Images Retrieval and Classification for Acute Myeloid Leukemia Blood Cell Using Deep Metric Learning.
Asian Conference on Intelligent Information and Database Systems(2023)
摘要
Deep metric learning-based image retrieval systems have recently been used in medical applications because they provide clinically relevant information-based similar images based on prior knowledge. Although train examiners and deep learning models successfully analyze leukocyte cells, there are still numerous difficult challenges due to biological variation, time constraints, and a variety of image-related aspects. In this study, we propose a deep metric learning model-based image retrieval and classification system for acute myeloid leukemia blood cells to address these issues and assist physicians. The proposed model utilizes the pre-trained ResNet-34 model as the backbone network, embedding loss with multi similarity miner, and M-Per-Class sampling strategy to learn an embedding function. The five embedding losses were also applied to compare the four performances in order to determine the best loss-based model. Based on the best loss-based model, the class-wise precision and sensitivity using a neighborhood size are also presented. The results show that the contrastive loss-based deep metric learning model achieved the highest precision of 94.90%, sensitivity of 94.85%, specificity of 99.64%, and accuracy of 99.32% in model comparison. Except for a few failures in small classes, the class-wise precision and sensitivity scores looked to be impressive in all classes. Therefore, this proposed system can highly be effective in screening and diagnosing of AML-related white blood cell stages that cause serious cancer.
更多查看译文
AI 理解论文
溯源树
样例
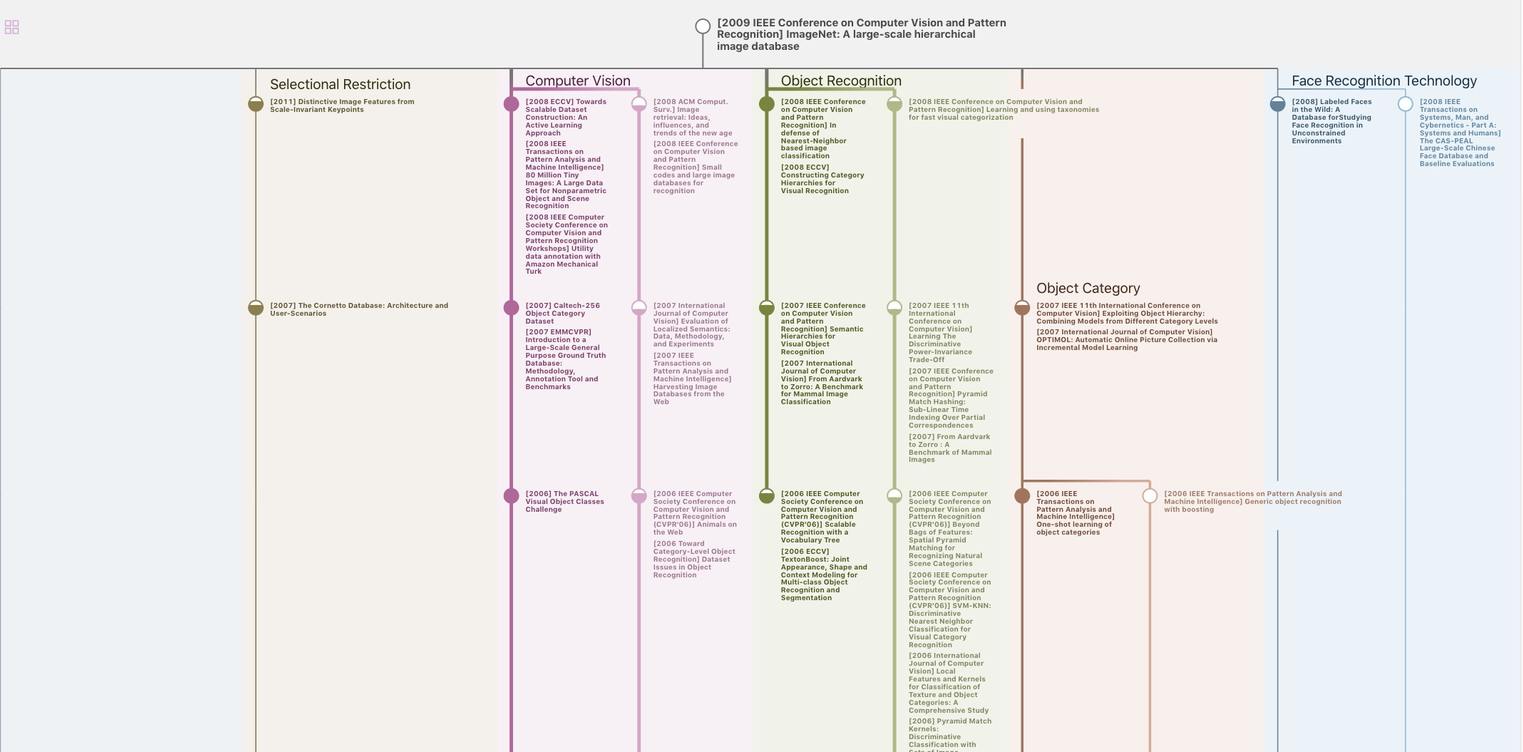
生成溯源树,研究论文发展脉络
Chat Paper
正在生成论文摘要