Development of a machine learning model to identify elbow implants from radiographic images
Seminars in Arthroplasty: JSES(2023)
摘要
Total elbow arthroplasty rates continue to rise year after year with up to 7.6% annual growth, yet complication and failure rates have been reported as high as 90%. Proper identification of implants prior to revision is crucial to optimize clinical resources and postoperative outcomes. This study aims to evaluate a machine learning algorithm for quick and accurate identification of elbow implants from radiographic images. A total of 142 elbow implant radiographs representing 5 unique prostheses were obtained from deidentified patients within the Mount Sinai Health System in New York. Images were split into training (n = 97) and test (n = 45) sets. A modified version of the convolutional neural network DenseNet-121 was used to train and evaluate the data. The model was then assessed for accuracy, sensitivity, positive predictive value, and f-1 scores. Overall model accuracy was 97.8% and top-3 accuracy was 100% for each of the 5 prostheses. Sensitivity, positive predictive value, and f-1 scores for the model were 0.978, 0.980, and 0.96, respectively. The algorithm took 14.10 seconds to classify the 45 images in the test set, averaging 0.31 seconds per image. The model demonstrated strong performance in the identification of elbow implants among the sample of 142 images and 5 implant types. While these results suggest promise in providing future clinical utility within surgical workflows, the method requires external validation and further testing with a greater number and wider variety of implants.
更多查看译文
关键词
Basic Science Study,Computer Modeling
AI 理解论文
溯源树
样例
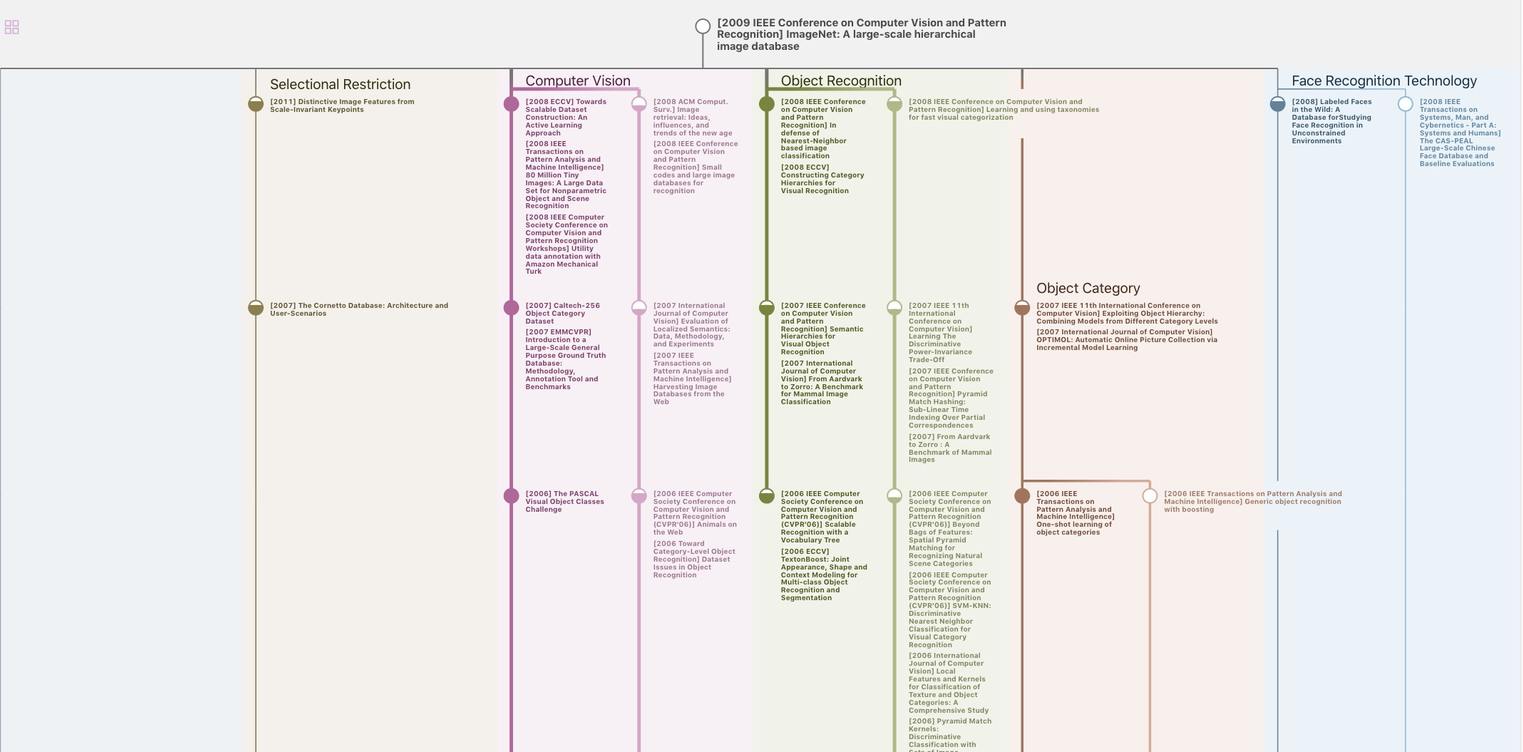
生成溯源树,研究论文发展脉络
Chat Paper
正在生成论文摘要