Two-Stage Grasp Detection Method for Robotics Using Point Clouds and Deep Hierarchical Feature Learning Network
IEEE TRANSACTIONS ON COGNITIVE AND DEVELOPMENTAL SYSTEMS(2024)
摘要
When human beings see different objects, they can quickly make correct grasping strategies through brain decisions. However, grasp, as the first step of most manipulation tasks, is still an open issue in robotics. Although many detection methods have been proposed to take RGB-D images or point clouds as input and output grasp candidates, these methods are still limited to algorithm robustness, such as network performance and graspable objects. In this article, a two-stage grasp detection method is proposed, in which we first use point clouds to train the deep hierarchical feature learning network, which can better capture features of grasped points. We also consider the distribution and discrimination of grasps to construct samples. The score of point clouds is related to the quality of the relevant grasp sample. The quality is given by several grasp metrics applied to the grasp samples obtained from the YCB data set. In the second stage, the network is used to evaluate the grasp candidates sampled from the preprocessed point clouds. The extensive simulation and real-scene experiment show that our grasp detection algorithm achieves satisfactory performance in both single and multiple objects situations. The generalization and scalability of our model also perform well under different conditions.
更多查看译文
关键词
Collaborative robot,grasp detection,point cloud,robotic manipulation
AI 理解论文
溯源树
样例
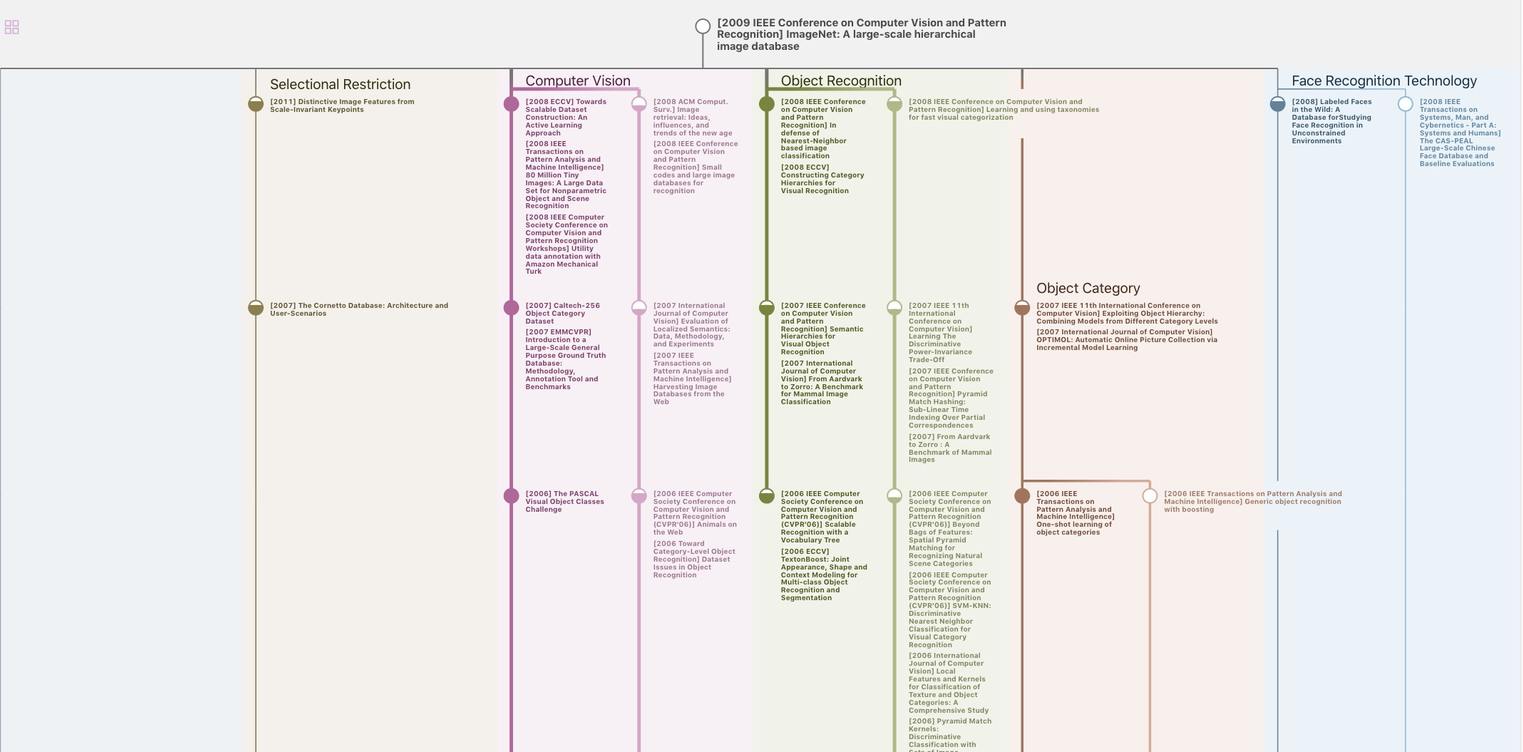
生成溯源树,研究论文发展脉络
Chat Paper
正在生成论文摘要