Trusting my predictions: on the value of Instance-Level analysis
ACM Computing Surveys(2023)
摘要
Machine Learning solutions have spread along many domains, including critical applications. The development of such models usually relies on a dataset containing labeled data. This dataset is then split into training and test sets and the accuracy of the models in replicating the test labels is assessed. This process is often iterated in a cross-validation procedure for obtaining average performance estimates. But is the average of the predictive performance on test sets enough for assessing the trustfulness of a Machine Learning model? This paper discusses the importance of knowing which individual observations of a dataset are more challenging than others and how this characteristic can be measured and used in order to improve classification performance and trustfulness. A set of strategies for measuring the hardness level of the instances of a dataset is surveyed and a Python package containing their implementation is provided.
更多查看译文
关键词
predictions,analysis,instance-level
AI 理解论文
溯源树
样例
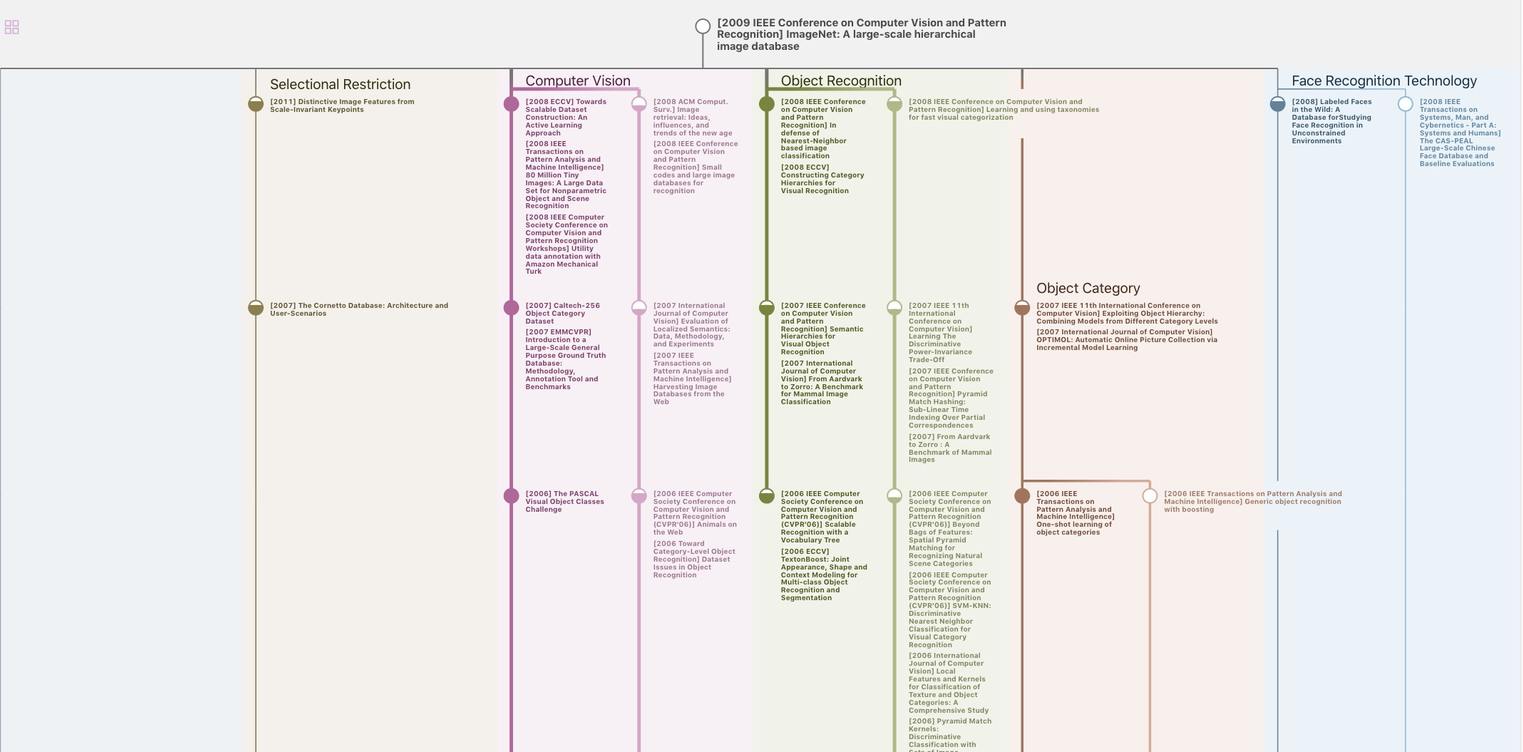
生成溯源树,研究论文发展脉络
Chat Paper
正在生成论文摘要