Deep Neural Networks for Source Detection in Radio Astronomical Maps
Astrophysics and space science proceedings(2023)
摘要
Source finding is one of the most challenging tasks in upcoming radio continuum surveys with SKA precursors, such as the Evolutionary Map of the Universe (EMU) survey of the Australian SKA Pathfinder (ASKAP) telescope. In this work, we explore the application of several deep learning methods, based both on classical CNNs and on more recent transformer architectures to perform object detection. All the models were trained using ASKAP data, taken during the Early Science phase, and previous radio survey data. Results in terms of Precision, Recall, and F1-Score are promising. We evaluate several training strategies and model architectures to determine which one fits the case study best. Finally, we exploited High Performance Computing to run our experiments, to evaluate the improvement of a distributed training process, running on multiple computing nodes, compared to a single-node, single-process approach.
更多查看译文
关键词
deep neural networks,source detection,radio,neural networks,maps
AI 理解论文
溯源树
样例
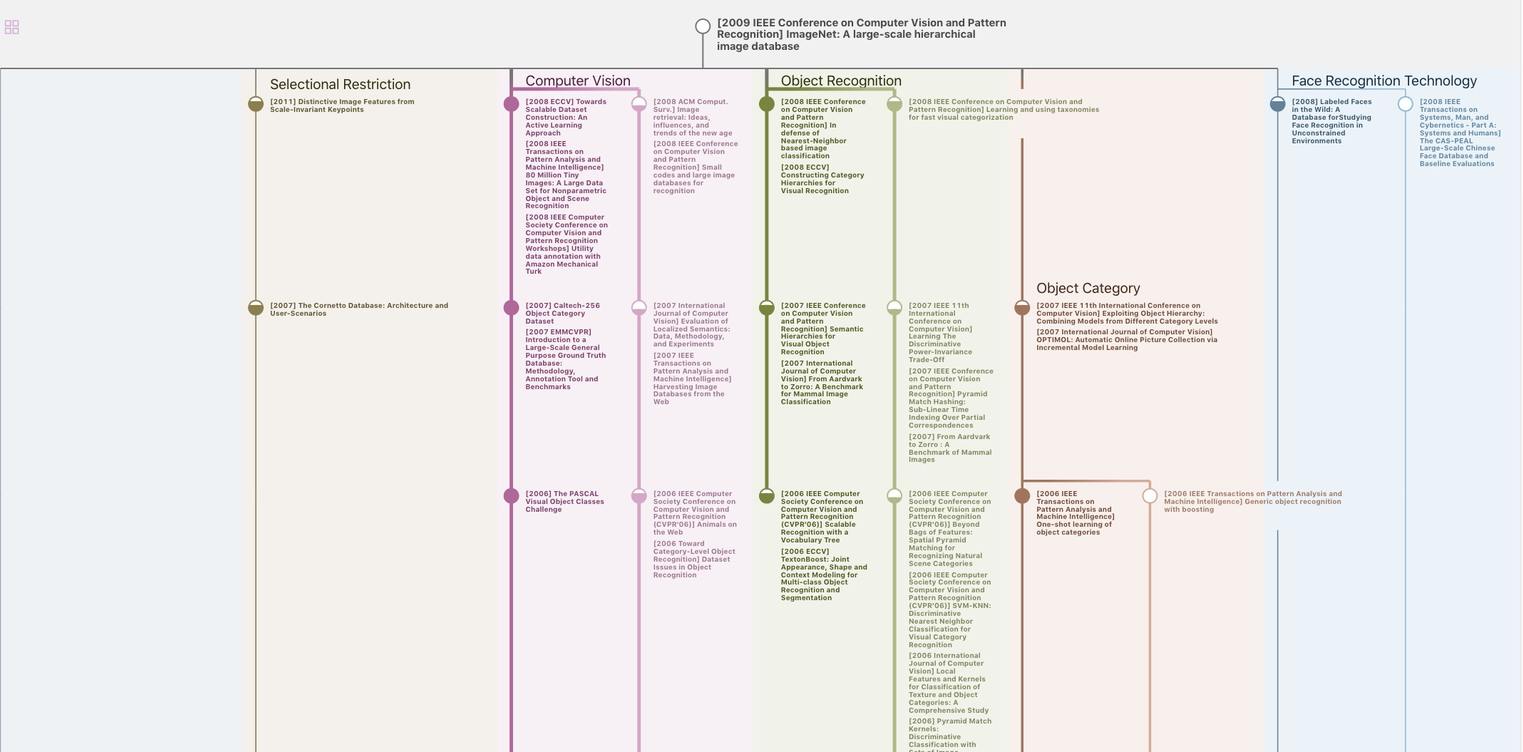
生成溯源树,研究论文发展脉络
Chat Paper
正在生成论文摘要