SemiAMR: Semi-Supervised Automatic Modulation Recognition With Corrected Pseudo-Label and Consistency Regularization
IEEE TRANSACTIONS ON COGNITIVE COMMUNICATIONS AND NETWORKING(2024)
摘要
In modern communication systems, automatic modulation recognition (AMR) has broad application prospects but a large amount of training data is usually required. Due to the high cost of adding annotations to signals, it is of great significance to study AMR under the condition of few labeled samples. In this paper, an end-to-end semi-supervised radio signal recognition algorithm is proposed. In view of the poor anti-noise performance of the original IQ data, short-time Fourier transform (STFT) is introduced to mine the stable modulation characteristics. In order to overcome the noise problem caused by pseudo-labels, a mixed sample data augmentation (MSDA) method PFMix is proposed, and the consistency constraint of the model disturbance based on mean teacher (MT) framework and data disturbance based on PFMix is designed. Meanwhile, in order to provide pseudo-labels with high confidence, entropy regularization is constructed by taking the prediction variance of MT framework as correction factor. The experimental results using the open source RML2016.10A dataset show the superior performance of the proposed method. The average recognition accuracy with 1% labeled data is 88.82% at 0 dB, which is a significant improvement compared with state of the art in semi-supervised AMR.
更多查看译文
关键词
Modulation,Feature extraction,Data models,Training,Task analysis,Entropy,Correlation,Automatic modulation recognition,semi-supervised learning,consistency constraint,entropy regularization,time-frequency transform
AI 理解论文
溯源树
样例
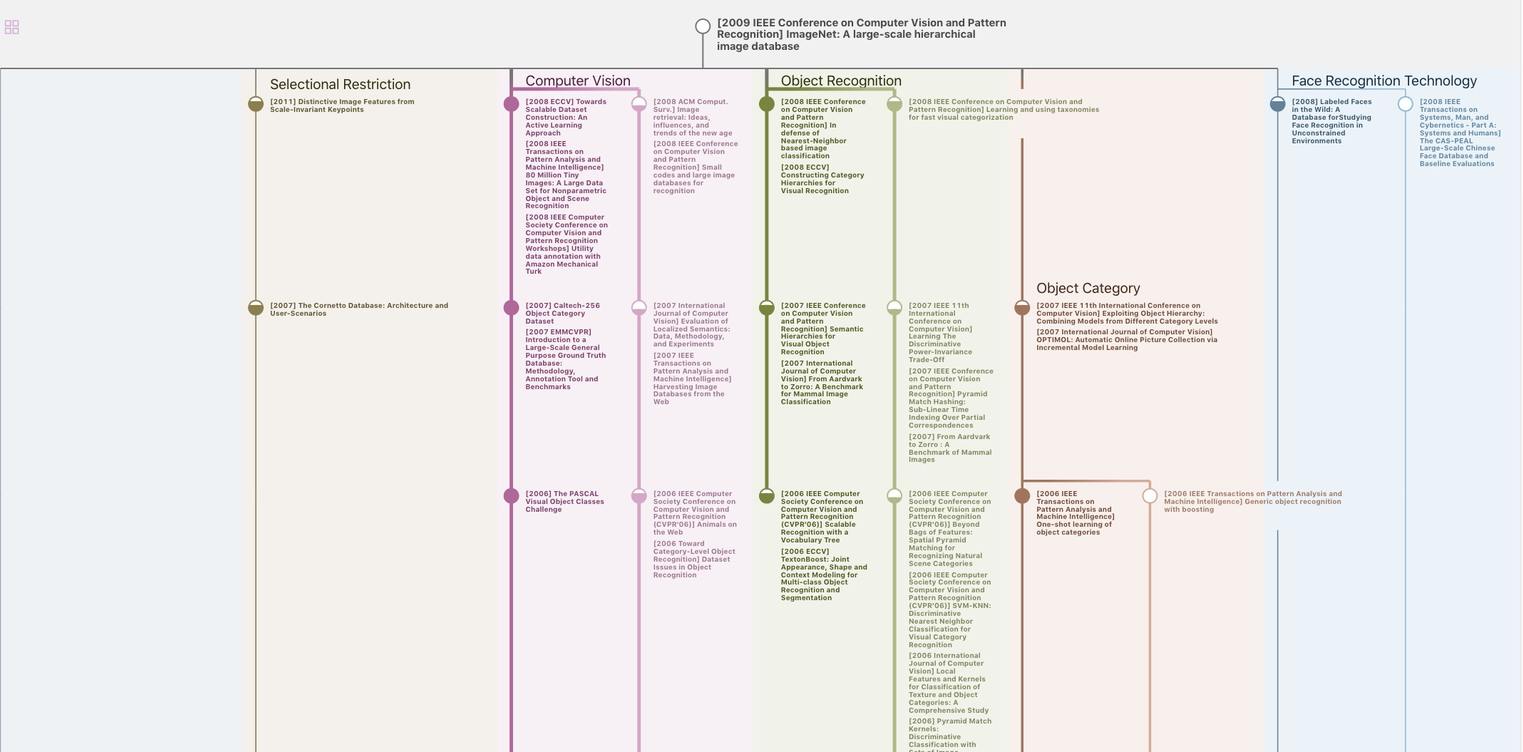
生成溯源树,研究论文发展脉络
Chat Paper
正在生成论文摘要