Comparison of a Long Short-Term Memory Model with Statistical-Based Water Demand Prediction Models on a Case Study of Spain
Lecture notes in civil engineering(2023)
摘要
Accurate prediction of future water demand is desirable in both design and operation of water distribution networks (WDNs). While the long-term forecasting is helpful in planning and designing the system, a short- to medium-term forecasting contributes in better operation, maintenance practices and calibration of the system. The automations in WDNs have emphasised on more accurate prediction of daily and weekly demands for online scheduling of pump operations and valve adjustments. In this paper, a deep learning algorithm called recurrent neural network (RNN) has been used to train the model and forecast hourly water demand, using long short-term memory (LSTM) layer for a city of Spain. The performance of LSTM model is compared with some statistical hybrid models such as ensemble empirical mode decomposition (EEMD), difference pattern sequence forecasting (DPSF) (EEMD-DPSF), and the EEMD with DPSF and autoregressive integrated moving average (ARIMA) (EEMD-DPSF-ARIMA), etc. by means of root mean squared error (RMSE) and mean absolute error (MAE) and mean percentage absolute error (MAPE). Results of this study show that the LSTM-based model can make predictions with improved accuracy than the other models that are being compared when dealing with data with higher time resolutions, data points with abrupt changes, and data with a relatively high uncertainty level. It is also observed that with respect to EEMD-DPSF, LSTM-based models provide better performance in predicting multiple successive water demands.
更多查看译文
关键词
water demand prediction models,prediction models,short-term,statistical-based
AI 理解论文
溯源树
样例
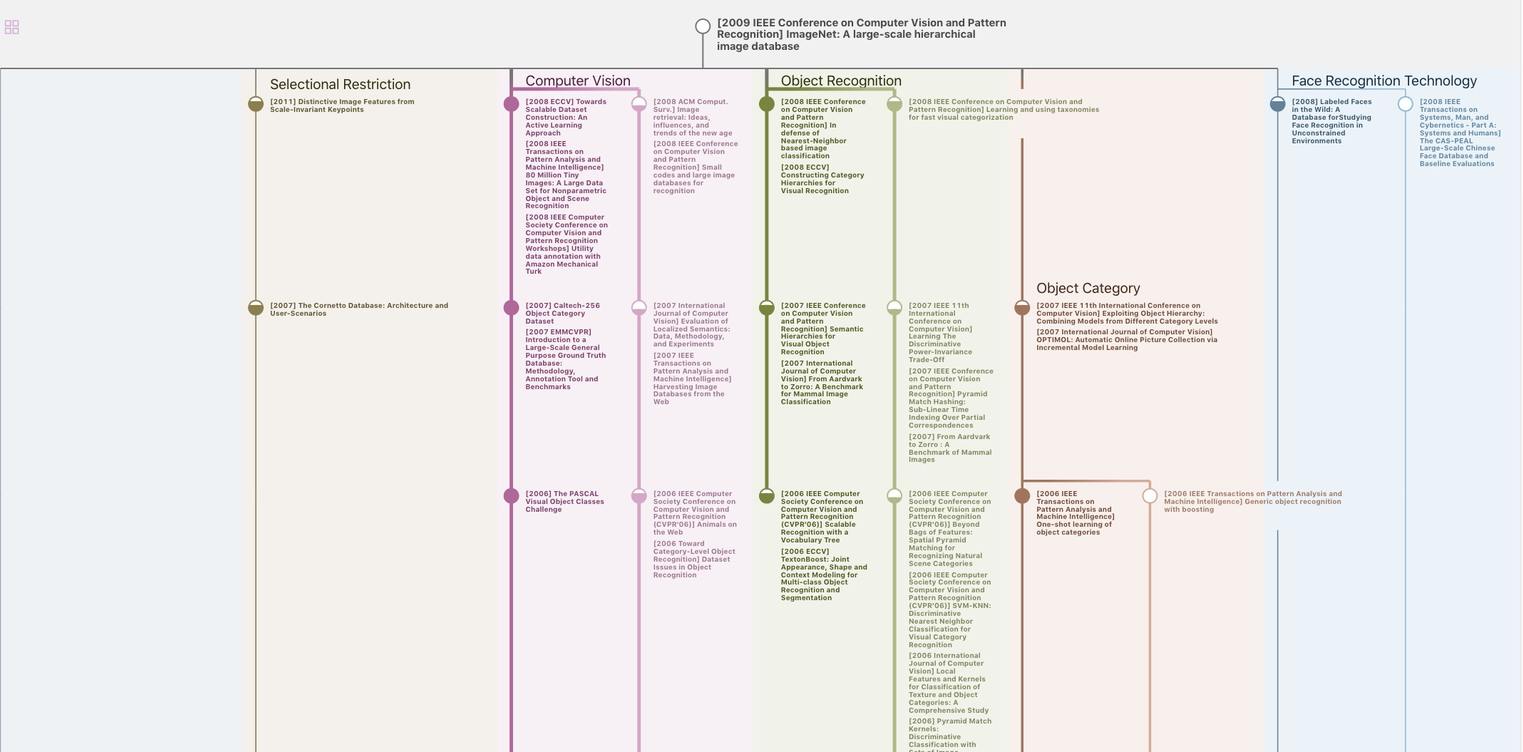
生成溯源树,研究论文发展脉络
Chat Paper
正在生成论文摘要