Experimental exploration of influential factors of concrete flexural strength through features engineering techniques: Insight from machine learning prediction
Research Square (Research Square)(2023)
摘要
Abstract The kind and quality of coarse aggregate have a direct impact on flexural strength (FS). As a result, this study used four different types of coarse aggregates, including those that depends on morphology, which contain coarse aggregates that can reach an extreme size of 20 mm and have the appearance of being flaky, angular, rounded, and irregular. The concrete mixtures were made based on Department of Environment (DoE) method of mix design, and a target FS of 5 MPa at 28 days was established. The FS of the concrete mixtures was assessed 7, 14, and 28 days after curing. In addition, the research employed Feedforward neural network (FFNN), Gaussian process regression (GPR), Multilinear Regression (MLR), and Stepwise Linear Regression (SWR) models in the prediction of concrete FS. The FFNN, GPR, MLR, and SWR models were trained on the investigational data obtained from the study's laboratory. The outcome showed that concrete with coarse aggregate in a round form had the maximum slump. Furthermore, the FS of the concrete produced with flaky and irregular coarse aggregates was the same as the FS of the concrete produced with angular and irregular coarse particles. The outcome also shows that the AI-based model (FFNN, GPR, MLR, and SWR) used in the study performs exceptionally well, with an R 2 -value higher than 0.90 during both the models’ training and testing phases respectively. The results also showed that SWR-M3 outperformed all other models with R 2 -value = 0.999 throughout the model's testing phase. This depicts that the model is highly accurate and successful in forecasting the concrete FS with various forms of coarse material.
更多查看译文
关键词
concrete flexural strength,features engineering techniques,machine learning prediction
AI 理解论文
溯源树
样例
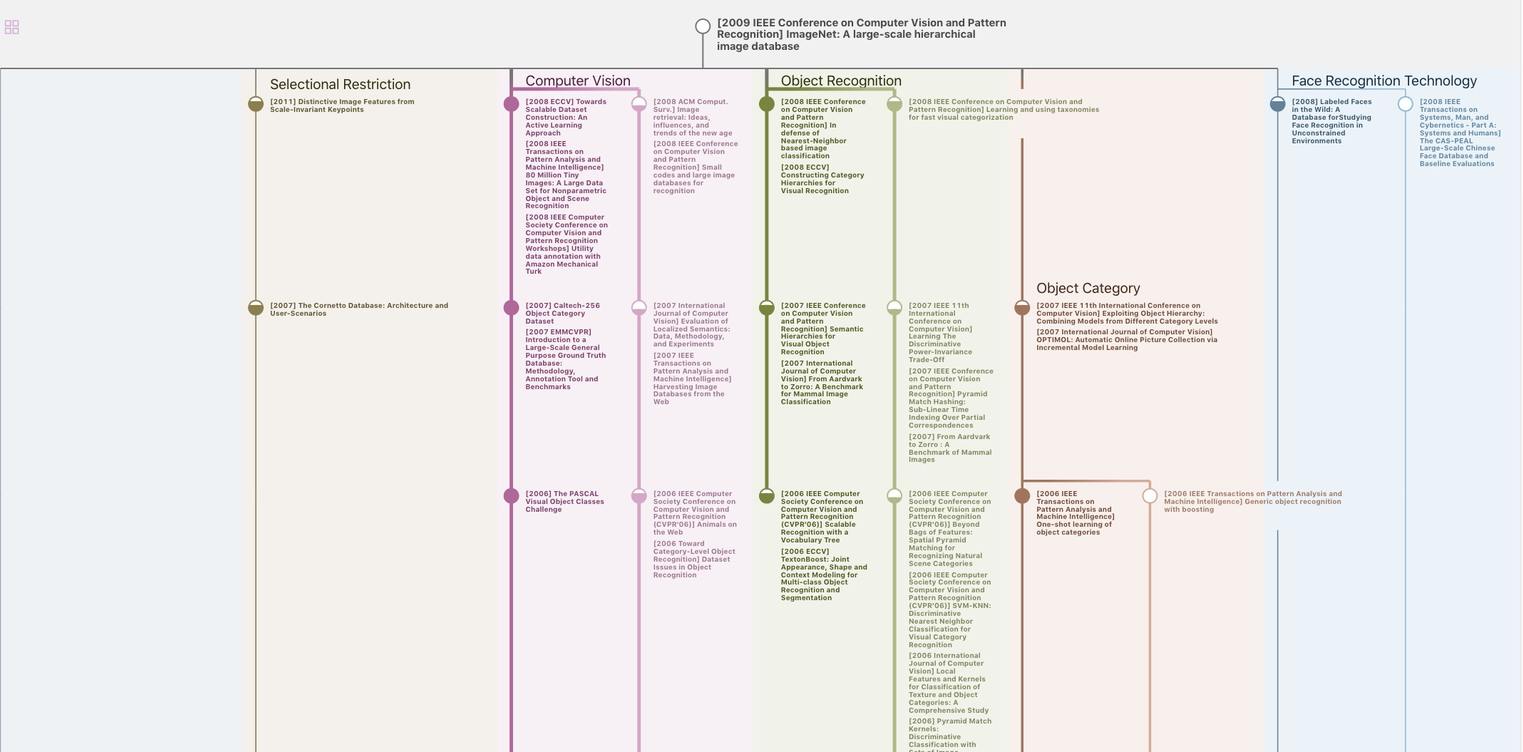
生成溯源树,研究论文发展脉络
Chat Paper
正在生成论文摘要