Uncertainty Estimation using the Local Lipschitz for Deep Learning Image Reconstruction Models
arXiv (Cornell University)(2023)
摘要
The use of supervised deep neural network approaches has been investigated to solve inverse problems in all domains, especially radiology where imaging technologies are at the heart of diagnostics. However, in deployment, these models are exposed to input distributions that are widely shifted from training data, due in part to data biases or drifts. It becomes crucial to know whether a given input lies outside the training data distribution before relying on the reconstruction for diagnosis. The goal of this work is three-fold: (i) demonstrate use of the local Lipshitz value as an uncertainty estimation threshold for determining suitable performance, (ii) provide method for identifying out-of-distribution (OOD) images where the model may not have generalized, and (iii) use the local Lipschitz values to guide proper data augmentation through identifying false positives and decrease epistemic uncertainty. We provide results for both MRI reconstruction and CT sparse view to full view reconstruction using AUTOMAP and UNET architectures due to it being pertinent in the medical domain that reconstructed images remain diagnostically accurate.
更多查看译文
关键词
uncertainty estimation,deep learning image
AI 理解论文
溯源树
样例
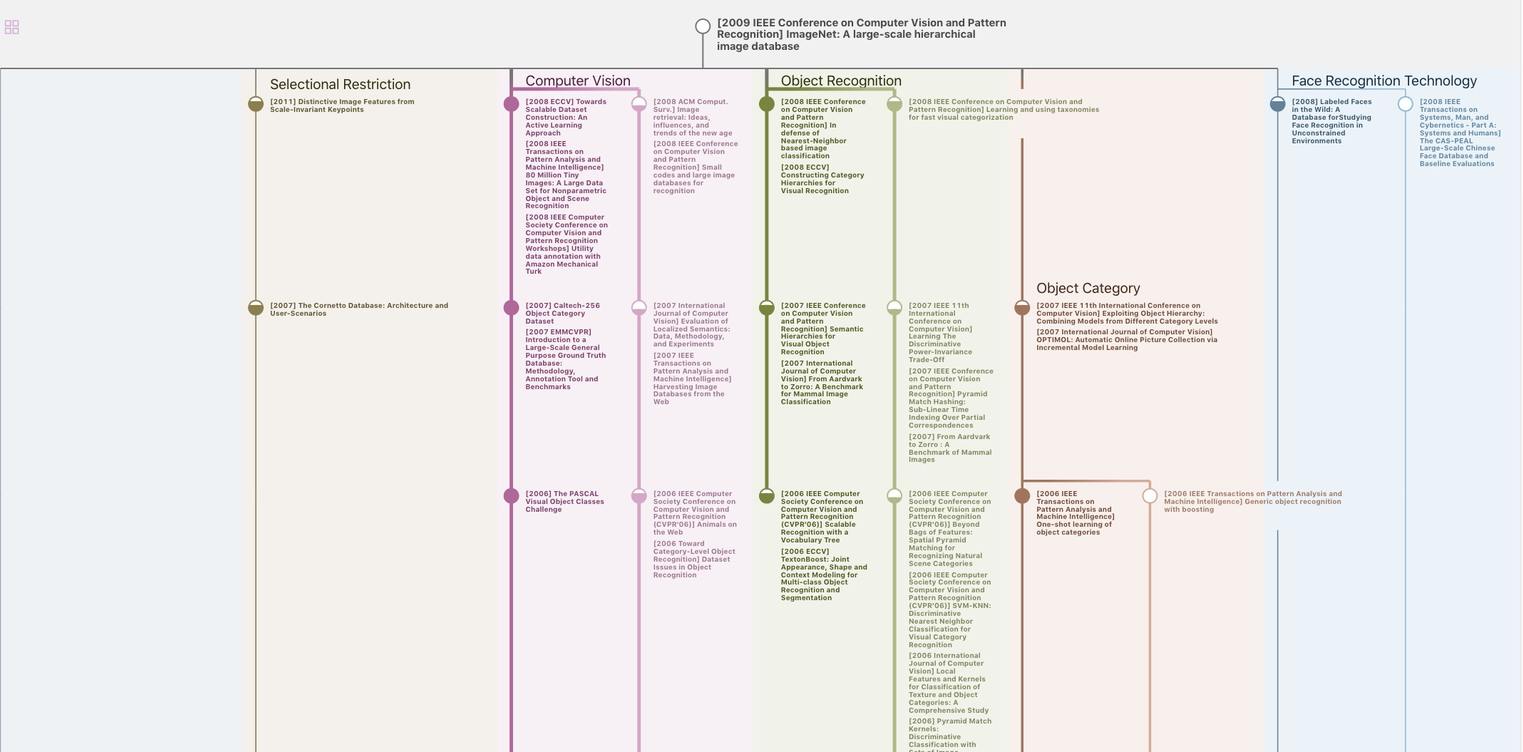
生成溯源树,研究论文发展脉络
Chat Paper
正在生成论文摘要