HSMH: A Hierarchical Sequence Multi-Hop Reasoning Model With Reinforcement Learning.
IEEE Trans. Knowl. Data Eng.(2024)
摘要
The incompleteness of knowledge graphs (KGs) negatively impacts the performance of KGs in downstream applications (e.g., recommendation systems and information retrieval). This phenomenon has brought an increasing rise in research related to knowledge graph reasoning. Recently, emerged reinforcement learning-based multi-hop reasoning methods can infer missing information through multi-hop reasoning according to the existing information in KGs, which has better reasoning performance and interpretability. However, these methods always use relation-entity pairs that have been pre-cropped as the action space of agents for path reasoning, which leads to two problems: 1) insufficient learning and reasoning ability of reasoning models and 2) the hard convergence of the training process of agents. To address these problems, we propose a H ierarchical S equence M ulti H op (HSMH) reasoning framework, which consists of the interactive search reasoning model, local-global knowledge fusion mechanism, and action optimization mechanism. We use interactive search reasoning models to select relations and entities independently, thus fully mining the semantic information of relations and entities and improving the learning and reasoning ability of reasoning models. In the HSMH framework, we design the local-global knowledge fusion mechanism to acquire the local knowledge of entities and neighboring relations and the global knowledge about KG structure, which can improve the learning ability of reasoning models. Meanwhile, we design the action optimization mechanism to combine the filtered action space and the additional action space for efficient path reasoning of agents. Experimental results on benchmark datasets show that our proposed HSMH framework comprehensively outperforms the state-of-the-art multi-hop reasoning model.
更多查看译文
关键词
Action optimization,knowledge graphs,local and global knowledge fusion,multi-hop reasoning,reinforcement learning
AI 理解论文
溯源树
样例
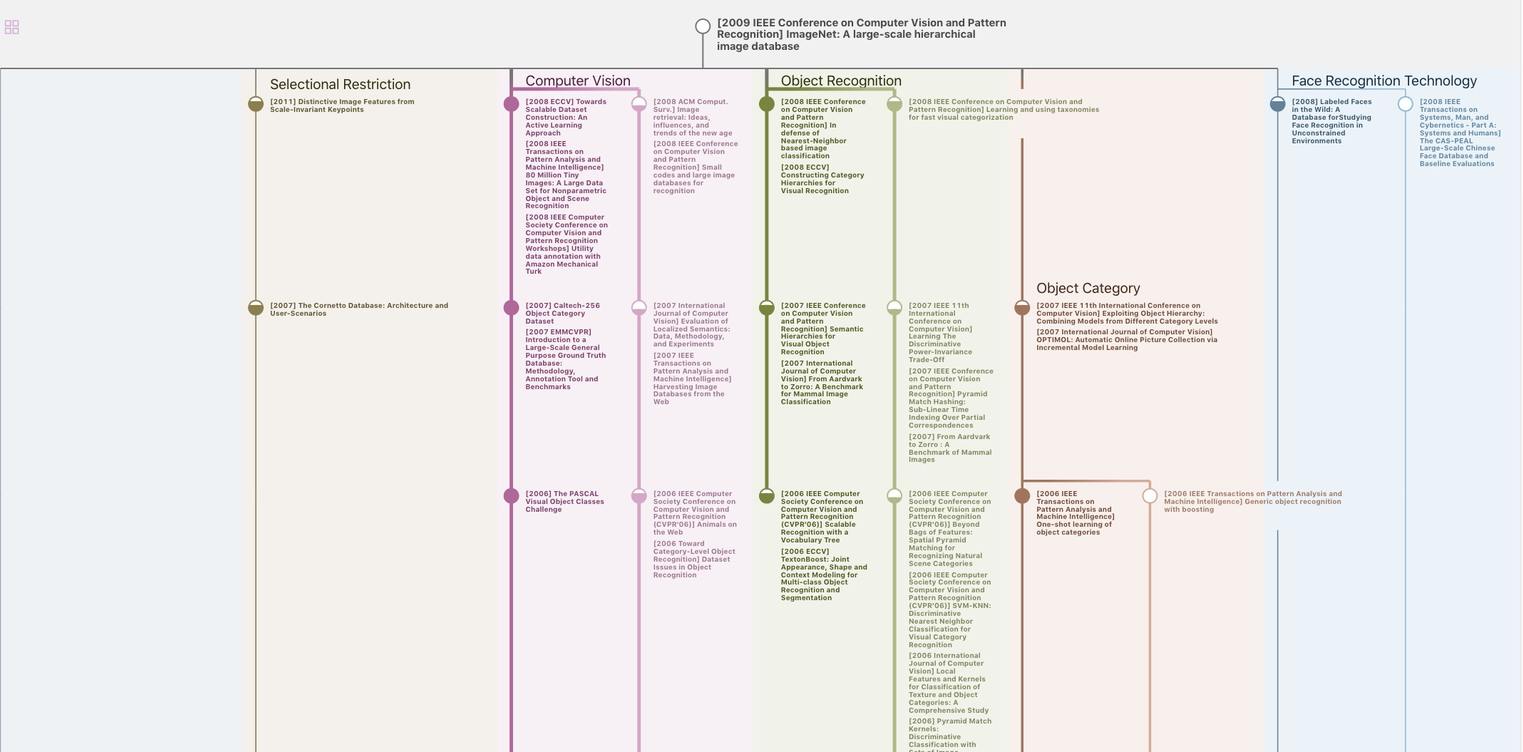
生成溯源树,研究论文发展脉络
Chat Paper
正在生成论文摘要