Planning-Inspired Hierarchical Trajectory Prediction via Lateral-Longitudinal Decomposition for Autonomous Driving.
IEEE Trans. Intell. Veh.(2024)
摘要
Trajectory prediction plays a crucial role in bridging the gap between perception and planning in autonomous driving systems. However, most existing methods perform motion forecasting directly in the coupled spatiotemporal space but disregard a more fundamental and faithful interpretation of path intentions. To address this challenge, we propose a novel Planning-inspired Hierarchical (PiH) trajectory prediction framework that selects path and goal intentions through a hierarchical lateral and longitudinal decomposition. For path selection, we propose a hybrid lateral predictor to choose fixed-distance lateral paths from a candidate set of map-based road-following paths and cluster-based free-move paths. For goal selection, we propose a lateral-conditional longitudinal predictor to choose plausible goals by sampling from the selected lateral paths. Finally, we incorporate lateral-longitudinal information to generate final future trajectories based on a category distribution of path-goal intentions. Experimental results demonstrate that PiH achieves competitive and well-balanced performance compared to state-of-the-art methods on both the Argoverse and the Waymo Open Motion Dataset.
更多查看译文
关键词
Autonomous driving,Trajectory prediction,Anchor-based prediction
AI 理解论文
溯源树
样例
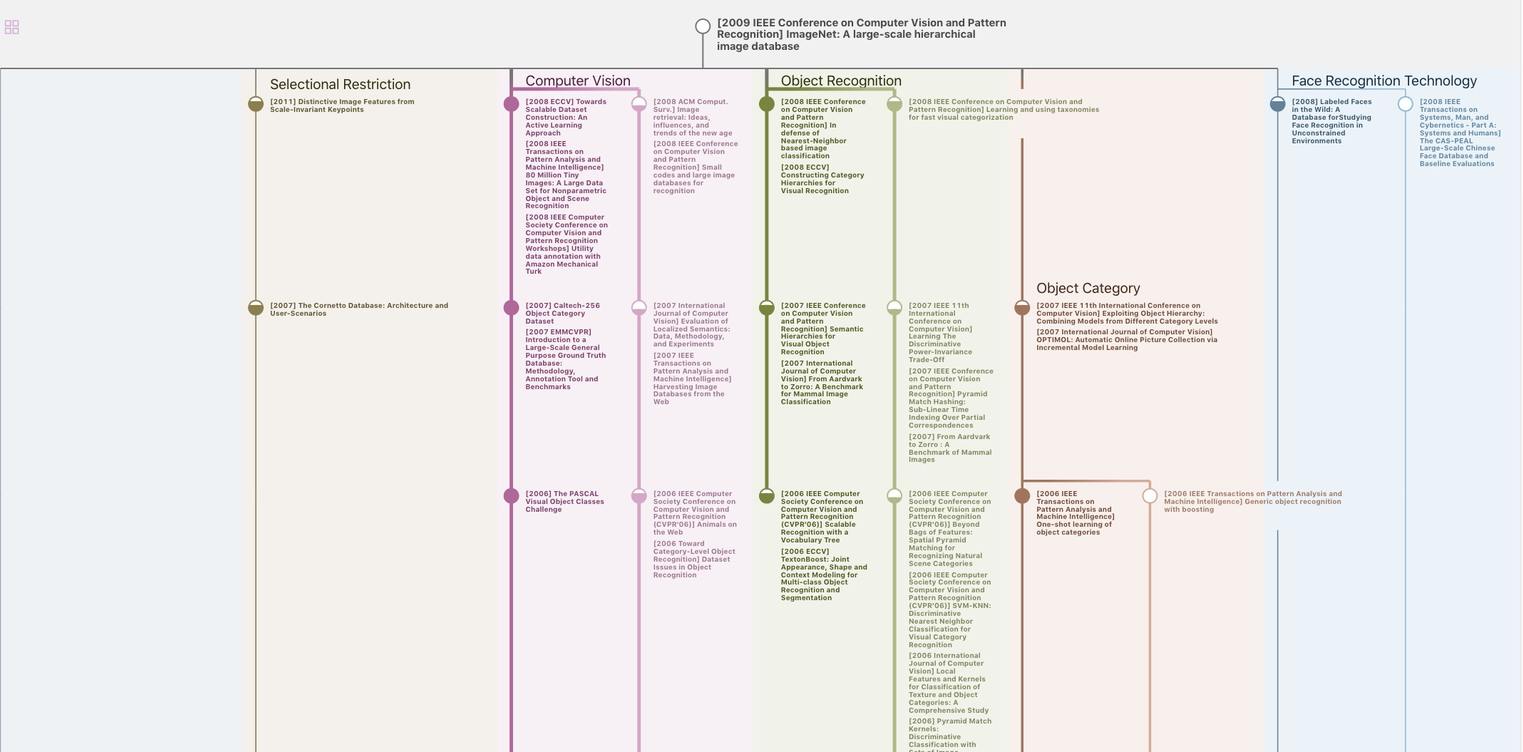
生成溯源树,研究论文发展脉络
Chat Paper
正在生成论文摘要