Word Prediction Model using an elaborated RNN integrated with N-gram for efficient Text Input
Research Square (Research Square)(2023)
摘要
Abstract Smartphone users are beyond two billion worldwide. Heavy users of the texting application rely on input prediction to reduce typing effort. In languages based on the Roman alphabet, many techniques are available. However, Japanese text is based on multiple character sets such as Kanji, Hiragana and Katakana. For its time intensive input, next word prediction an open challenge. To tackle this, a hybrid language model is proposed. It integrates a Recurrent Neural Network (RNN) with an n-gram model. RNNs are powerful models for learning long sequences for next word prediction. N-gram models are best at current word completion. Our RNN language model predicts next words. According the “price” of the performance gain paid by a higher time complexity, our model best deploys on a client-server architecture. Heavily-loaded RNN-LM deploys on the server while the n-gram model on the client. It consists of an input layer equipped with word embedding, an output layer, and hidden layers connected with LSTMs (Long Short-Term Memories). Training is done via BPTT (Back Propagation Through Time). For robust training, BPTT is elaborated by learning rate refinement and gradient norm scaling. To avoid overfitting, the dropout technique is applied except for LSTM. Due to synergetic elaboration, it shows 10% lower perplexity than Zaremba’s excellent conventional models in our experiment. Our model has been incorporated into IME (Input Method Editor) we call Flick. In our experiment, Flick outperforms Mozc (Google Japanese Input) by 16% in time and 34% in the number of key strokes.
更多查看译文
关键词
word prediction model,rnn,input,n-gram
AI 理解论文
溯源树
样例
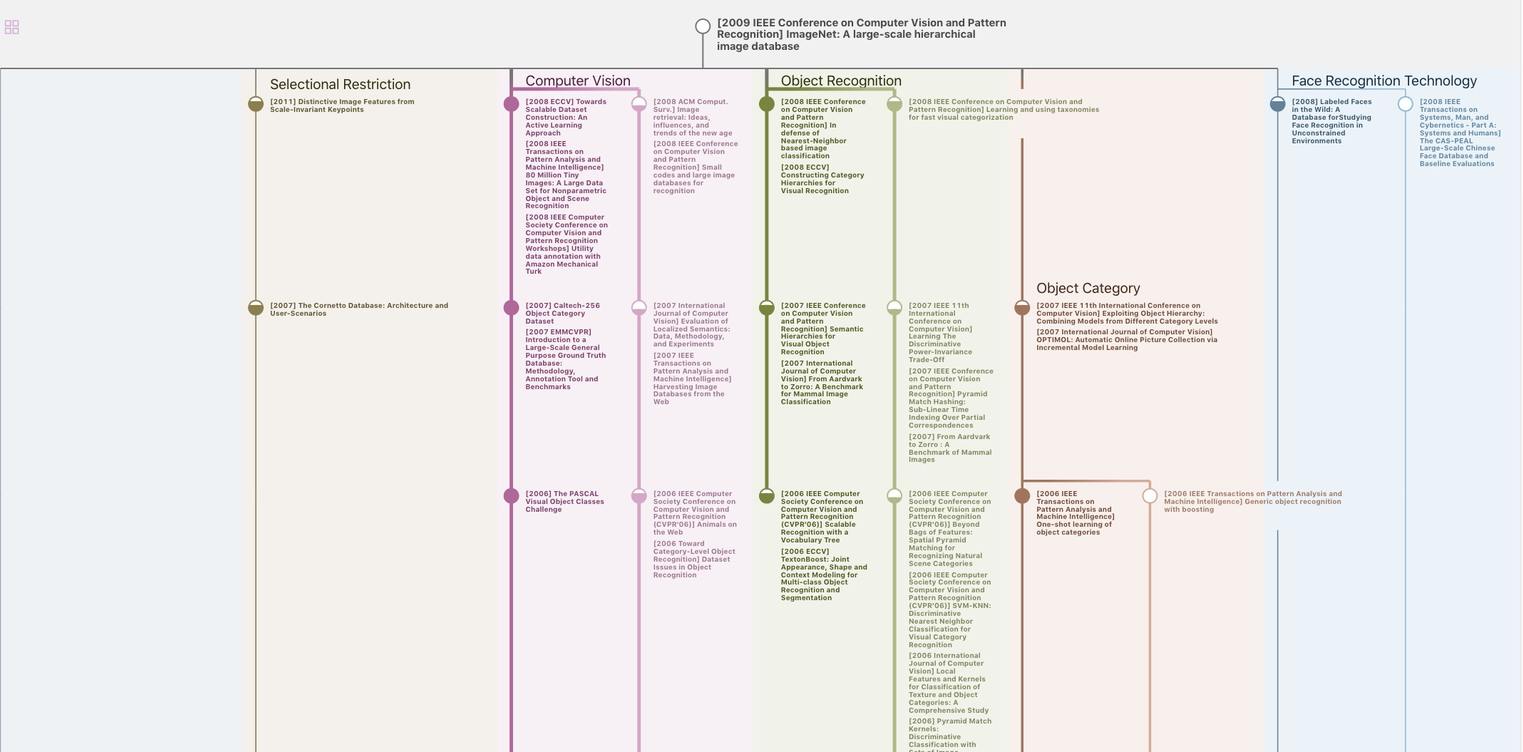
生成溯源树,研究论文发展脉络
Chat Paper
正在生成论文摘要