Prediction of Treatment Failure in Hodgkin Lymphoma: A Machine Learning Radiomic Approach in Baseline 18F-FDG PET/CT
Revista Brasileira de Física Médica(2023)
摘要
Purpose: Hodgkin Lymphoma staging and prognostic evaluation through radiomic and machine learning (ML) methods has been little explored. This study explores radiomic features and proposes a new ML radiomic model to predict treatment failure in Hodgkin lymphoma patients from PET images. Methods: 13 radiomic features were extracted from PET images of 51 subjects with Hodgkin’s Lymphoma with response to therapy and 6 subjects with treatment failure as classified by the Lugano criteria for lymphoma response. Univariate analysis was performed for feature selection by means of the areas under ROC curves (AUC), and Pearson correlation was assessed to reduce redundancy in the feature space. A ML algorithm was developed and trained-validated with a six-fold stratified cross validation. The ML performance was assessed through the results of 15 combinations of two-fold validation samples, deriving two ML methods (M1 and M2) for tumor classification at the tumor and patient levels. Results: The features zone percentage (ZP), high intensity large area emphasis (HILAE), entropy and standardized uptake value of maximum pixel (SUVmax) were independent predictors of treatment failure. The ML algorithm performed with AUC=0.86 (M1) and AUC=0.96 (M2) to classify “individual tumors” and at “patient level” performed with sensitivity = 80.0% /specificity = 88.3% (M1) and sensitivity = 100% /specificity = 100% (M2). The ML outperformed the Ann Arbor staging that achieved, respectively, 83.3% and 31.7% for sensitivity and specificity. Conclusions: Evaluation of baseline 18F-FDG PET scans of individuals with Hodgkin’s lymphoma using a ML radiomic model enabled accurate classification of patients at higher risk of treatment failure.
更多查看译文
关键词
hodgkin lymphoma,machine learning radiomic approach,f-fdg
AI 理解论文
溯源树
样例
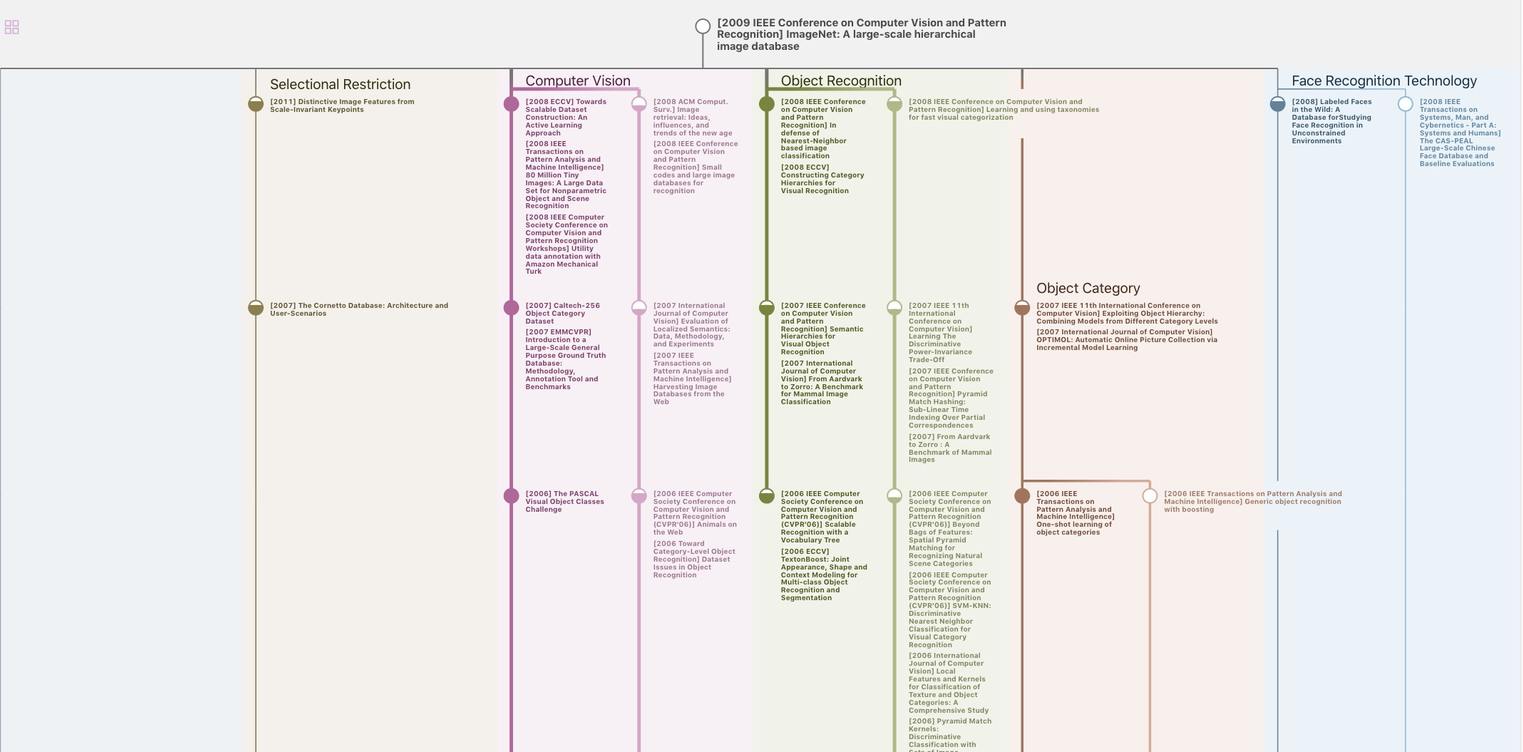
生成溯源树,研究论文发展脉络
Chat Paper
正在生成论文摘要