Forecasting Tourist Arrivals Using a Combination of Long Short-Term Memory and Fourier Series
Lecture notes on data engineering and communications technologies(2023)
摘要
The sector that contributes most to the nation's economy nowadays is tourism. Policymakers, decision-makers, and organisations involved in the tourist sector can use tourism demand forecasting to gather important information for planning and making important decisions. However, it is difficult to produce an accurate forecast because tourism data is critical, especially when a periodic pattern, such as seasonality, trends, and non-linearity, is present in a dataset. In this research, we present a hybrid modelling approach for modelling tourist arrivals time series data that combines the long short-term memory (LSTM) with the Fourier series method. This method is proposed to capture the components of seasonality and trend in the data set. Various single models, such as ARIMA and LSTM, as well as a modified ARIMA model based on Fourier series, are evaluated to confirm the suggested model's accuracy. The efficiency of the proposed model is compared using monthly tourism arrivals data from Langkawi Island, which has a notable pattern and seasonality. The findings reveal that the proposed model is more reliable than the other models in forecasting tourist arrivals series.
更多查看译文
关键词
tourist arrivals,forecasting,fourier series,memory,short-term
AI 理解论文
溯源树
样例
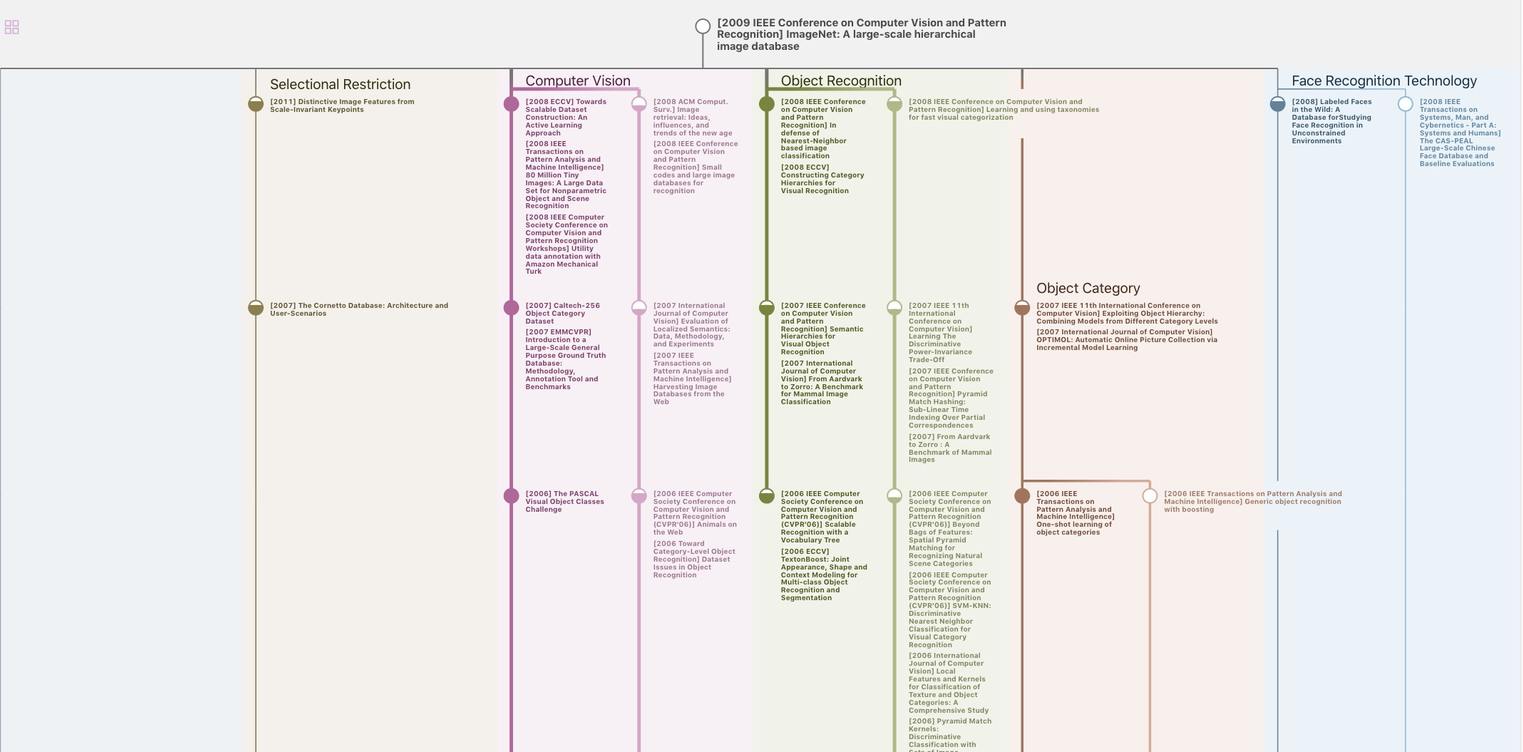
生成溯源树,研究论文发展脉络
Chat Paper
正在生成论文摘要