GeNAS: Neural Architecture Search with Better Generalization
arXiv (Cornell University)(2023)
摘要
Neural Architecture Search (NAS) aims to automatically excavate the optimal network architecture with superior test performance. Recent neural architecture search (NAS) approaches rely on validation loss or accuracy to find the superior network for the target data. In this paper, we investigate a new neural architecture search measure for excavating architectures with better generalization. We demonstrate that the flatness of the loss surface can be a promising proxy for predicting the generalization capability of neural network architectures. We evaluate our proposed method on various search spaces, showing similar or even better performance compared to the state-of-the-art NAS methods. Notably, the resultant architecture found by flatness measure generalizes robustly to various shifts in data distribution (e.g. ImageNet-V2,-A,-O), as well as various tasks such as object detection and semantic segmentation. Code is available at https://github.com/clovaai/GeNAS.
更多查看译文
关键词
neural architecture search,better generalization
AI 理解论文
溯源树
样例
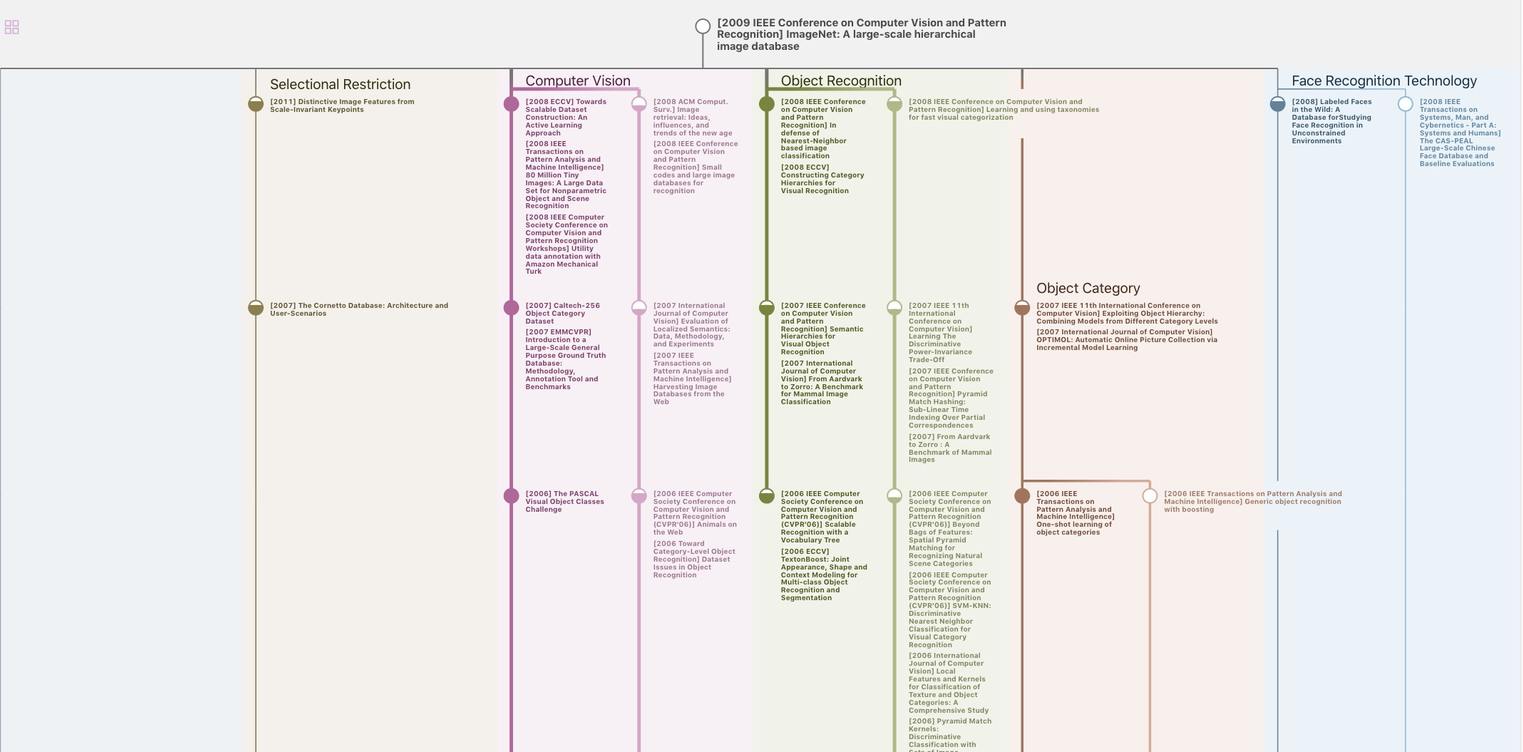
生成溯源树,研究论文发展脉络
Chat Paper
正在生成论文摘要