Establishing a prediction model of severe acute mountain sickness using deep learning of support vector machine recursive feature elimination
Research Square (Research Square)(2023)
摘要
Abstract Background Severe acute mountain sickness (sAMS) can be life-threatening, but little is known about its genetic basis. Using microarray genotype data and phenotype data for deep learning, we aimed to explore the genetic susceptibility of sAMS for the purpose of prediction. Methods The study was based on microarray data from 112 peripheral blood mononuclear cell (PBMC) samples of 21 subjects, who were exposed to very high altitude (5260 m), low barometric pressure (406 mmHg), and hypobaric hypoxia (VLH) at various timepoints. Subjects were investigated for the interplay effects between multiple phenotypic risk factors, and the underlying risk genes were identified to establish the prediction model of sAMS using the support vector machine recursive feature elimination (SVM-RFE) method. Results Exposure to VLH activated the gene expression in leukocytes, resulting in inverted CD4/CD8 ratio which interplayed with other phenotypic risk factors at the genetic level (P < 0.001). 2291 underlying risk genes were input to SVM-RFE system for deep learning, and a prediction model was established with satisfactory predictive accuracy (C-index = 1), and clinical applicability for sAMS using ten featured genes with significant predictive power (P < 0.05). Five featured genes ( EPHB3 , DIP2B , RHEBL1 , GALNT13 , and SLC8A2 ) were identified as the upstream of hypoxia and/ or inflammation-related pathways mediated by micorRNAs as potential biomarkers for sAMS. Conclusions The established prediction model of sAMS holds promise to be clinically applied as a genetic screening tool for sAMS. More studies are needed to establish the role of the featured genes as biomarker for sAMS.
更多查看译文
关键词
severe acute mountain sickness,support vector machine,deep learning
AI 理解论文
溯源树
样例
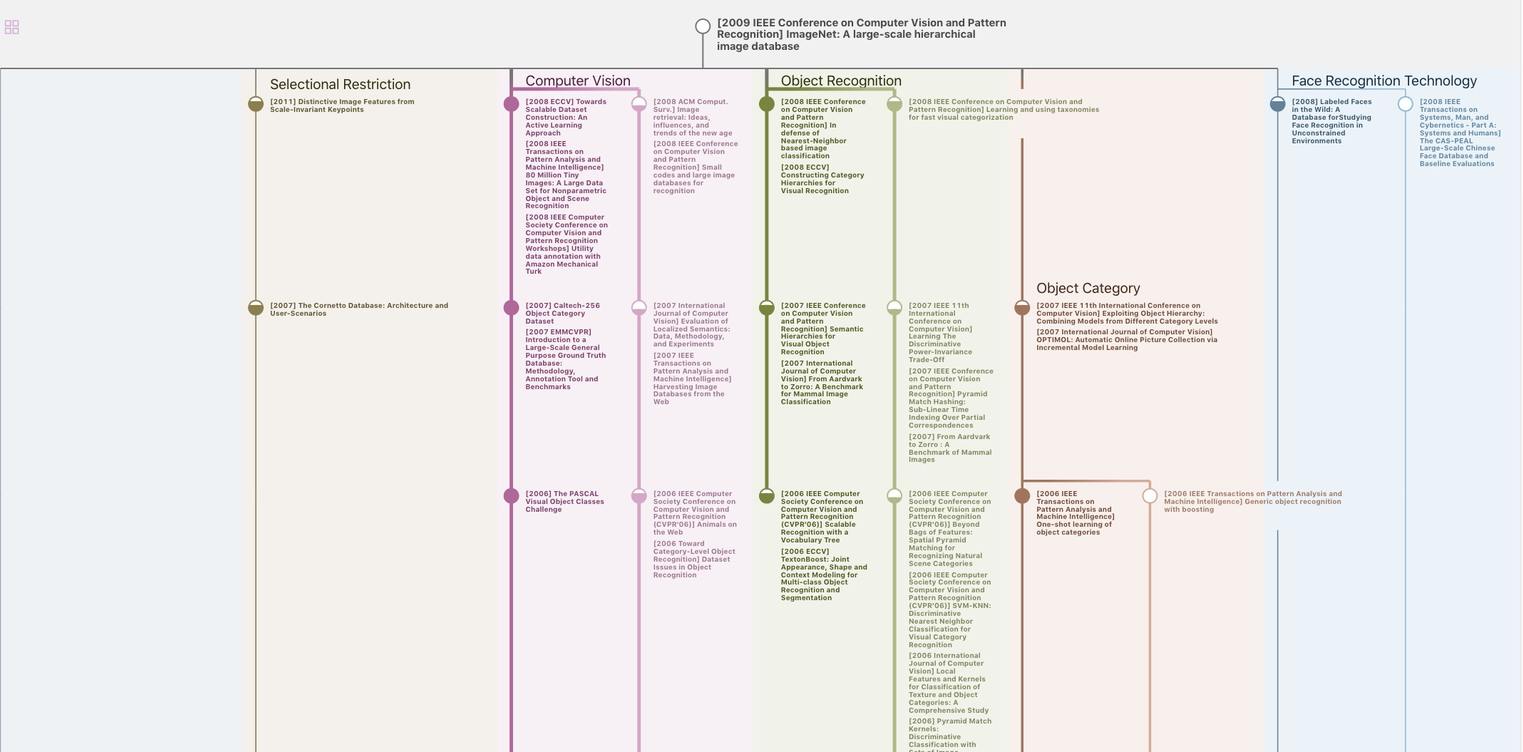
生成溯源树,研究论文发展脉络
Chat Paper
正在生成论文摘要