Data-driven signal analysis of sensory cortical processing using high-resolution fMRI across different studies
bioRxiv (Cold Spring Harbor Laboratory)(2023)
Abstract
Abstract The analysis of large data sets within and across preclinical studies has always posed a particular challenge in terms of data volume and method heterogeneity between studies. Recent developments in machine learning (ML) and artificial intelligence (AI) allow to address these challenges in complex macro- and microscopic data sets. Because of their complex data structure, functional magnetic resonance imaging (fMRI) measurements are perfectly suited to develop such ML/AI frameworks for data-driven analyses. These approaches have the potential to reveal patterns, including temporal kinetics, in blood-oxygen-level-dependent (BOLD) time series with a reduced workload. However, the typically poor signal-to-noise ratio (SNR) and low temporal resolution of fMRI time series have so far hampered such advances. Therefore, we used line scanning fMRI measurements with high SNR and high spatio-temporal resolution obtained from three independent studies and two imaging centers with heterogeneous study protocols. Unbiased time series clustering techniques were applied for the analysis of somatosensory information processing during electrical paw and optogenetic stimulation. Depending on the similarity formulation, our workflow revealed multiple patterns in BOLD time series. It produced consistent outcomes across different studies and study protocols, demonstrating the generalizability of the data-driven method for cross-study analyzes. Further, we introduce a statistical analysis that is entirely based on cluster distribution. Using this method, we can reproduce previous findings including differences in temporal BOLD characteristics between two stimulation modalities. Our data-driven approach proves high sensitivity, robustness, reproducibility, and generalizability and further quickly provides highly detailed insight into characteristics of BOLD time series. Therefore, it holds great potential for further applications in fMRI data including whole-brain task and resting-state fMRI, which can support fMRI routines. Furthermore, the analytic framework can be used for datasets that have a time-dependent data structure to integrate study results and create robust and generalizable datasets, despite different study protocols.
MoreTranslated text
Key words
sensory cortical processing,signal analysis,data-driven,high-resolution
AI Read Science
Must-Reading Tree
Example
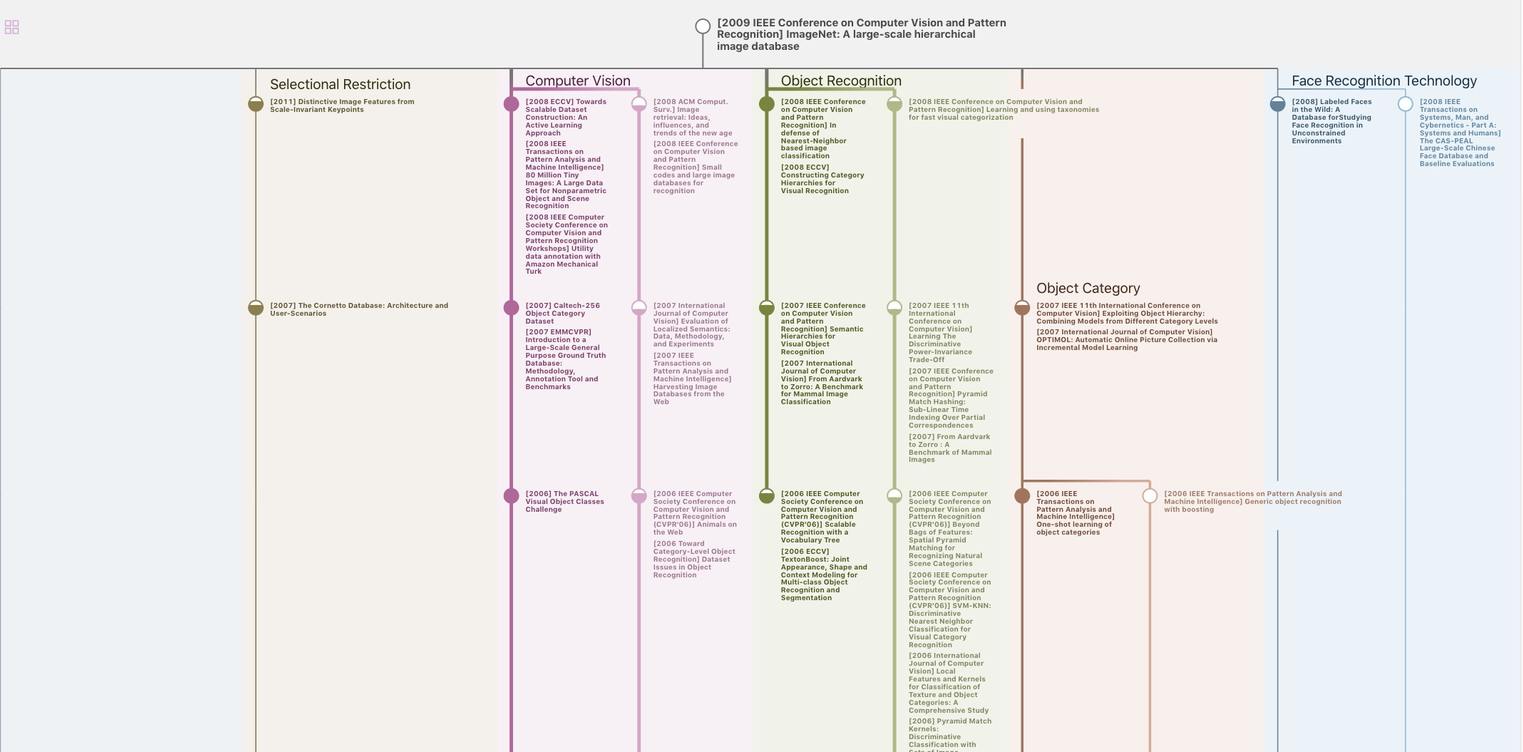
Generate MRT to find the research sequence of this paper
Chat Paper
Summary is being generated by the instructions you defined