Discrimination of Wheat Varieties by Terahertz Time-domain Spectroscopy and Convolutional Neural Network
Research Square (Research Square)(2023)
摘要
Abstract A feasibility study on variety discrimination was organized for 12 wheat varieties before warehousing by applying the Terahertz time-domain spectroscopy (THz-TDS) technology in combination with convolutional neural network (CNN) considering the time-consuming and complex traditional methods for discrimination of wheat varieties that require great number of pretreatment processes. In the experiment, the original time-domain spectra (TDS) of wheat in the range of 0.1~2.0THz were acquired, and the frequency domain spectra (FDS), the refractive index spectra and the absorption coefficient spectra in the range of 0.1~1.4THz were obtained by removing noise and irrelevant information through Fourier Transform and SNV smoothing pretreatment. The competitive adaptive reweighted sampling (CARS) and Uninformative Variable Elimination (UVE) algorithms were applied to screen the absorption coefficient spectra, which were then trained as the input parameters for building the Support Vector Machine (SVM) model, the Least Square Support Vector Machine (LS-SVM) model and the Back-Propagation Neural Networks (BPNN) model for discrimination. A seven-layer convolutional neutral network (CNN) was designed to train the full-spectrum information matrix, which comprises 3 convolution layers, 2 pooling layers, 1 full connection layer and 1 output layer. In the meantime, the Stochastic Gradient Descent Momentum (SGDM) and Dropout operation were introduced in CNN, facilitating the convergence of model and effectively avoiding the overfitting. As for the CNN built during the experiment, the calibration set accuracy and predication set accuracy reached up to 98.7% and 97.8% respectively, with the wrong discrimination rate of 2.2% only. As for the traditional SVM, BPNN and LS-SVM models, the accuracy rates were 89.6%, 87.2% and 90.6% respectively, while the wrong discrimination rates were 10.4%, 12.2% and 9.4%, respectively. The accuracy of the aforesaid three traditional models is lower than that of CNN built during the experiment. According to the results, THz-TDS can be applied to effectively acquire the relevant information about wheat varieties. It is a reliable and efficient solution to discriminate wheat varieties by applying THz-TDS technology in combination with CNN, bearing important practical significance and positive application prospect.
更多查看译文
关键词
wheat varieties,convolutional neural network,time-domain
AI 理解论文
溯源树
样例
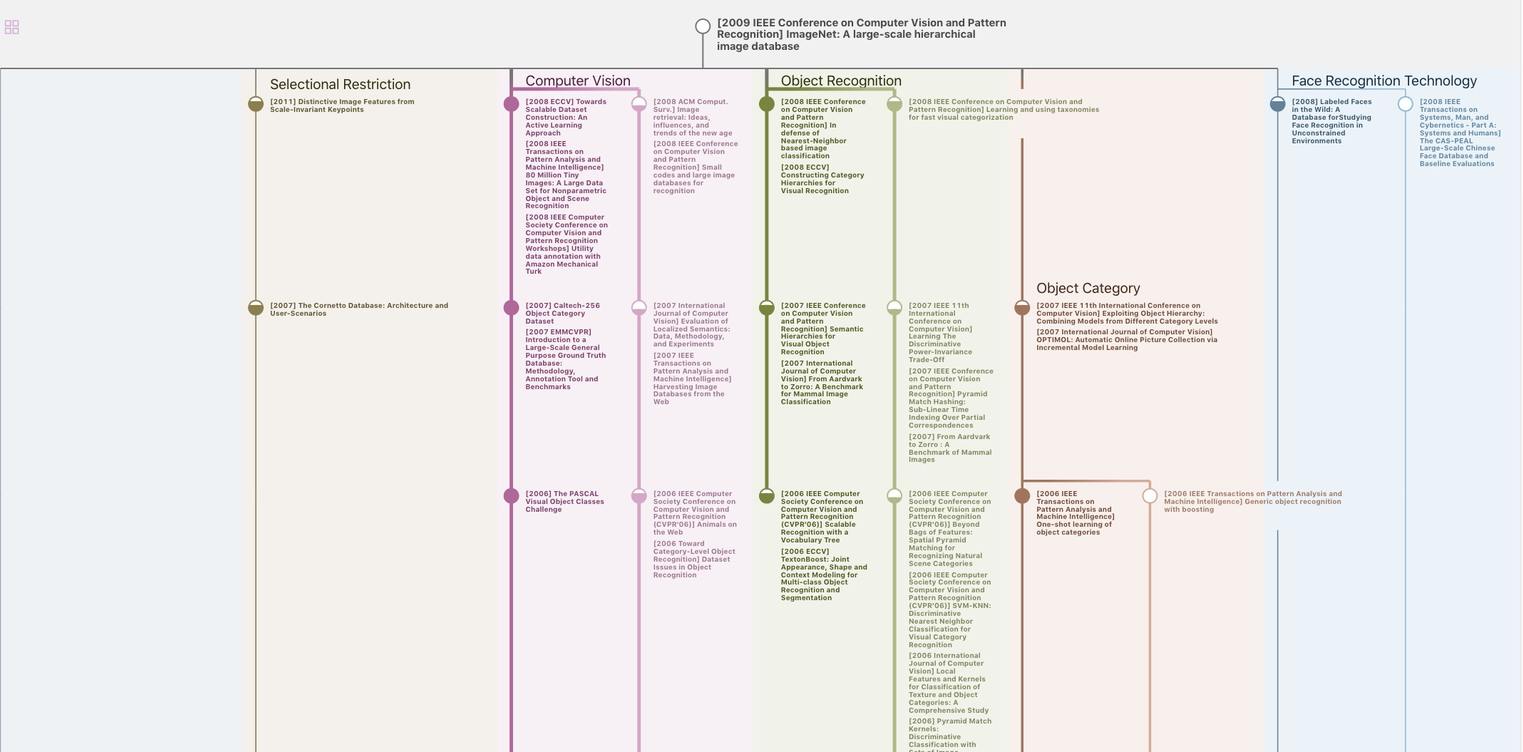
生成溯源树,研究论文发展脉络
Chat Paper
正在生成论文摘要