Multiclass Damage Detection for Autonomous Post-disaster Reconnaissance Using Quantum Convolutional Neural Network
Lecture notes in civil engineering(2023)
Abstract
Timely assessment of earthquake-induced building damage is critical for ensuring life safety, mitigating financial losses, expediting the rehabilitation process, and improving structural resilience. Due to the exponential growth in computer power and intrinsic capacity to address problems with manual inspections, the use of artificial intelligence (AI) in post-earthquake inspections and reconnaissance has drawn a lot of attention in recent years. With recent advancements in non-contact sensing technologies such as cameras, unmanned aerial and ground vehicles, the structural health monitoring (SHM) community has seen a significant increase in deep learning-based condition assessment methodologies of structural system. These deep learning algorithms mostly rely on convolutional neural networks (CNNs), which has risen its popularity for many machines learning (ML) applications, particularly in the field of image recognition. However, machine learning algorithms experience computational bottlenecks due to the curse of dimensionality. This study presents the adoption of Quantum Convolutional Neural Network (QCNN) to classify the building damages into five damage grades (1) Negligible to slight damage (2) Moderate damage (3) Heavy damage (4) Very heavy damage and (5) Collapse. The reinforced concrete building damage images collected from past-earthquake events are used to train the models and their performance is evaluated based on the testing images, neither of which are included to the training dataset. Furthermore, the comparison is made between the results obtained from QCNN and various CNNs architectures.
MoreTranslated text
Key words
quantum convolutional neural network,convolutional neural network,damage,post-disaster
AI Read Science
Must-Reading Tree
Example
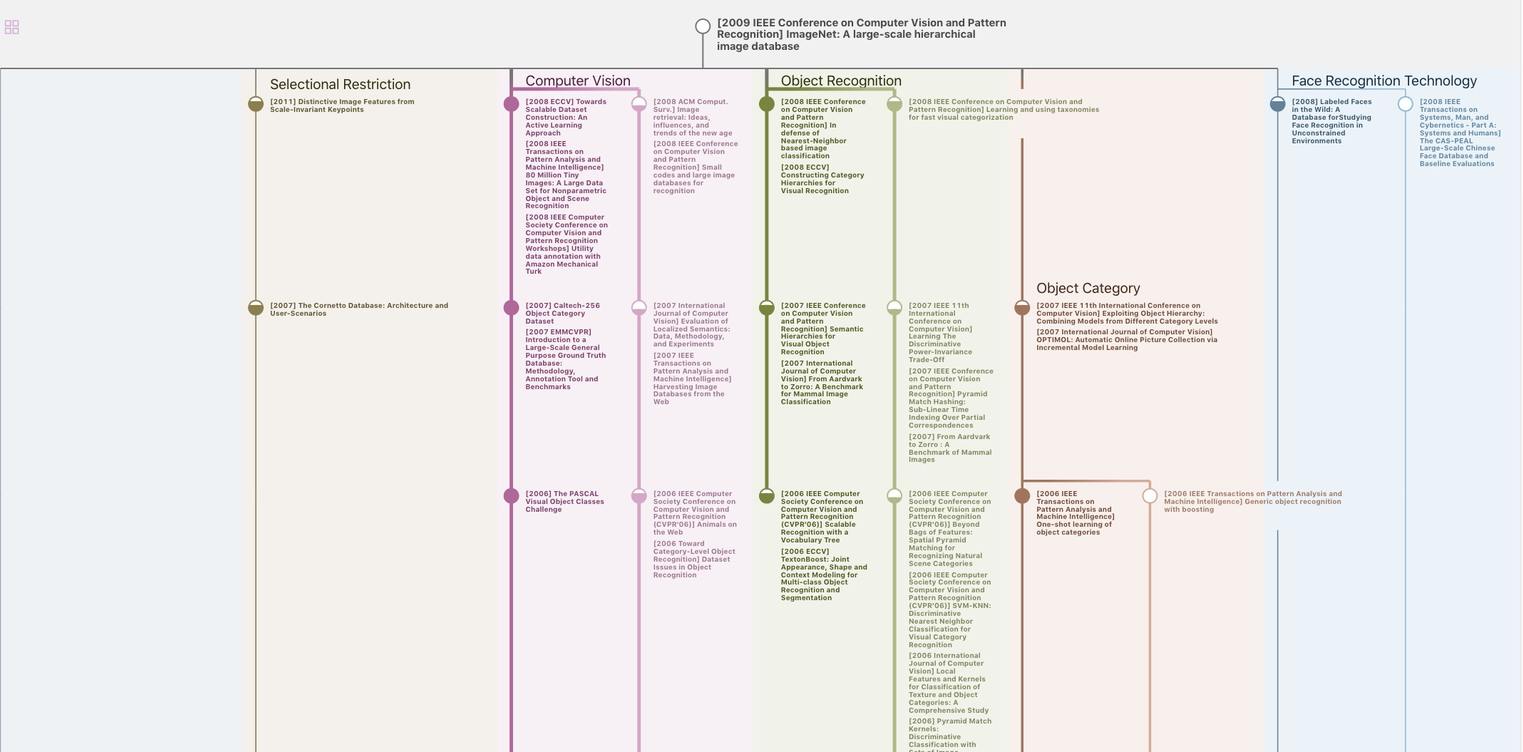
Generate MRT to find the research sequence of this paper
Chat Paper
Summary is being generated by the instructions you defined