Can Generative Large Language Models Perform ASR Error Correction?
arXiv (Cornell University)(2023)
摘要
ASR error correction is an interesting option for post processing speech recognition system outputs. These error correction models are usually trained in a supervised fashion using the decoding results of a target ASR system. This approach can be computationally intensive and the model is tuned to a specific ASR system. Recently generative large language models (LLMs) have been applied to a wide range of natural language processing tasks, as they can operate in a zero-shot or few shot fashion. In this paper we investigate using ChatGPT, a generative LLM, for ASR error correction. Based on the ASR N-best output, we propose both unconstrained and constrained, where a member of the N-best list is selected, approaches. Additionally, zero and 1-shot settings are evaluated. Experiments show that this generative LLM approach can yield performance gains for two different state-of-the-art ASR architectures, transducer and attention-encoder-decoder based, and multiple test sets.
更多查看译文
AI 理解论文
溯源树
样例
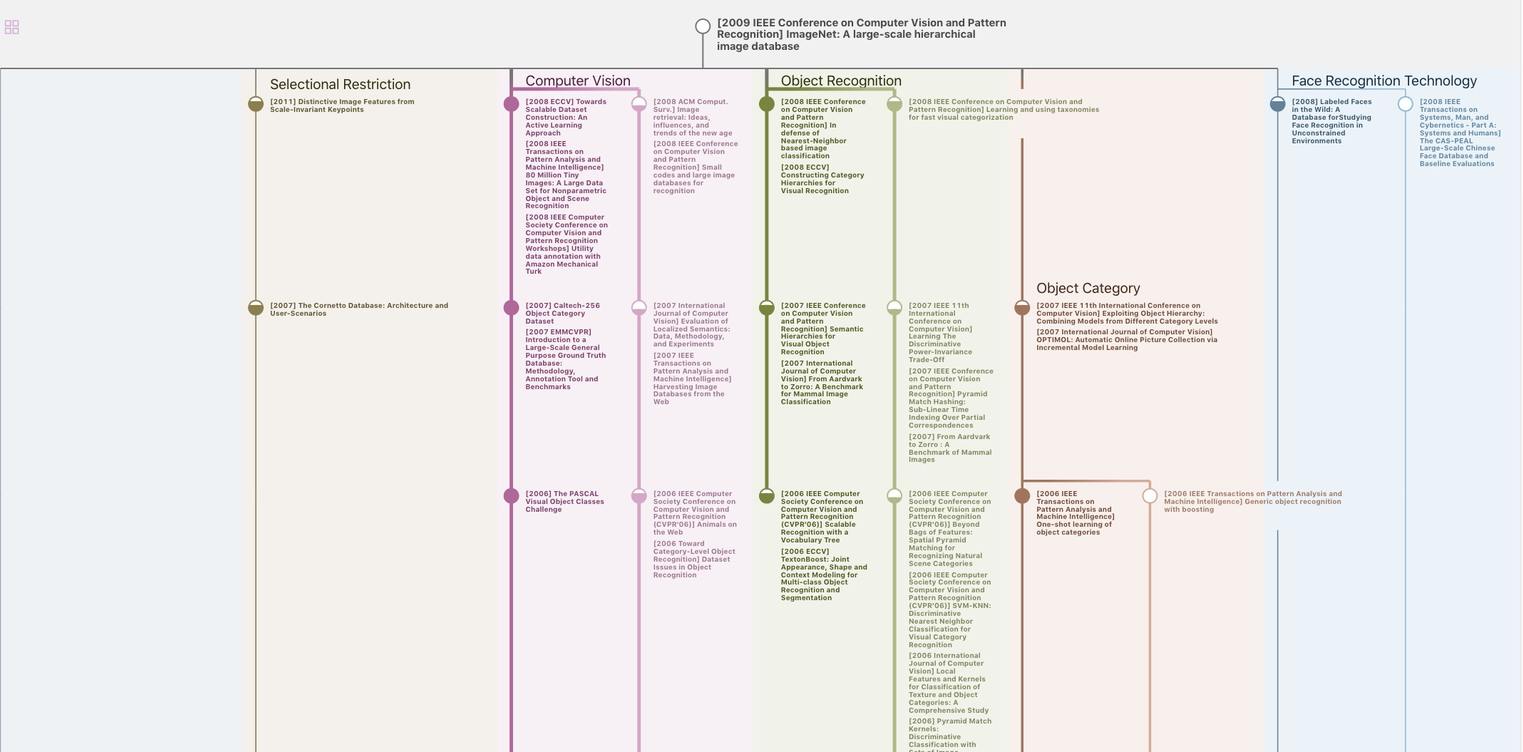
生成溯源树,研究论文发展脉络
Chat Paper
正在生成论文摘要