A High Computationally Efficient Parallel Partial Gaussian Process for Large-Scale Power System Probabilistic Transient Stability Assessment
IEEE Transactions on Power Systems(2023)
摘要
This paper proposes a specifically designed parallel partial Gaussian process (PPGP) for large-scale probabilistic power system transient stability assessment (TSA). The differential and algebraic equations with uncertain resources are defined and reformulated regarding uncertainty quantification. The challenges of probabilistic TSA in large-scale systems are investigated and the necessity of introducing efficient modeling approaches is emphasized. PPGP inherits the advantages of Gaussian process modeling, including data-driven modeling that does not require the knowledge of input distribution and can effectively quantify the confidence interval of the estimate. It accelerates the model construction and evaluation by reducing the number of parameters to be estimated. In addition, thanks to the enhancement techniques via isotropic kernel, reparameterization, and composite likelihood, PPGP is able to achieve probabilistic TSA under high uncertainty conditions while maintaining very high computational efficiency. A theoretical analysis of the time complexity of PPGP is provided. Comparison results with the Latin hypercube sampling method, many single Gaussian processes, and sparse Gaussian process methods on the Texas 2000- bus system with 471 PVs and 470 wind generations highlight the significantly improved model efficacy and efficiency of the proposed method. Furthermore, experimental validation demonstrates the proposed method's efficacy in handling the co-existence of both stable and unstable cases.
更多查看译文
关键词
Uncertainty quantification,probabilistic transient stability assessment,parallel partial Gaussian process,power system dynamics,renewable energy
AI 理解论文
溯源树
样例
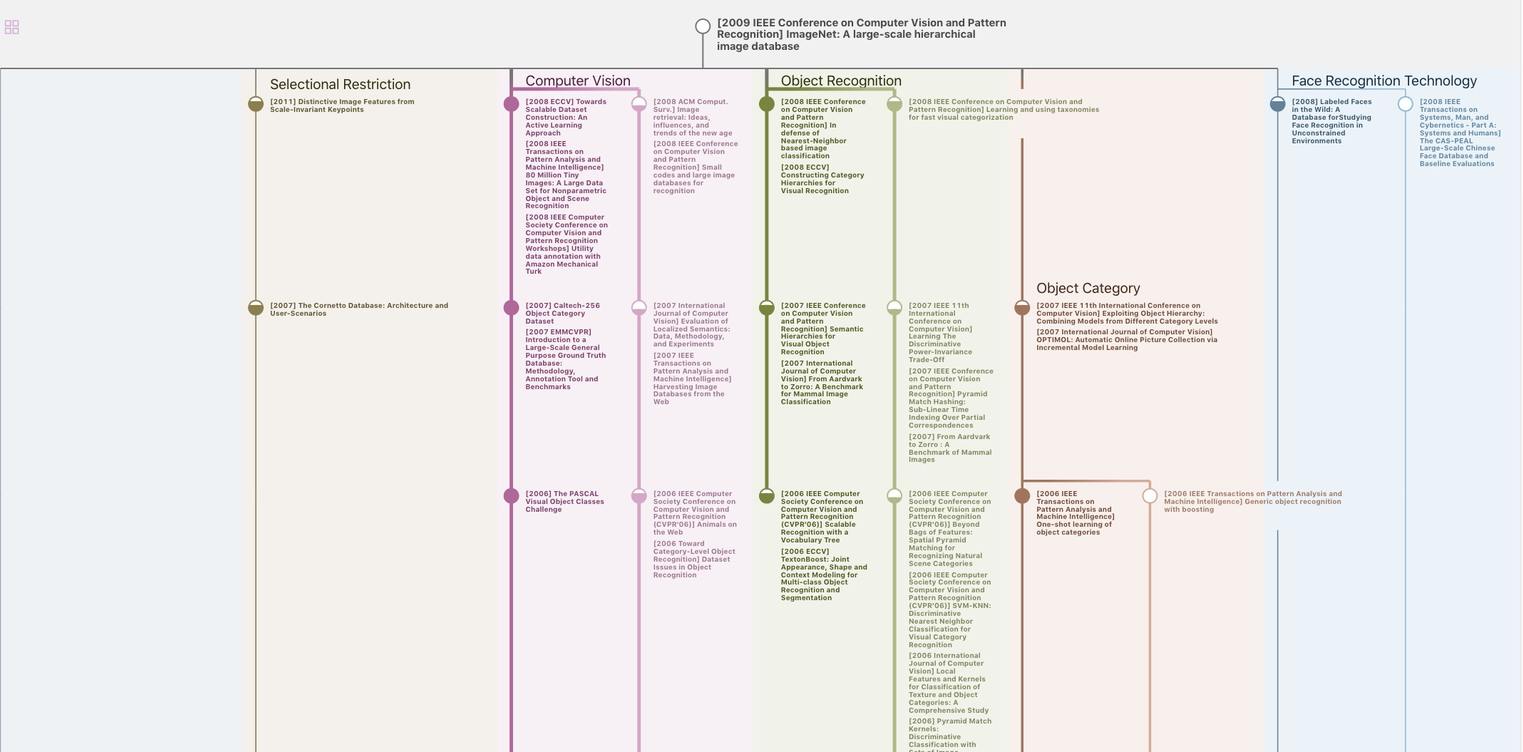
生成溯源树,研究论文发展脉络
Chat Paper
正在生成论文摘要