Abstract 4292: Gene model correction for PVRIG and TIGIT in single cell sequencing data enables accurate detection and study of its functional relevance
Cancer Research(2023)
摘要
Abstract Single cell RNA sequencing (scRNA-seq) has gained increased popularity in recent years and has revolutionized the study of cell populations; however, this technology presents several caveats regarding specific gene expression measurement. Here we examine the expression levels of several immune checkpoint genes, which are currently assessed in clinical studies. We find that unlike in most bulk sequencing studies, PVRIG and murine TIGIT suffers from poor detection in 10x Chromium scRNA-seq and other types of assays that utilize the GENCODE gene model. We show that the default GENCODE gene model, typically used in the analysis of such data, is incorrect in the PVRIG genomic region and contains also a predicted read-through transcript to which PVRIG reads co-align, causing these to be discarded and hence hindering its proper detection. Moreover, we find that the murine TIGIT 3’ UTR is mis-annotated, leading to the loss of legitimate reads. We thus generated a corrected reference genome, by removing the faulty read-through in the case of PVRIG and by extending the TIGIT 3’ UTR and demonstrate that by employing these changes we can correctly capture genuine expression levels of these checkpoints, and which align with our findings at the protein level using FACS and CITEseq. Furthermore, we show that specialized read multimap algorithms such as RSEM and STARsolo can also partially improve the detection of PVRIG. Our study provides means to better interrogate the expression of PVRIG and murine TIGIT in scRNA-seq and emphasize the importance of optimizing gene models and alignment algorithms to enable accurate gene expression measurement in scRNA-seq and bulk sequencing. Moreover, our results support detailed study of the expression of immune checkpoints in clinical and pre-clinical studies towards the development of cancer immunotherapy treatments. Citation Format: Sergey Nemzer, Niv Sabath, Assaf Wool, Zoya Altber, Hirofumi Ando, Drew M. Pardoll, Sudipto Ganguly, Yaron Turpaz, Zurit Levine, Roy Z. Granit. Gene model correction for PVRIG and TIGIT in single cell sequencing data enables accurate detection and study of its functional relevance. [abstract]. In: Proceedings of the American Association for Cancer Research Annual Meeting 2023; Part 1 (Regular and Invited Abstracts); 2023 Apr 14-19; Orlando, FL. Philadelphia (PA): AACR; Cancer Res 2023;83(7_Suppl):Abstract nr 4292.
更多查看译文
关键词
gene model correction,cell sequencing,pvrig,single cell
AI 理解论文
溯源树
样例
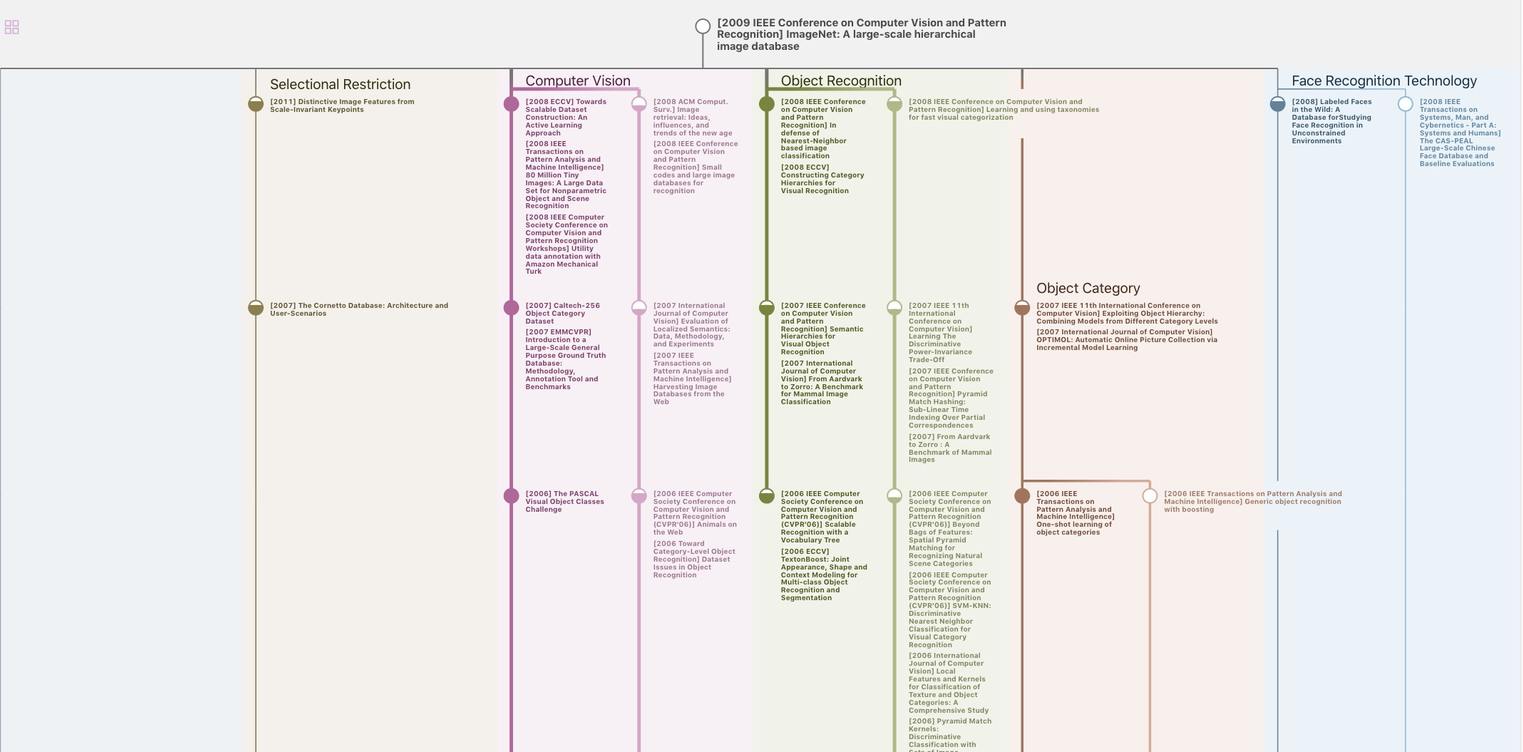
生成溯源树,研究论文发展脉络
Chat Paper
正在生成论文摘要