Human-AI collaboration to identify literature for evidence synthesis
Cell Reports Sustainability(2024)
Abstract
Systematic approaches to evidence synthesis can improve the rigor, transparency, and replicability of a literature review. However, these systematic approaches are resource intensive. We evaluate the ability of ChatGPT to undertake two stages of evidence syntheses (searching peer-reviewed literature and screening for relevance) and develop a collaborative framework to leverage both human and AI intelligence. Using a scoping review of community-based fisheries management as a case study, we find that with substantial prompting, the AI can provide critical insight into the construction and content of a search string. Thereafter, we evaluate five strategies for synthesizing AI output to screen articles based on predefined inclusion criteria. We find that low omission rates (<1%) of relevant literature by the AI are achievable, which is comparable to human screeners. These findings suggest that generalized AI tools can assist reviewers to accelerate the implementation and improve the reliability of literature reviews, thus supporting evidence-informed decision-making.
MoreTranslated text
Key words
artificial intelligence,systematic review,large language models,scientific publication,natural-language,processing,evidence synthesis,ChatGPT,collaborative intelligence
AI Read Science
Must-Reading Tree
Example
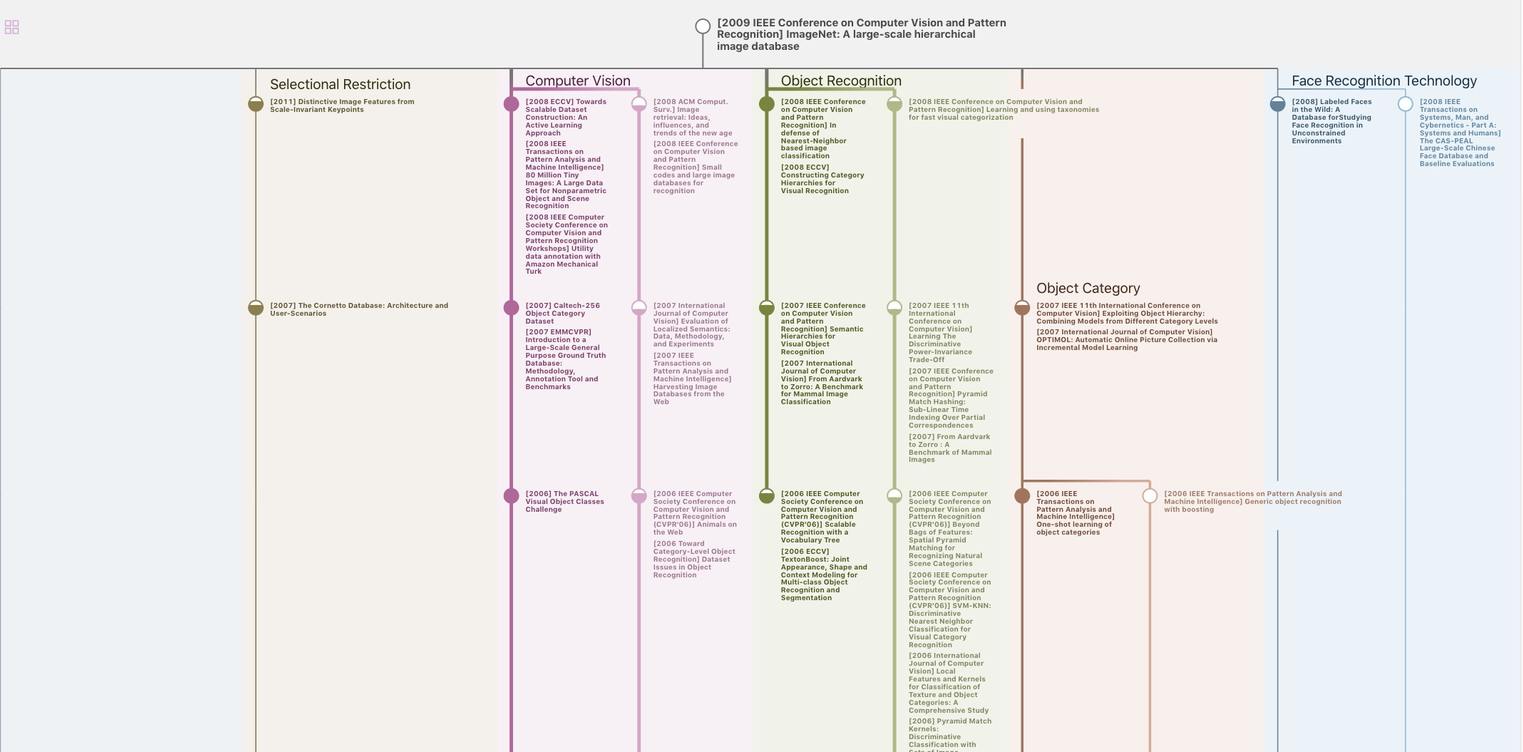
Generate MRT to find the research sequence of this paper
Chat Paper
Summary is being generated by the instructions you defined