How to Build Low-cost Networks for Large Language Models (without Sacrificing Performance)?
arXiv (Cornell University)(2023)
摘要
This paper challenges the well-established paradigm for building any-to-any networks for training Large Language Models (LLMs). We show that LLMs exhibit a unique communication pattern where only small groups of GPUs require high-bandwidth communication to achieve near-optimal training performance. Across these groups of GPUs, the communication is insignificant and homogeneous. We propose a new network architecture that resembles the communication requirement of LLMs. Our architecture partitions the cluster into sets of GPUs interconnected with non-blocking any-to-any high-bandwidth interconnects that we call HB domains. Across the HB domains, the network only connects GPUs with non-zero communication demands. We develop an analytical formulation of the training iteration time to evaluate our proposal. Our formulation closely estimates the hardware floating-point utilization within 0.15\% from the ground truth established in prior studies for larger models. We show that our proposed architecture reduces the network cost by 37% to 75% compared to the state-of-the-art any-to-any Clos networks without compromising the performance of LLM training.
更多查看译文
关键词
large language model training,network architectures
AI 理解论文
溯源树
样例
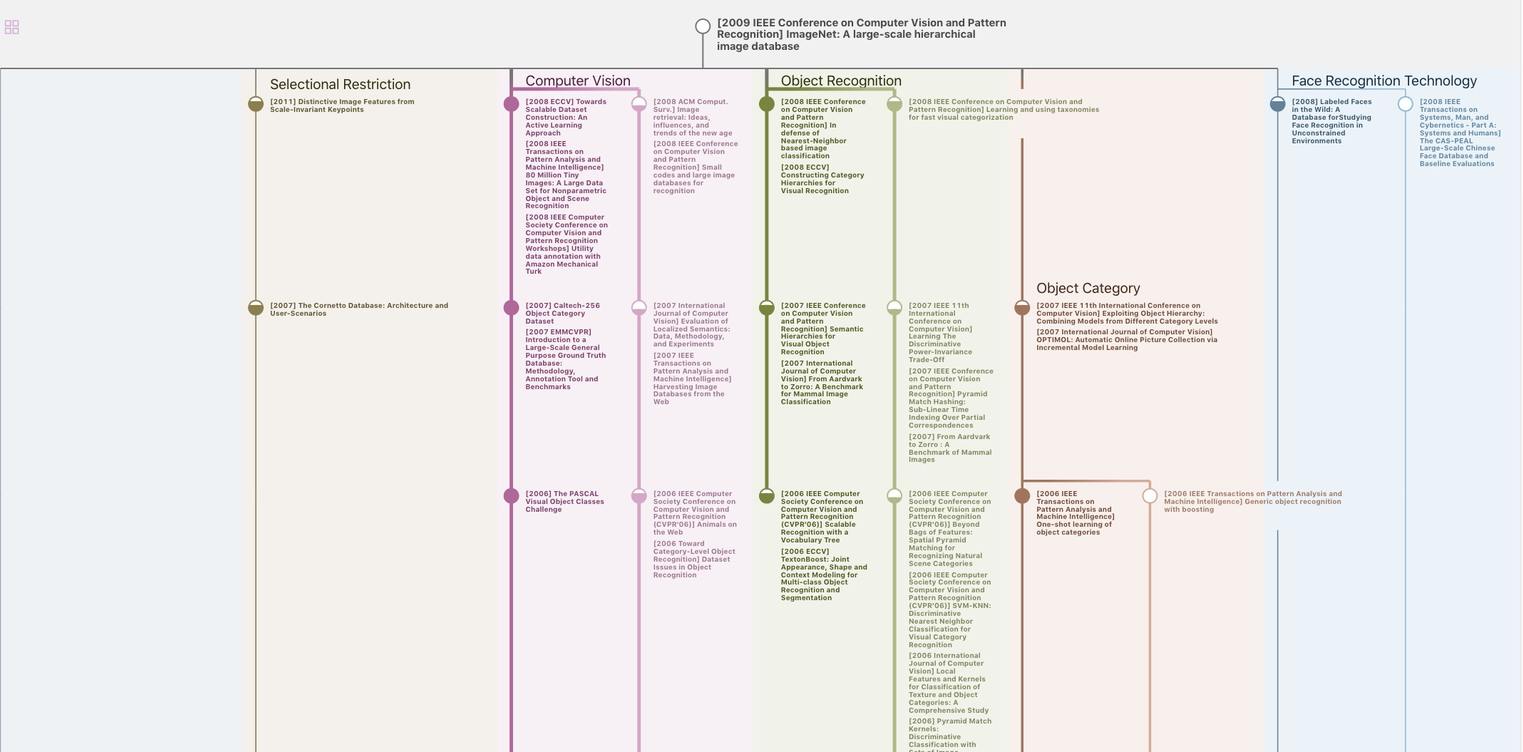
生成溯源树,研究论文发展脉络
Chat Paper
正在生成论文摘要