A Multi-Graph Neural Networks Based on Dynamic Spatio-temporal for Urban Traffic Flows Prediction
Research Square (Research Square)(2023)
摘要
Abstract The primary function of an intelligent transportation system (ITS) is traffic prediction. Perfect traffic forecasting can largely enhance the use of public incomes. It is, however, difficult due to the dynamic and complex nature of spatial-temporal dependencies. Largely, the existing methods rely on static adjacency matrices, which do not reflect the simultaneous collaboration of several spatial-temporal correlations and therefore limit the accuracy and correctness of forecasting. In order to address this issue, and to illustrate the dynamic interplay of various spatial-temporal correlations in the traffic flows network, this research suggests a unified simultaneous Multi Fusion Graph Network (DMFGNet) model. The dynamic graph fusion in the proposed DMFGNet model is intended to extract dynamic spatial-temporal dependencies among different areas. Meanwhile, the model Spatial-Temporal attention Unit (DSTAU) is proposed to regulate the weights of aggregation of various neighbours. It may carefully aggregate spatiotemporal properties from various neighbours. To display the usefulness and practicality of the suggested model, extensive evaluation and experiments were conducted on two large scale real-world traffic flow data-sets. The experimental findings validate the benefit of the advised model over prevailing state-of-the-art baseline techniques. Furthermore, the outcomes show that the suggested approach is efficient and practical.
更多查看译文
关键词
urban traffic flows prediction,networks,multi-graph,spatio-temporal
AI 理解论文
溯源树
样例
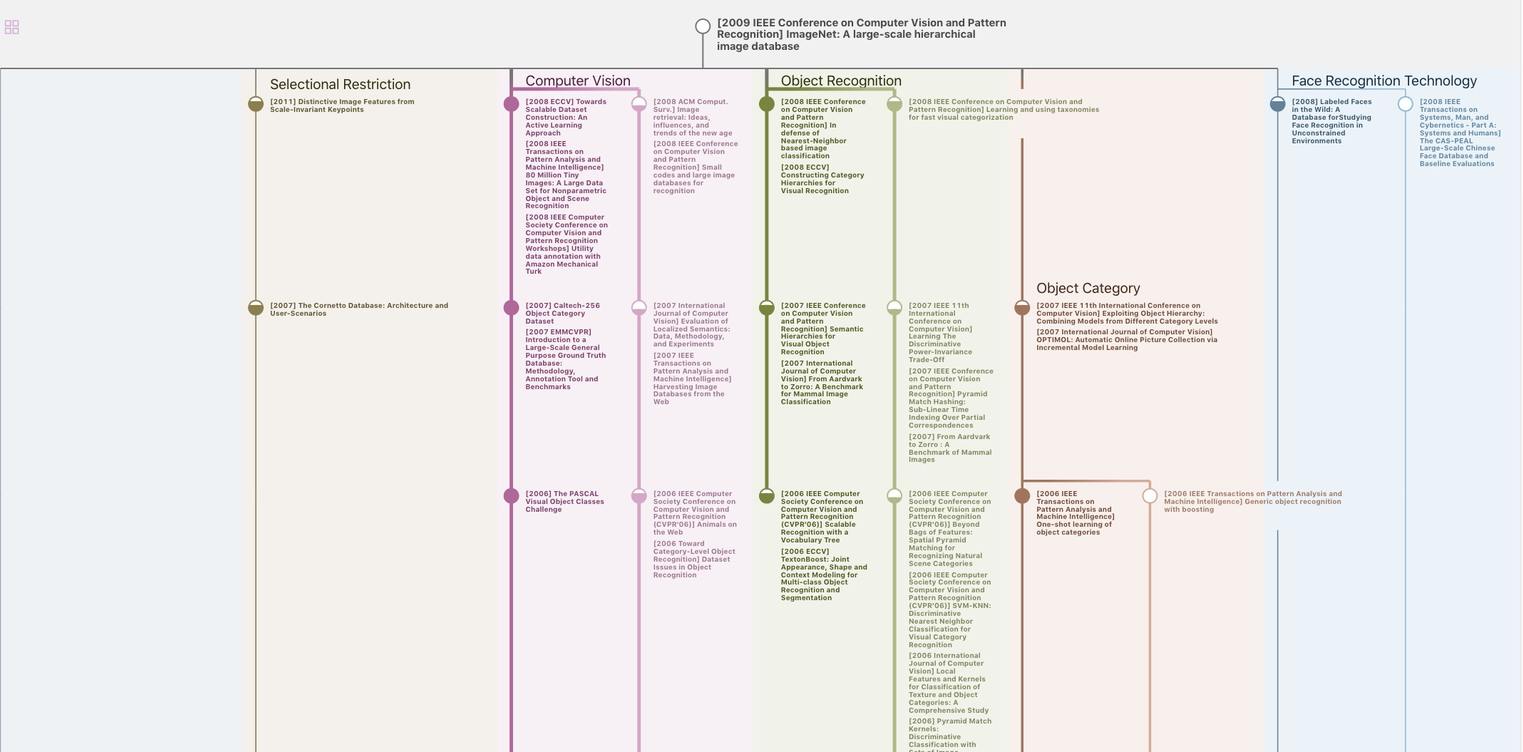
生成溯源树,研究论文发展脉络
Chat Paper
正在生成论文摘要