A linear-programming portfolio optimizer to mean-variance optimization
INTERNATIONAL JOURNAL OF THEORETICAL AND APPLIED FINANCE(2023)
Abstract
In the Markowitz mean-variance portfolio optimization problem, the estimation of the inverse covariance matrix is not trivial and can even be intractable, especially when the dimension is very high. In this paper, we propose a linear-programming portfolio optimizer (LPO) to solve the Markowitz optimization problem in both low-dimensional and high-dimensional settings. Instead of directly estimating the inverse covariance matrix sigma-1, the LPO method estimates the portfolio weights sigma-1 mu through solving an l1-constrained optimization problem. Moreover, we further prove that the LPO estimator asymptotically yields the maximum expected return while preserving the risk constraint. To offer a practical insight into the LPO approach, we provide a comprehensive implementation procedure of estimating portfolio weights via the Dantzig selector with sequential optimization (DASSO) algorithm and selecting the sparsity parameter through cross-validation. Simulations on both synthetic data and empirical data from Fama-French and the Center for Research in Security Prices (CRSP) databases validate the performance of the proposed method in comparison with other existing proposals.
MoreTranslated text
Key words
Markowitz mean-variance portfolio optimization,sparsity,asymptotic consistency
AI Read Science
Must-Reading Tree
Example
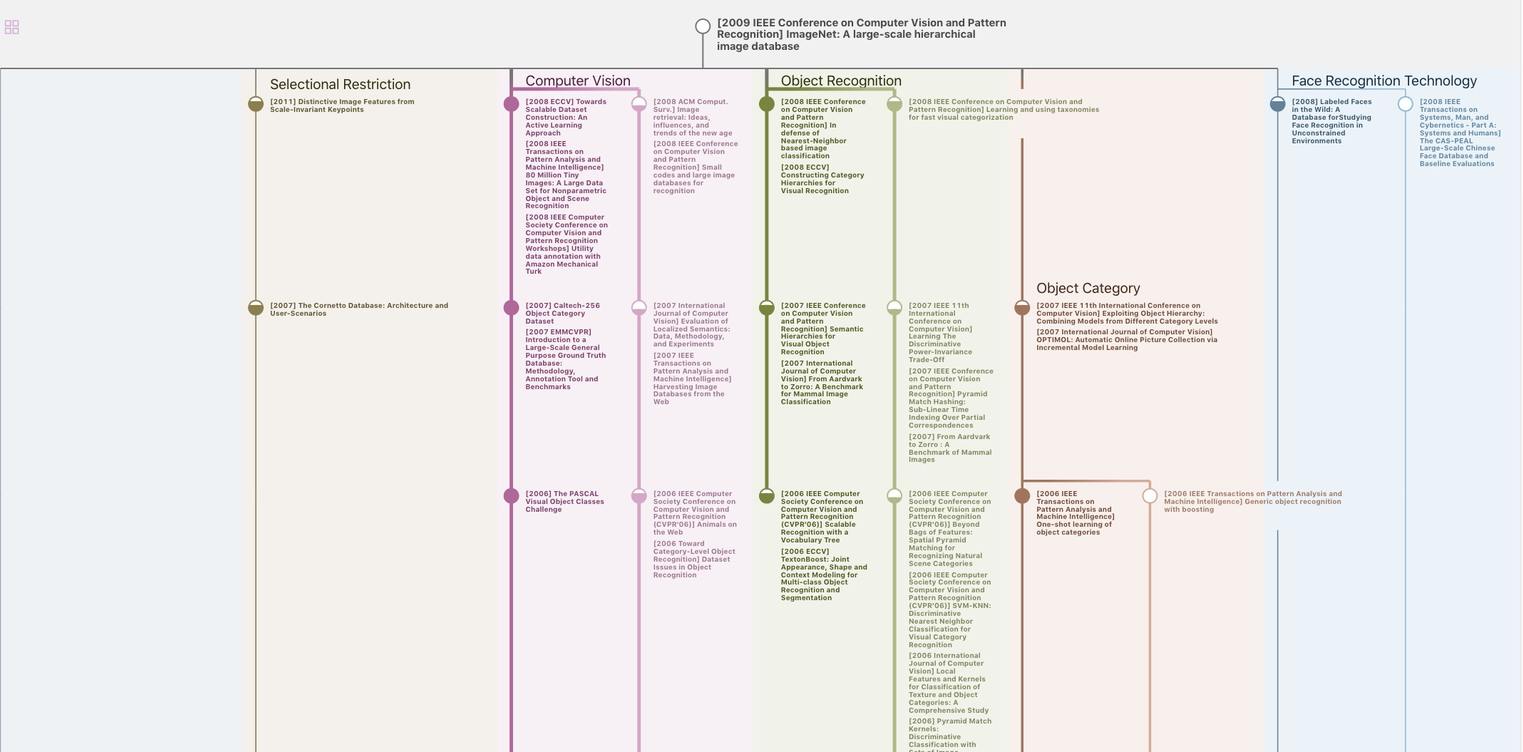
Generate MRT to find the research sequence of this paper
Chat Paper
Summary is being generated by the instructions you defined