Ontology-Driven Semantic Analysis of Tabular Data: An Iterative Approach with Advanced Entity Recognition
APPLIED SCIENCES-BASEL(2023)
摘要
This study focuses on the extraction and semantic analysis of data from tables, emphasizing the importance of understanding the semantics of tables to obtain useful information. The main goal was to develop a technology using the ontology for the semantic analysis of tables. An iterative algorithm has been proposed that can parse the contents of a table and determine cell types based on the ontology. The study presents an automated method for extracting data in various languages in various fields, subject to the availability of an appropriate ontology. Advanced techniques such as cosine distance search and table subject classification based on a neural network have been integrated to increase efficiency. The result is a software application capable of semantically classifying tabular data, facilitating the rapid transition of information from tables to ontologies. Rigorous testing, including 30 tables in the field of water resources and socio-economic indicators of Kazakhstan, confirmed the reliability of the algorithm. The results demonstrate high accuracy with a notable triple extraction recall of 99.4%. The use of Levenshtein distance for matching entities and ontology as a source of information was key to achieving these metrics. The study offers a promising tool for efficiently extracting data from tables.
更多查看译文
关键词
semantic analysis,OWL ontology,table interpretation,knowledge triplets,entity classification,Levenshtein distance
AI 理解论文
溯源树
样例
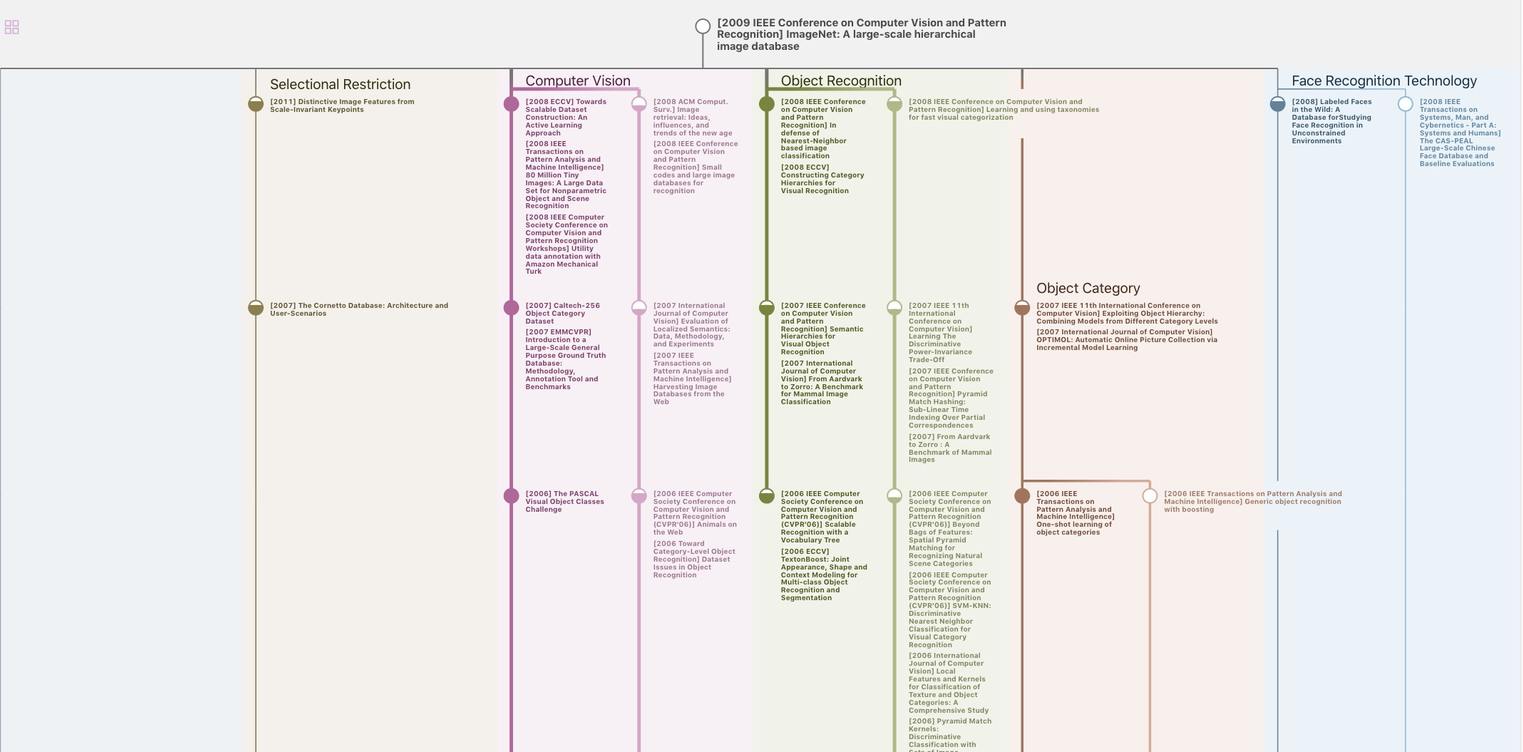
生成溯源树,研究论文发展脉络
Chat Paper
正在生成论文摘要