Robust Optimization of Brine Extraction Well Location for CCS: A Surrogate Model Using FMM and CNN
openalex(2023)
摘要
Summary In geological carbon storage projects, it is essential to manage reservoir pressures for long-term safety. The pressure buildup caused by CO2 injection may lead to serious safety issues, caprock damage, induced seismicity, and leakage. One of the practical solutions for mitigating reservoir pressure buildup is brine extraction. Still, the location of the brine extraction well should be optimized because the performance is affected by the well placement in heterogeneous reservoirs. The optimization of brine extraction well placement is computationally expensive because many simulation runs are required. Robust optimization using multiple reservoir models requires much higher computational costs. Therefore, we propose a machine-learning-based surrogate model that quickly predicts the optimality of brine extraction well. The surrogate model uses a fast-marching method to calculate time-of-flight values that can represent hydraulic connectivity. Then, a convolutional neural network (CNN) predicts the net present value (NPV) by considering the spatial connectivity of a TOF map. When applied to an actual field model, our surrogate model predicted the NPV very well, achieving the averaged determination coefficient value of 0.84. Also, using only 10% of the total location candidates, our surrogate model significantly reduced computational costs.
更多查看译文
关键词
brine extraction well location,robust optimization,ccs
AI 理解论文
溯源树
样例
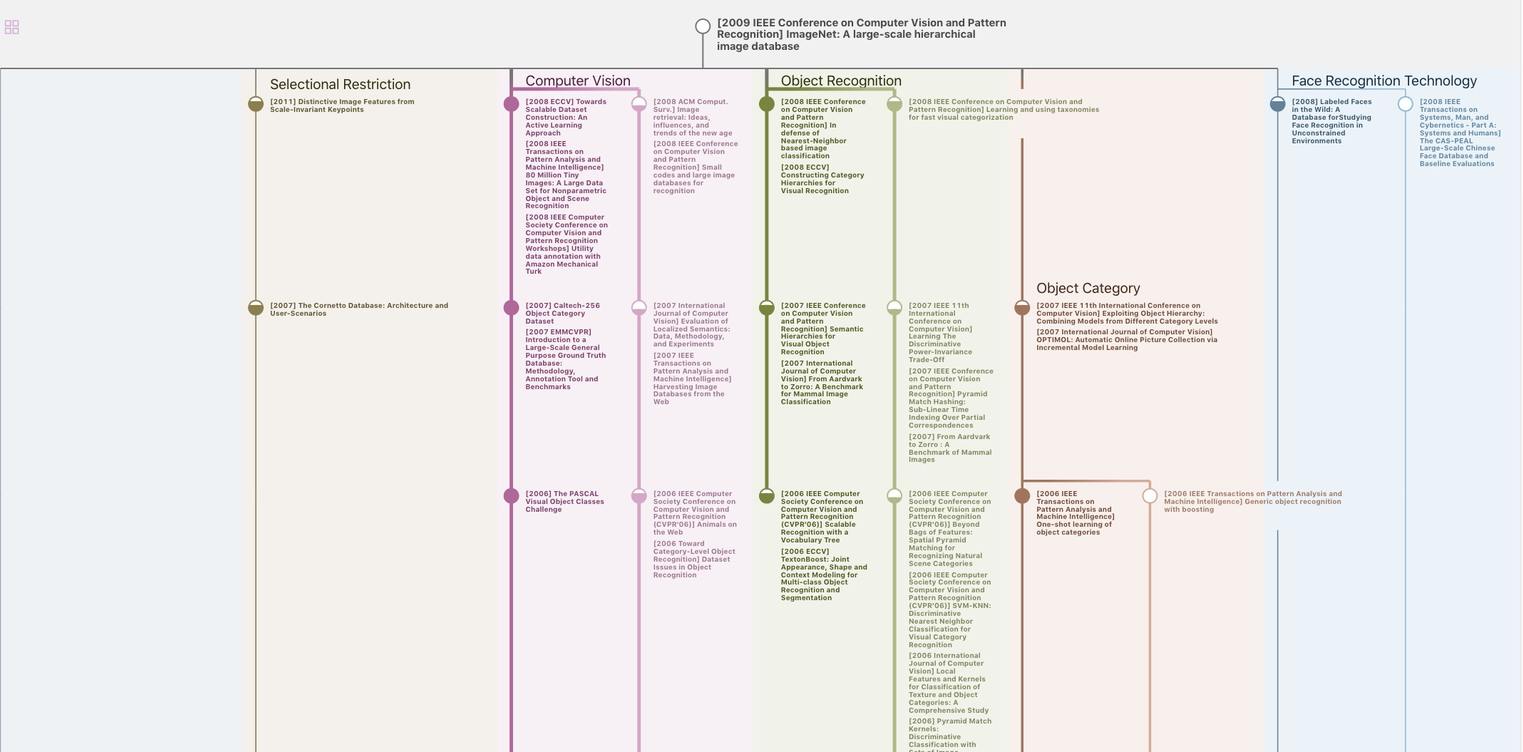
生成溯源树,研究论文发展脉络
Chat Paper
正在生成论文摘要