Neuro or Symbolic? Fine-tuned Transformer with Unsupervised LDA Topic Clustering for Text Sentiment Analysis
IEEE Transactions on Affective Computing(2023)
摘要
For text sentiment analysis, state-of-the-art neural language models have demonstrated promising performance. However, they lack interpretability, require vast volumes of annotated data, and are typically specialized for tasks. In this paper, we explore a connection between fine-tuned Transformer models and unsupervised LDA approach to cope with text sentiment analysis tasks, inspired by the concept of Neuro-symbolic AI. The Transformer and LDA models are combined as a feature extractor to extract the hidden representations of the input text sequences. Subsequently, we employ a feedforward network to forecast various sentiment analysis tasks, such as multi-label emotion prediction, dialogue quality prediction, and nugget detection. Our proposed method obtains the best results in the NTCIR-16 dialogue evaluation (DialEval-2) task, as well as cutting-edge results in emotional intensity prediction using the Ren_CECps corpus. Extensive experiments show that our proposed method is highly explainable, cost-effective in training, and superior in terms of accuracy and robustness.
更多查看译文
关键词
Neuro-symbolic AI,Sentiment Analysis,Fine-tuned Transformer,Latent Dirichlet Allocation
AI 理解论文
溯源树
样例
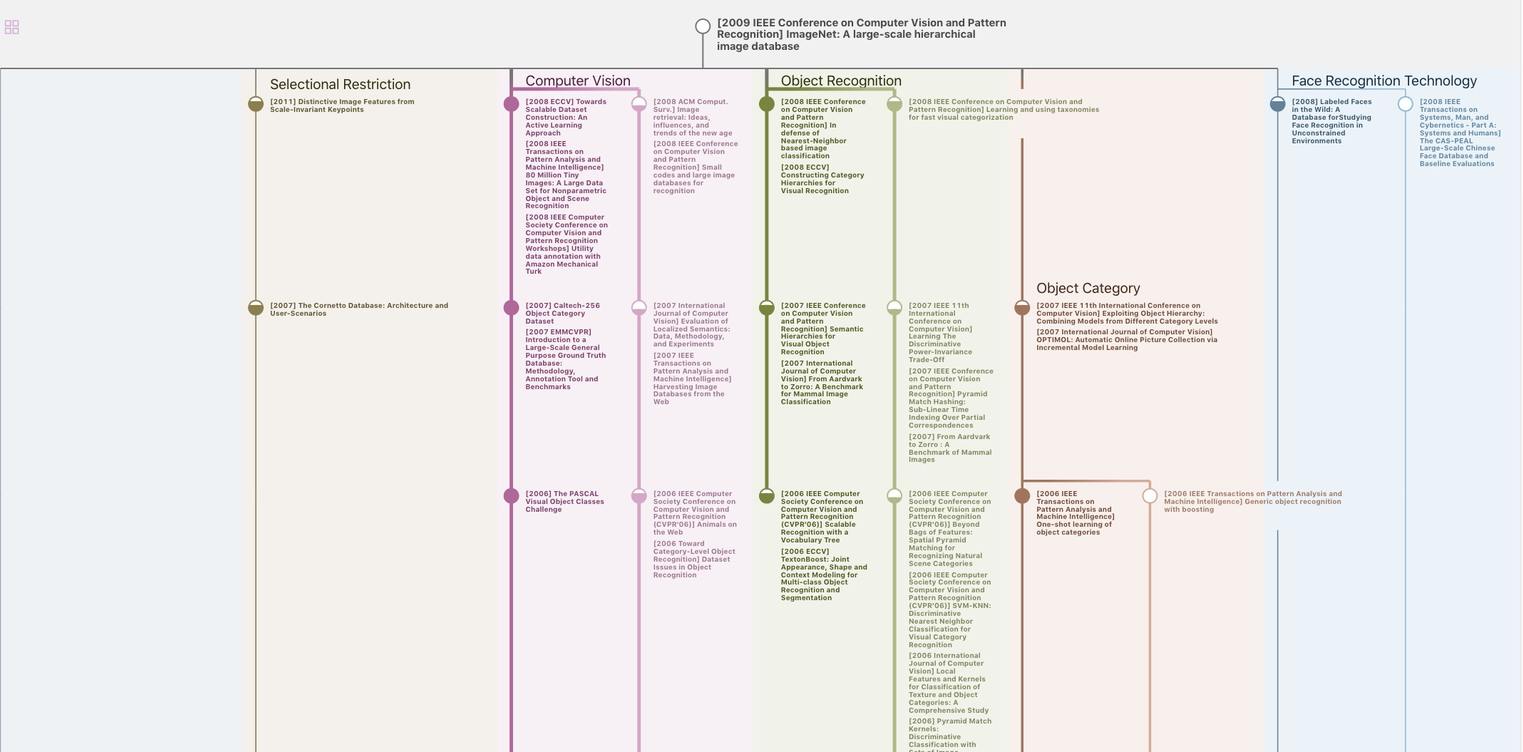
生成溯源树,研究论文发展脉络
Chat Paper
正在生成论文摘要