GPU Accelerated Full Homomorphic Encryption Cryptosystem, Library, and Applications for IoT Systems.
IEEE Internet Things J.(2024)
摘要
Deep learning such as convolutional neural networks (CNNs) have been utilized in a number of cloud-based Internet of Things (IoT) applications. Security and privacy are two key considerations in any commercial deployments. Fully homomorphic encryption (FHE) is a popular privacy protection approach, and there have been attempts to integrate FHE with CNNs. However, a simple integration may lead to inefficiency in single-user services and fail to support many of the requirements in real-time applications. In this paper, we propose a novel confused modulo projection based FHE algorithm (CMP-FHE) that is designed to support floating-point operations. Then we developed a parallelized runtime library based on CMP-FHE and compared it with the widely employed FHE library. Our results show that our library achieves a faster speeds. Furthermore, we compared it with the state-of-the-art confused modulo projection based library and the results demonstrated a speed improvement of 841.67 to 3056.25 times faster. Additionally, we construct a Real-Time Homomorphic Convolutional Neural Network (RT-HCNN) under the ciphertext-based framework using CMP-FHE, as well as using graphics processing units (GPUs) to facilitate acceleration. To demonstrate utility, we evaluate the proposed approach on the MNIST dataset. Findings demonstrate that our proposed approach achieves a high accuracy rate of 99.13%. Using GPUs acceleration for ciphertext prediction results in us achieving a single prediction time of 79.5 ms. This represents the first homomorphic CNN capable of supporting real-time application and is approximately 58 times faster than Microsoft’s Lola scheme.
更多查看译文
关键词
Convolutional neural networks (CNNs),Fully homomorphic encryption (FHE),Internet of Things (IoT),Graphics processing units (GPUs)
AI 理解论文
溯源树
样例
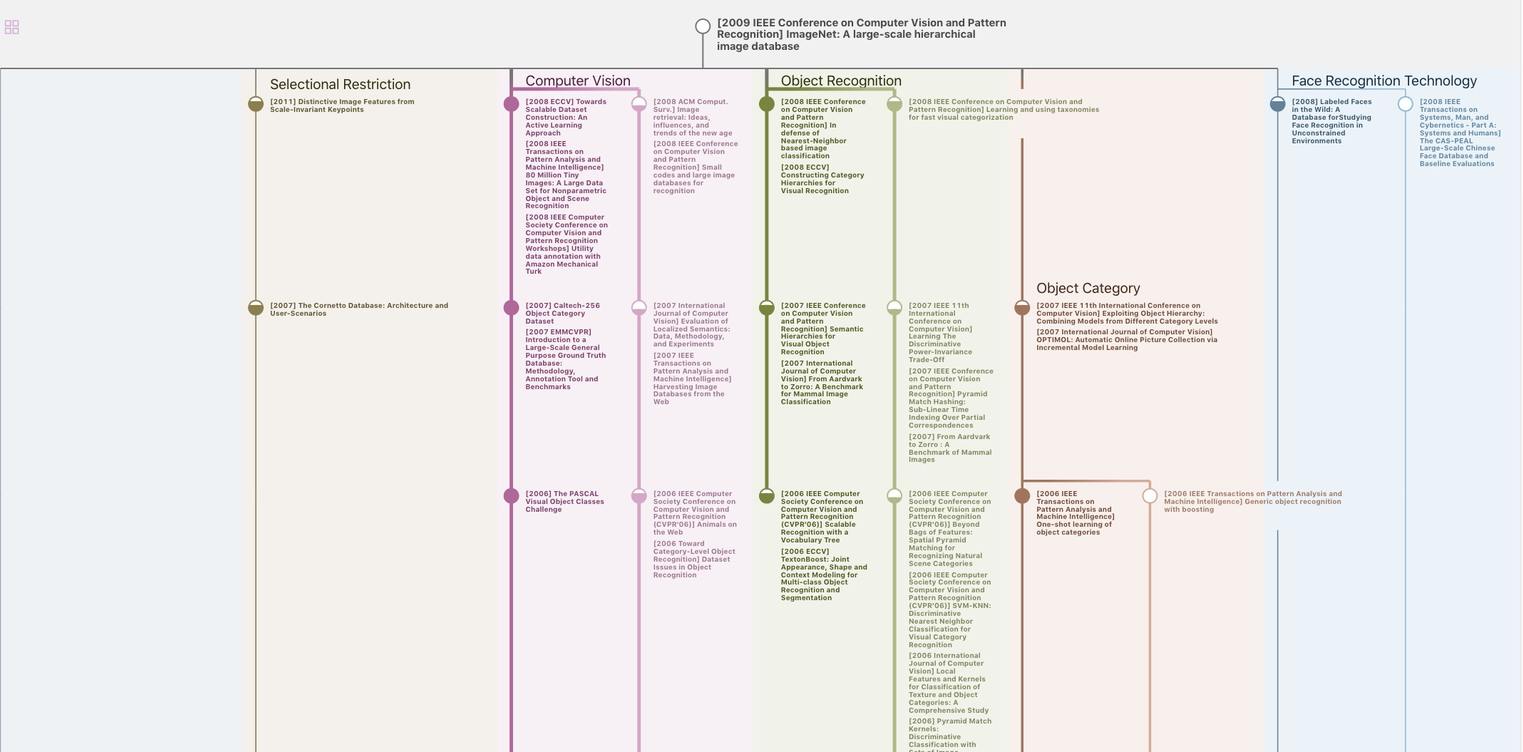
生成溯源树,研究论文发展脉络
Chat Paper
正在生成论文摘要