pNeurFill: Enhanced Neural Network Model-Based Dummy Filling Synthesis With Perimeter Adjustment
IEEE TRANSACTIONS ON COMPUTER-AIDED DESIGN OF INTEGRATED CIRCUITS AND SYSTEMS(2024)
摘要
Dummy filling is widely applied to significantly improve the planarity of topographic patterns for the chemical mechanical polishing (CMP) process in VLSI manufacturing. In the dummy filling flow, dummy synthesis works as the key step to adjust the post- CMP profile height. However, existing dummy synthesis optimization approaches usually fail to balance the filling quality and efficiency. This article proposes a novel model-based dummy filling synthesis framework NeurFill, integrated with multiple starting points-sequential quadratic programming (MSP-SQP) optimization solver. Inside this framework, a full-chip CMP simulator is first migrated to the neural network, achieving $8134\times $ speedup on gradient calculation by backward propagation. Entrenched in the CMP neural network models, we further implement an improved version of NeurFill (pNeurFill) to alleviate the post- CMP height variation caused by dummy perimeter. After each iteration of dummy density optimization, an additional perimeter adjustment based on a given candidate dummy pattern set is applied to search for the optimal perimeter fill amount. The experimental results show that the proposed NeurFill outperforms existing rule- and model-based methods. The extra perimeter adjustment strategy in pNeurFill can achieve an average 66.97 angstrom decreasing in height variation and 8.92% quality improvement compared to NeurFill. This will provide guidance for DFM so as to increase IC chip yield.
更多查看译文
关键词
Chemical mechanical polishing (CMP),design for manufacturability,metal fill synthesis,neural network,sequential quadratic programming (SQP)
AI 理解论文
溯源树
样例
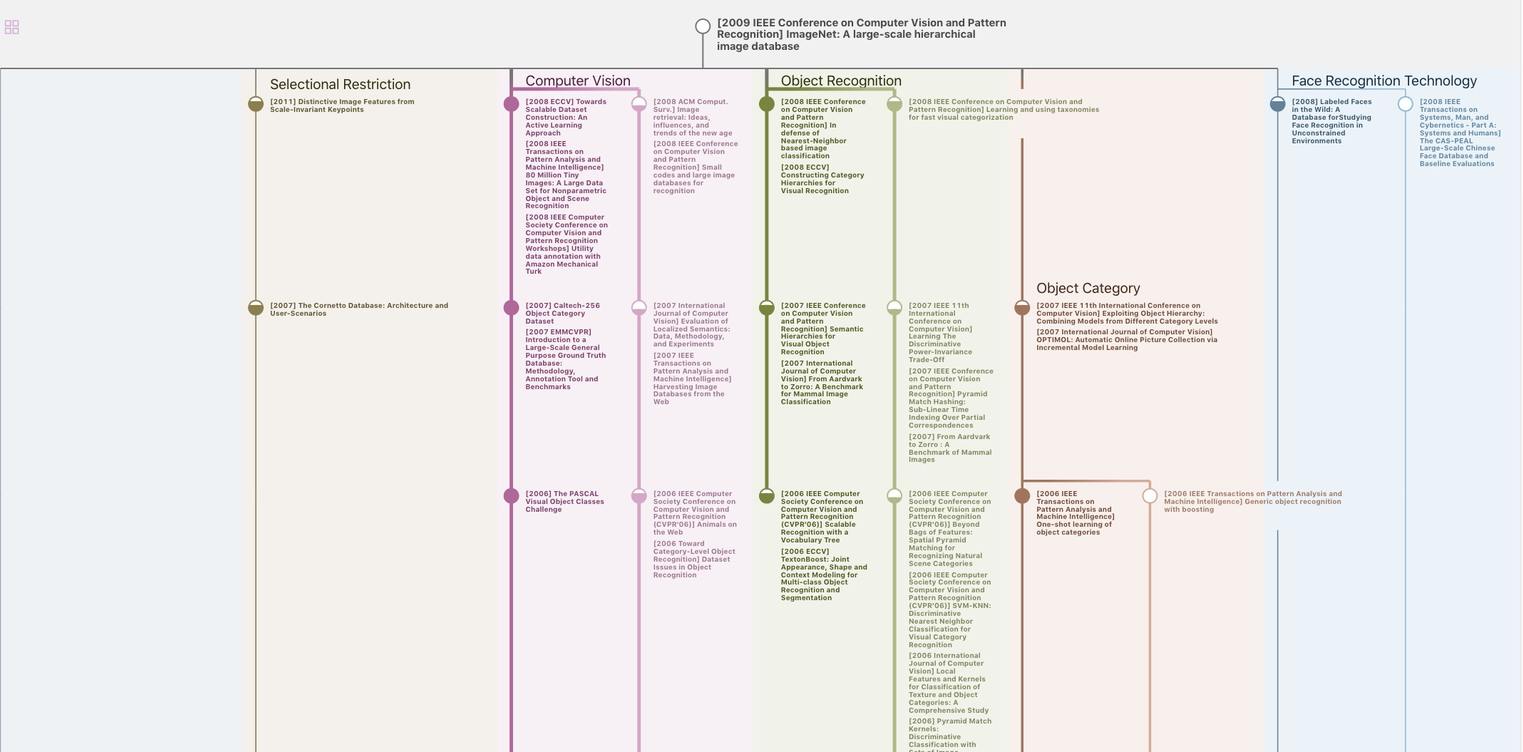
生成溯源树,研究论文发展脉络
Chat Paper
正在生成论文摘要