Detecting Outliers in Cardiopulmonary Exercise Testing Data of Ski Racers - A Comparison of Methods and their Effect on the Performance of Fatigue Prediction.
International journal of computer science in sport(2023)
摘要
Abstract In sports science, cardiopulmonary data is used to assess exercise intensity, performance and health status of athletes and derive relevant target values. However, sensors may produce flawed data and data may include a wide variety of artifacts, which could potentially lead to false conclusions. Thus, appropriate and customized pre-processing algorithms are a vital prerequisite for producing reliable and valid analysis results. To find adequate outlier detection methods for this type of data, we compared three algorithms by applying them on seven ergospirometric measures of junior ski racing athletes and applied a model to predict fatigue during skiing based on the pre-processed data. While values that lie outside a realistic spectrum were consistently labelled as outliers by all methods, and mean values and standard deviations changed in similar ways, methods differed from each other when it comes to changing trends, recurring patterns, and subsequent outliers. Decomposing the sensor data into different components (trend, seasonality, remainder) before dealing with outliers increased average predictive performance the most. However, pre-processing remarkably improved prediction results for certain study participants and not for others. Thus, handling outliers correctly prior to deriving information from ergospirometric data is recommended but more research should be conducted to find methods that achieve more consistent improvement.
更多查看译文
关键词
cardiopulmonary exercise testing data,fatigue prediction,ski racers,outliers
AI 理解论文
溯源树
样例
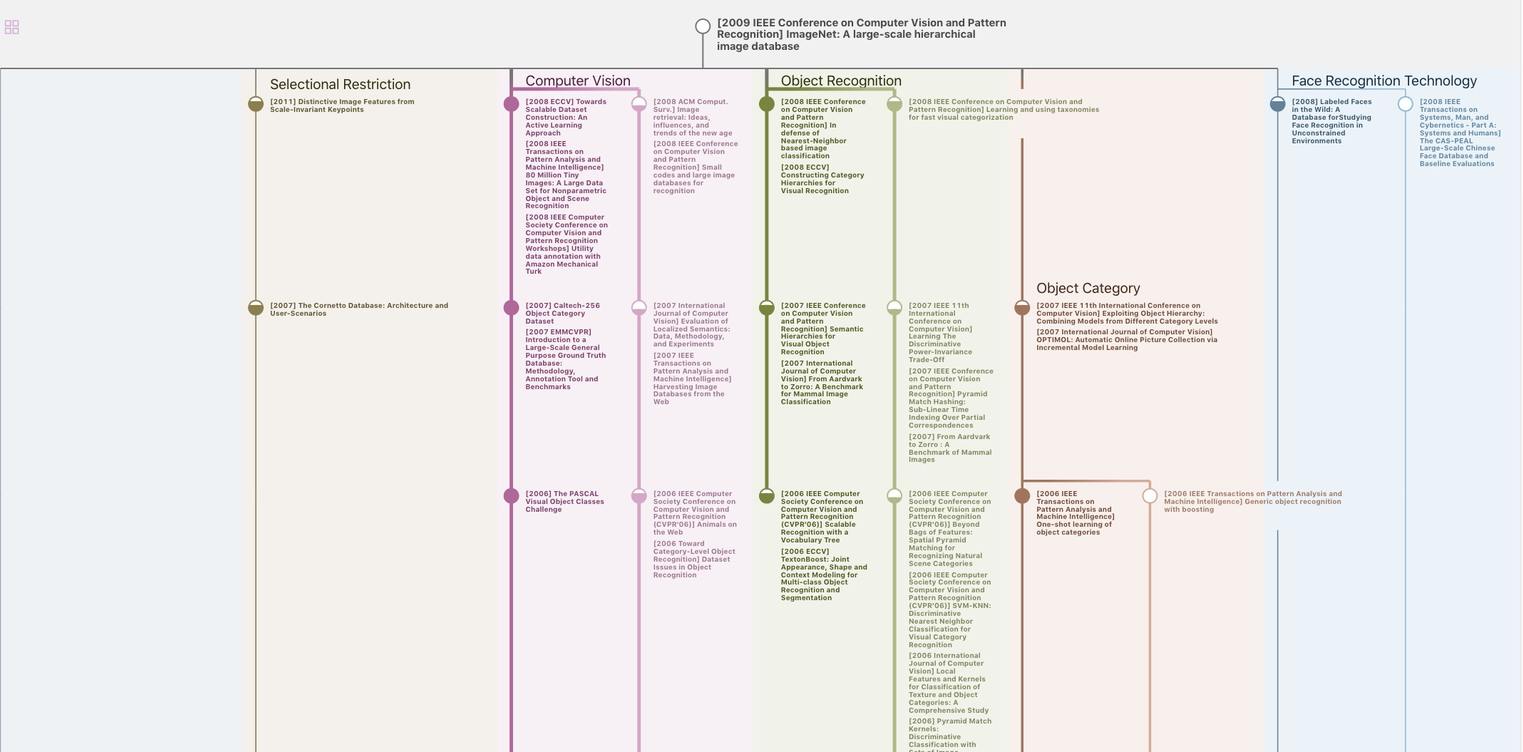
生成溯源树,研究论文发展脉络
Chat Paper
正在生成论文摘要