guided attention to incorporate geometry constraints, and multi-view attention to better aggregate multi-view information. Finally, we design a hierarchy generation paradigm to generate long sequences of consistent views, allowing a full 360 observation of the provided object image. Qualitative and quantitative evaluation over multiple datasets demonstrate the effectiveness of the proposed mechanisms against state-of-the-art approaches. Our project page is at https://jianglongye.com/consistent123/
MoreTranslated text
Key words
3d view synthesis,consistent-1-to-3 image,diffusion,geometry-aware
AI Read Science
Must-Reading Tree
Example
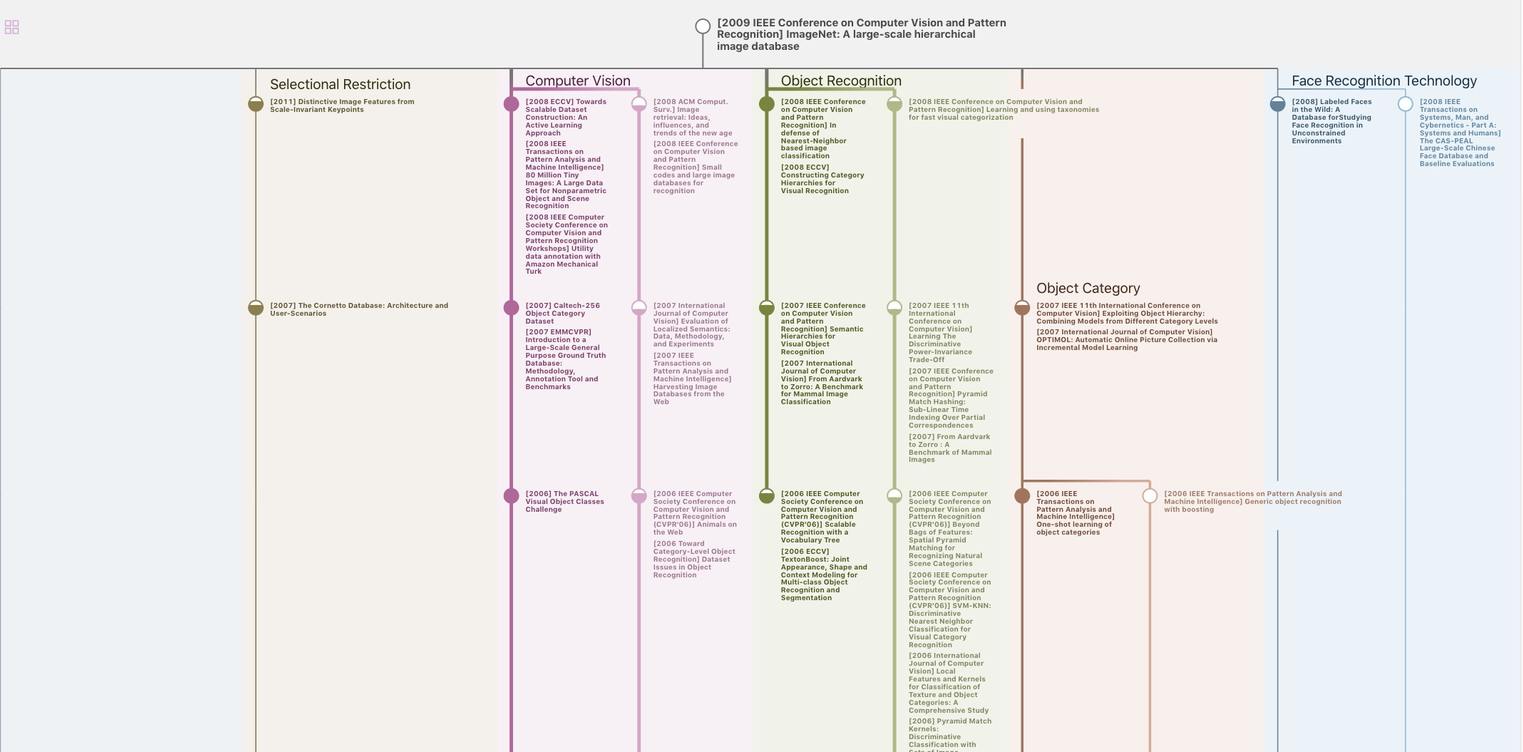
Generate MRT to find the research sequence of this paper
Chat Paper
Summary is being generated by the instructions you defined