A Survey of What to Share in Federated Learning: Perspectives on Model Utility, Privacy Leakage, and Communication Efficiency
arXiv (Cornell University)(2023)
摘要
Federated learning (FL) has emerged as a highly effective paradigm for privacy-preserving collaborative training among different parties. Unlike traditional centralized learning, which requires collecting data from each party, FL allows clients to share privacy-preserving information without exposing private datasets. This approach not only guarantees enhanced privacy protection but also facilitates more efficient and secure collaboration among multiple participants. Therefore, FL has gained considerable attention from researchers, promoting numerous surveys to summarize the related works. However, the majority of these surveys concentrate on methods sharing model parameters during the training process, while overlooking the potential of sharing other forms of local information. In this paper, we present a systematic survey from a new perspective, i.e., what to share in FL, with an emphasis on the model utility, privacy leakage, and communication efficiency. This survey differs from previous ones due to four distinct contributions. First, we present a new taxonomy of FL methods in terms of the sharing methods, which includes three categories of shared information: model sharing, synthetic data sharing, and knowledge sharing. Second, we analyze the vulnerability of different sharing methods to privacy attacks and review the defense mechanisms that provide certain privacy guarantees. Third, we conduct extensive experiments to compare the performance and communication overhead of various sharing methods in FL. Besides, we assess the potential privacy leakage through model inversion and membership inference attacks, while comparing the effectiveness of various defense approaches. Finally, we discuss potential deficiencies in current methods and outline future directions for improvement.
更多查看译文
关键词
federated learning,privacy leakage,communication
AI 理解论文
溯源树
样例
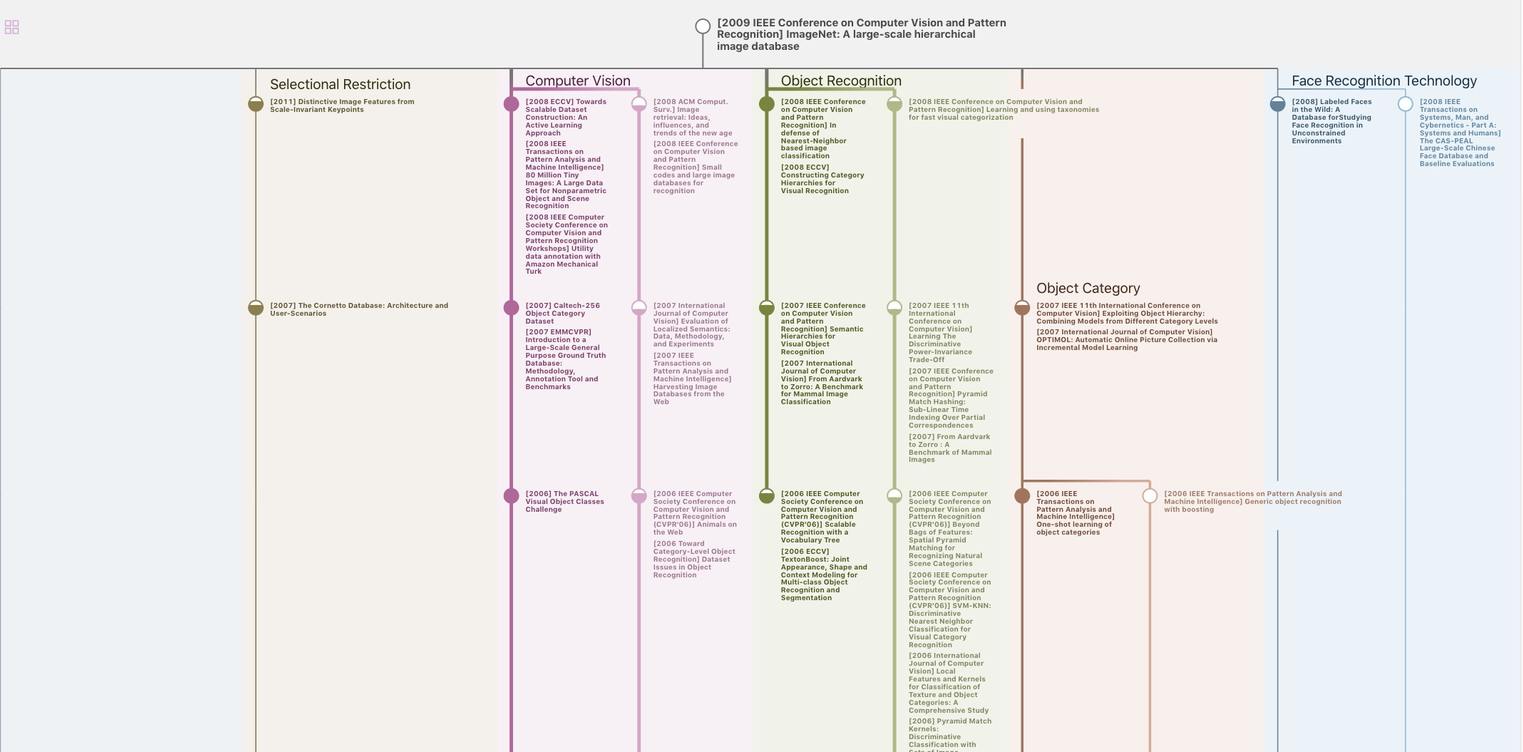
生成溯源树,研究论文发展脉络
Chat Paper
正在生成论文摘要