NineRec: A Benchmark Dataset Suite for Evaluating Transferable Recommendation
arXiv (Cornell University)(2023)
摘要
Learning a recommender system model from an item's raw modality features (such as image, text, audio, etc.), called MoRec, has attracted growing interest recently. One key advantage of MoRec is that it can easily benefit from advances in other fields, such as natural language processing (NLP) and computer vision (CV). Moreover, it naturally supports transfer learning across different systems through modality features, known as transferable recommender systems, or TransRec. However, so far, TransRec has made little progress, compared to groundbreaking foundation models in the fields of NLP and CV. The lack of large-scale, high-quality recommendation datasets poses a major obstacle. To this end, we introduce NineRec, a TransRec dataset suite that includes a large-scale source domain recommendation dataset and nine diverse target domain recommendation datasets. Each item in NineRec is represented by a text description and a high-resolution cover image. With NineRec, we can implement TransRec models in an end-to-end training manner instead of using pre-extracted invariant features. We conduct a benchmark study and empirical analysis of TransRec using NineRec, and our findings provide several valuable insights. To support further research, we make our code, datasets, benchmarks, and leaderboards publicly available at https://github.com/westlake-repl/NineRec.
更多查看译文
关键词
benchmark dataset suite,recommendation
AI 理解论文
溯源树
样例
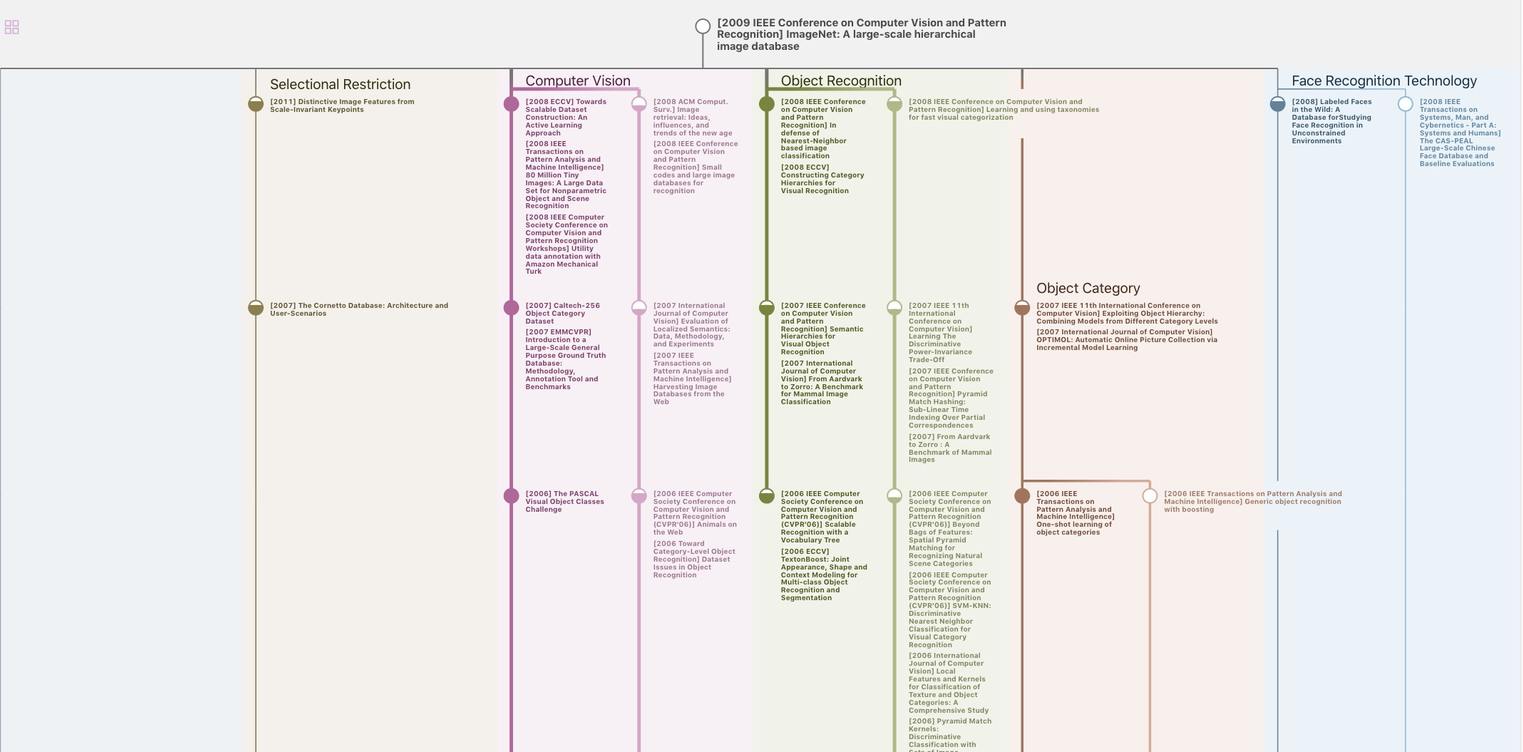
生成溯源树,研究论文发展脉络
Chat Paper
正在生成论文摘要