Machine Learning Identification Of Transcriptomics And Clinical Characteristics Associated With Myocardial Recovery In Heart Failure Patients Undergoing Mechanical Circulatory Support
Journal of Cardiac Failure(2023)
摘要
Background Mechanical unloading and circulatory support with left ventricular assist devices (LVADs) leads to significant structural and functional myocardial improvement in a subset of advanced heart failure (HF) patients, but the interplay between clinical parameters and underlying biological phenomena driving these changes is not well understood. Hypothesis By applying novel machine learning (ML) techniques, we sought to identify transcriptomics and clinical characteristics before the LVAD intervention that associate with subsequent structural and functional cardiac improvement. Methods In a multicenter collaboration, baseline clinical variables and left ventricular apical tissue were collected from HF patients at the time of LVAD implantation (n=208). RNA was isolated from the apical tissue and mRNA transcripts were identified through RNA sequencing. Next, we used ML based on 59 clinical variables and 22,373 mRNA transcripts for the prediction of combined structural and functional myocardial improvement using a cutoff for left ventricular ejection fraction (LVEF) ≥40% and left ventricular end-diastolic diameter (LVEDD) ≤5.9cm. We also independently assessed LVEF and LVEDD as continuous variables representative of functional and structural myocardial improvement respectively, within the year post-LVAD implant. Results Combining the RNA and clinical data resulted in a support vector machine (SVM) model with 80 variables achieving an AUC of 0.73±0.15 for predicting LVAD-mediated myocardial recovery (Figure). Clinical factors associated with myocardial recovery included HF symptoms duration, pre-LVAD LVEF, LVEDD, and HF pharmacologic therapy, as well as LVAD configuration (axial vs. centrifugal). Transcriptomics associated with myocardial recovery included expression of CYREN (DNA repair), ATF3 (cellular stress), DUSP5 (cellular proliferation), and LRRN4C (ligand binding and programmed cell death). Conclusion Using ML, we revealed specific clinical and molecular drivers of LVAD-mediated myocardial recovery. We also identified mechanistic pathways that may have diagnostic, prognostic, and therapeutic implications for the entire spectrum of HF. Mechanical unloading and circulatory support with left ventricular assist devices (LVADs) leads to significant structural and functional myocardial improvement in a subset of advanced heart failure (HF) patients, but the interplay between clinical parameters and underlying biological phenomena driving these changes is not well understood. By applying novel machine learning (ML) techniques, we sought to identify transcriptomics and clinical characteristics before the LVAD intervention that associate with subsequent structural and functional cardiac improvement. In a multicenter collaboration, baseline clinical variables and left ventricular apical tissue were collected from HF patients at the time of LVAD implantation (n=208). RNA was isolated from the apical tissue and mRNA transcripts were identified through RNA sequencing. Next, we used ML based on 59 clinical variables and 22,373 mRNA transcripts for the prediction of combined structural and functional myocardial improvement using a cutoff for left ventricular ejection fraction (LVEF) ≥40% and left ventricular end-diastolic diameter (LVEDD) ≤5.9cm. We also independently assessed LVEF and LVEDD as continuous variables representative of functional and structural myocardial improvement respectively, within the year post-LVAD implant. Combining the RNA and clinical data resulted in a support vector machine (SVM) model with 80 variables achieving an AUC of 0.73±0.15 for predicting LVAD-mediated myocardial recovery (Figure). Clinical factors associated with myocardial recovery included HF symptoms duration, pre-LVAD LVEF, LVEDD, and HF pharmacologic therapy, as well as LVAD configuration (axial vs. centrifugal). Transcriptomics associated with myocardial recovery included expression of CYREN (DNA repair), ATF3 (cellular stress), DUSP5 (cellular proliferation), and LRRN4C (ligand binding and programmed cell death). Using ML, we revealed specific clinical and molecular drivers of LVAD-mediated myocardial recovery. We also identified mechanistic pathways that may have diagnostic, prognostic, and therapeutic implications for the entire spectrum of HF.
更多查看译文
关键词
heart failure patients,transcriptomics,myocardial recovery,machine learning
AI 理解论文
溯源树
样例
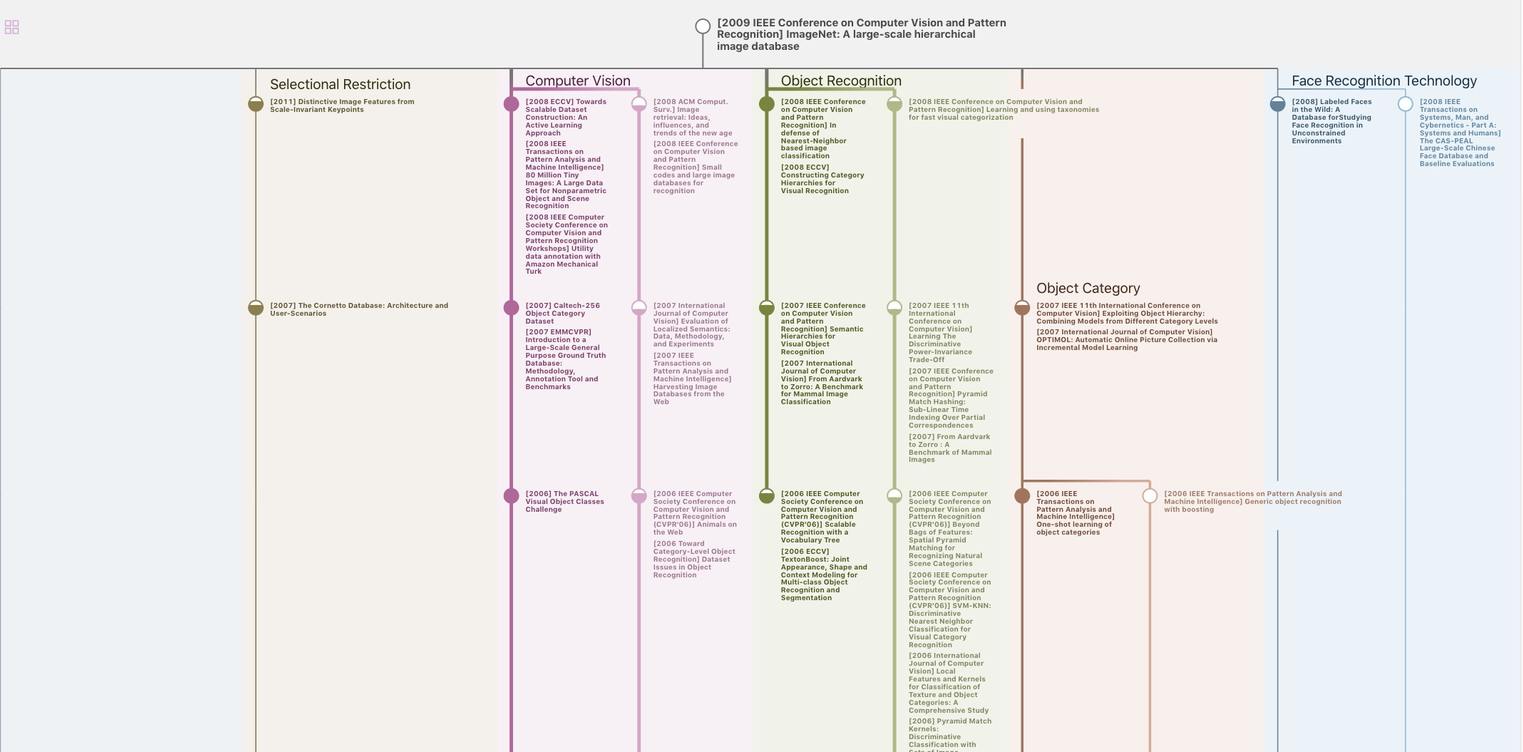
生成溯源树,研究论文发展脉络
Chat Paper
正在生成论文摘要