Characterisation of responder profiles for cardiac resynchronisation therapy based on explainable machine-learning and virtual patient approaches
European Heart Journal - Cardiovascular Imaging(2023)
摘要
Abstract Funding Acknowledgements Type of funding sources: None. Context Cardiac resynchronisation therapy (CRT) is an implant-based therapy applied to patients with a specific heart failure (HF) profile. The characterisation and identification of CRT candidates is challenging since the application of current guidelines still induces a non-responder rate of about 30%. Recently, the assessment of left ventricular mechanics by speckle tracking echocardiography has been shown to provide useful information for patient’s selection. However, the interpretation of stain curves remains difficult due to the great heterogeneities in dyssynchrony patterns. The objective of this study is to propose a characterisation of HF patient profiles considering their responses to CRT, based on unsupervised machine-learning and virtual patient approaches. Methods The prospective database includes 250 patients. Before implantation of CRT, transthoracic echocardiography were performed in order to extract regional myocardial longitudinal strain curves. At 6-month follow-up, response to CRT were defined as 15 % decrease in LV end-systolic volume. Feature extraction was performed from clinical, electrocardiographic, and echocardiographic data, leading to a set of 70 features per patient. The set of all features was clustered by applying the K-Means method. Virtual patients were defined from patient-specific computational models for patients associated with the barycenter of each cluster. The proposed model includes the cardiac electrical system, right and left atria, multi-segment representation of the right and left ventricles, systemic and pulmonary circulations. Contractility, stiffness and electrical activation delays are identified from each virtual patient and each LV cardiac segment. Results Five clusters were identified from clinical, original and classical echocardiographic features with response rates ranging from 50% to 93%. Virtual patients were defined for the 5 patients associated with each cluster barycenter visualised by a principal component analysis. Patient-specific simulated strain curves were provided for the 16 LV segments of these 5 patients. A close match was observed between experimental and simulated curves pattern. The mean RMSE on the five patients is 3.97% ± 1.74. Cluster 1 and 2, which are the two groups with below-average rate, are associated with low contractility of myocardial segments. The other clusters with normal or elevated response rates present higher contractility and delayed electrical activation of the lateral wall. Conclusions A new pipeline, combining personalised cardiovascular modelling and unsupervised machine-learning algorithms, was proposed to provide a phenotyping of HF patient profiles in the context of CRT. This novel approach appears a promising tool to improve understanding of LV mechanics, patient characterisation and selection before CRT.
更多查看译文
关键词
cardiac resynchronisation therapy,machine-learning machine-learning,patient,responder profiles
AI 理解论文
溯源树
样例
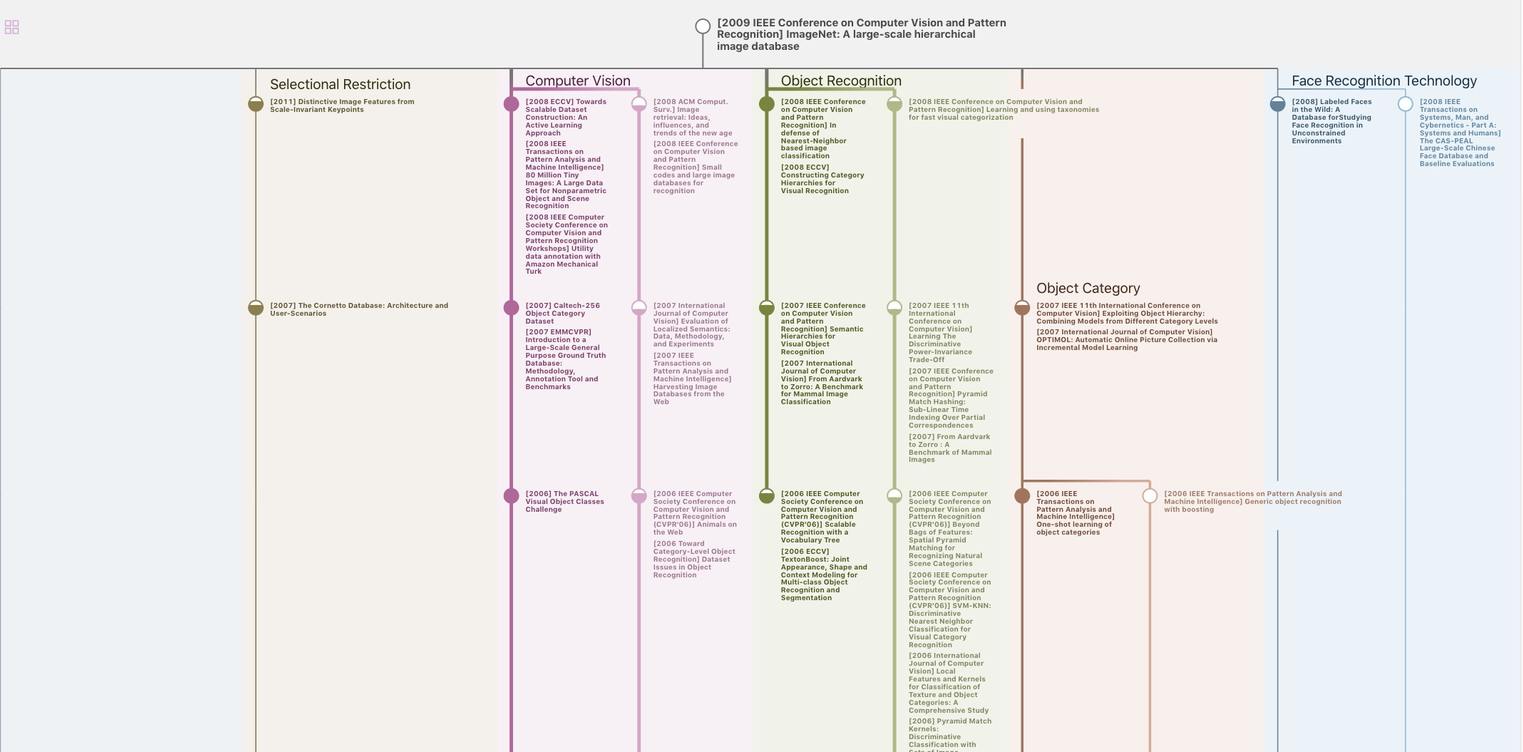
生成溯源树,研究论文发展脉络
Chat Paper
正在生成论文摘要